By Dean Jackson and Meghan Conroy
“There was nobody at the plane,” wrote a frustrated Donald Trump, the forty-fifth president of the United States and Republican nominee for president in 2024. “She ‘A.I.’d’ it, and showed a massive ‘crowd’ of so-called followers, BUT THEY DIDN’T EXIST!”
It was August 2024, and Trump was accusing his Democratic Party opponent, Vice President Kamala Harris, of faking photographs of crowds at her rallies using generative artificial intelligence (GAI, or generative AI). Unlike photo-editing techniques used by political operatives in decades past, GAI tools allow relative novices to create convincing forgeries in moments with a few keystrokes.
Harris had not faked the photos; the crowds were real. Thousands of people saw them. Journalists took photos of their own. A viral claim that the photos must have been faked because the crowd’s reflection could not be seen on the side of Harris’s plane turned out to have a simple explanation: the plane was angled away from the crowd.
For months, the public had been warned to expect a GAI-fueled surge of deception to disrupt elections around the world. And yet, ironically, here was an instance of a real image being called a fake. Some observers had also pointed to the potential for widespread fears about synthetic content to create a “liar’s dividend,” allowing public figures accused of bad behavior to cast doubt on evidence of their wrongdoing by claiming it was AI-generated. But in the case of the crowds at Harris’s rallies, Trump was doing something subtly different. Instead of avoiding accountability, he was denying evidence of his opponent’s popularity.
There had been other high-profile incidents in which GAI really was used to deceive: synthetic audio of a Chicago mayoral candidate endorsing police violence, a Slovak parliamentary candidate discussing election fraud (and threatening to raise the price of beer), and, most famously, US President Joe Biden calling New Hampshire voters and urging them to sit out the Democratic primary in their state. Fake images circulated too: of Trump embracing former health official Anthony Fauci (a hugely controversial figure on the right for his role in pandemic response), Trump posing with Black supporters, Trump being placed under arrest, and a lawless United States under a hypothetical second Biden administration. In a year in which more than half of humanity lives in countries holding an election, dozens more examples have emerged month after month. “AI deepfakes threaten to upend global elections. No one can stop them,” read one Washington Post headline in April.
Threat actors have also continued to experiment. In September—just two months before Election Day in the United States—the US Department of Justice (DOJ) announced that it had disrupted a Russian influence operation known as “Doppelganger,” seizing thirty-two web domains used to spread pro-Russian propaganda and imitate authentic news sites, including the Washington Post. Some of these sites were promoted through social media advertisements created by GAI. Doppelganger was not the only operation that the DOJ disrupted in September. And while it was the one that made the clearest use of AI, there are almost certainly yet undiscovered operations—by Russia, or by other state adversaries such as Iran or the People’s Republic of China—that have used or will use AI for malign purposes.
But few deepfakes have landed with the impact observers dreaded, with most treated more like memes than credible evidence. By May, major outlets began managing expectations around AI deepfakes with headlines such as “A.I. Promised to Upend the 2024 Campaign. It Hasn’t Yet.” Concerns about AI-generated disinformation have moderated as the 2024 US elections approach. An August 9 report from the Microsoft Threat Analysis Center noted that while “nearly all actors seek to incorporate AI content in their operations . . . more recently many actors have pivoted back to techniques that have proven effective in the past—simple digital manipulations, mischaracterization of content, and use of trusted labels or logos atop false information.”
This is not to say the risk is not real. Down-ballot elections, less visible in the national media, may prove to be less resilient than the presidential contest. As with any early analysis of emerging technology, the assessment informed by our research is mid-stride. New examples of artificial intelligence in politics and elections continue to surface. Expert opinion is still nascent and could significantly shift.
A consensus among experts is emerging, though, that AI’s chief short-term impact on elections will not be to create wholly new problems but rather to make previous threats more common and easier to carry out by a wider array of actors, leading to a higher volume of disinformation, cyberattacks, and other issues. In the long-term, AI seems poised to inject more paranoia and uncertainty into societies (such as the United States) that are already suffering from deep mistrust in government, in media, and among citizens.
In short, we are learning that the potential risks posed to elections from generative AI are more akin to a cancer than a heart attack.
In the headlines
Our expert gauge
Since late 2023, sudden and dramatic interest in artificial intelligence has led to boundless speculation in the United States about AI-borne threats to the 2024 elections. Artificial intelligence is not new to politics. Any ad placed by a politician on Facebook, TikTok, or YouTube is delivered partially by AI systems. Generative AI, however, is relatively new to the political scene, with recent advances making it more potent and accessible. GAI’s ability to interpret human-generated text and draw on vast amounts of data to create novel media in many formats opens new possibilities for influencing politics and elections while supercharging older ones.
In response, the Atlantic Council’s Digital Forensic Research Lab (DFRLab) sought to understand these risks and how experts thought about them. After nine interviews with individuals working in philanthropy, technology, academia, advocacy groups, and political campaigns, the DFRLab conducted a wider survey in which respondents were asked to rate hypothetical risk scenarios according to their likelihood and potential impact (two facts that, taken together, constitute a reasonable definition of “risk”). On January 31, 2024, the DFRLab hosted an expert forum during which many of the survey participants discussed these risk scenarios in dedicated focus groups. Participants in these focus groups are quoted throughout the analysis below, though their names are not included because the sessions were conducted under the Chatham House rule.
Despite widespread concerns over political deepfakes, many interview and survey participants were skeptical when asked about scenarios involving a high-profile deepfake. Some noted that the quality of a fake is not always determinative of its spread or influence. In early 2020, for example, a crudely manipulated video (or “cheapfake”) of Nancy Pelosi made the former speaker of the US House of Representatives appear inebriated during a speech. The video spread quickly across social media without the benefit of GAI, perhaps because deceptive content is often less valuable as a tool for persuasion than as a way of encouraging audiences to reinforce and repeat their preexisting beliefs and biases.
Other participants noted that many swing voters in the United States still rely on traditional media gatekeepers for news and information, and that national media would be swift to debunk synthetic video of high-profile figures such as presidential candidates. But at the local level, AI-enabled deception might be more dangerous. Local media in the United States is in dire economic shape. The number of working journalists has been declining since the 1980s, and more newspapers shut down each year. This leaves fewer reliable sources of information to debunk viral falsehoods.
Female candidates for political office are also at high risk, as several cases from around the world indicate. Cara Hunter, a member of the Northern Ireland Legislative Assembly, was the victim of AI-generated sexual imagery during her campaign in 2022. In Bangladesh’s 2024 election, female candidates were also targeted with synthetic sexualized images. (More than 90 percent of deepfake videos are believed to be pornographic, with nearly all of them depicting women.)
Another risk is that social media platforms, in an effort to prevent the spread of political deepfakes, will inadvertently remove a genuine recording, leading to a controversy over content moderation like that which occurred during the Hunter Biden laptop affair. The poor quality of many automated detection tools contributes to this risk; participants in our research activities described some tools as being “less accurate than a coin flip.”
Despite widely reported concerns about synthetic video and images, synthetic audio might ultimately be more threatening. The less flashy use of fake audio is relatively harder to detect and even easier to create; it can be done for pennies using only a few minutes of real recordings. If what one participant called a “big deepfake” does derail an election, it could well be in an audio format rather than a visual one.
AI astroturfing and the pink-slime tsunami
GAI is not limited to images or audio. The tool that captured imaginations in November 2022, ChatGPT, allows users to access a large language model (LLM) to generate text. As a medium, text might seem too old-school to matter in the age of synthetic video, but computer code is ultimately text-based. An interactive video chatbot would generate its dialogue as text first, and then use a different application to render that text into speech and real-time video movements. Text is the basis for nearly everything, and the ability to generate convincingly human prose fluently in real time is powerful.
While ChatGPT’s creator, OpenAI, has implemented safeguards such as prohibiting the impersonation of real political candidates using its services, it is only one of a growing number of providers. And it has not been able to stop a different type of threat: the creation of an unlimited stream of “pink slime” clickbait propaganda.
Organizations including Newsguard have documented the use of GAI to create dozens of clickbait pages. While these kinds of pages have existed for several years, GAI allows opportunists and bad actors to produce them in larger numbers, at a higher level of quality, much more quickly, and at trivial cost. Russia and other state actors already appear to be experimenting with GAI for exactly this purpose. For instance, one of the indictments that the Department of Justice brought against Russian operatives in September showed hallmarks of AI-generated content, such as chatbot prompts or other information accidentally included in website copy.
This capability could be combined with automated accounts to enhance existing methods of political “astroturfing,” in which operatives create fake grassroots movements. A 2023 RAND study called the prospects for a revolution in astroturfing the “most concerning” shift that GAI might introduce into social media manipulation. Automated bot accounts are cheap; networks of thousands are bought and sold on the internet for trivial sums. But bot accounts have also traditionally been limited in their functionality. Generative AI could change this equation by converting bot accounts into fully interactive, fully automated chatbots, finally recognizing the potential of previous experiments such as SmarterChild or Microsoft’s Tay. That could add exponentially more variety to spammed messages, making them more credible and more difficult to detect. Some speculate that this technology could be combined with text or private messaging applications, robocallers, and other technologies to bring these chatbots off social media and onto other platforms.
Techniques such as those above might increase the volume of disinformation through sheer scale of production, but distribution hurdles remain. For starters, the impact of political outreach tends to diminish as it becomes more widespread. Many of our interview participants referenced the explosion of political “text-banking,” which was widely considered successful in 2020 but has become so ubiquitous by 2024 that users have begun to treat political texts more like spam. Similarly, most robocall recipients hang up. As one interview participant said, “Unless AI can pretend to be your brother, the call doesn’t matter. That channel is clogged.”
A 2023 article in the Harvard Misinformation Review issued similar warnings. The authors argue that increased volume might not translate into increased impact if AI-generated content fails to achieve widespread distribution. Online content is engaged in a competition for attention, a resource that is far more limited than the vast oceans of incendiary and misleading content on the internet—even before generative AI’s emergence.
On the other hand, some of our expert participants flagged that in emergency situations a higher volume of misleading content could be consequential. Trustworthy news takes time to produce; bad actors have a first-mover advantage and can flood the zone with rumors, innuendo, and outright lies. Generative AI makes this easier and faster. As one interview participant said, “Imagine if we had the Black Lives Matter summer now.” The impact of GAI in a situation such as that could be significant. And it would only have to motivate a particular subset of the public—for example, armed militia members—to matter.
More pervasive does not mean more persuasive
Many experts fear that messages generated by LLMs will be more persuasive than human-created variants. This fear is rooted in a few predictions: that AI will facilitate even more narrowly targeted messaging campaigns (sometimes called hyper-targeting); that AI will allow political operatives, domestic partisans, and foreign propagandists to phrase messages in ways that are more credible to specific communities; or that GAI-generated text will be optimized to influence audiences in other ways, above and beyond human-generated messages.
Interviewees working in political and advocacy campaigns were excited about the possibilities afforded by GAI for better microtargeting of audience segments. GAI can provide an interface with which analysts can more quickly identify and assess patterns. Communicators can then use GAI to produce many variations of content narrowly targeting audience segments based on that analysis. This streamlines what has heretofore been a time- and labor-intensive process for campaigns. (Indeed, it does something similar for commercial advertisers, who for years have been using digital tools to produce multiple versions of ads targeting different consumer segments.)
But it is easy to overstate the persuasive power of micro-targeted political ads (or political ads in general), about which previous studies have reached mixed findings. Indeed, as one of our expert participants said, “If we’re talking about its ability to persuade or change votes in like the electoral process . . . it could just be that everyone who liked it was already predisposed to liking it.” Or as another participant said, “We worry too much about the persuasive power of a single message” in a saturated media and campaign environment.
New evidence is adding nuance to debates over the persuasive power of AI-written messages. Recent research, for example, suggests that headlines written by GAI actually perform slightly worse on average than human-written headlines, though this gap disappears if humans provide modest editorial oversight of GAI-produced headlines. This study was based on GPT-3, which debuted in June 2020, and more recent LLMs might change the equation. But it appears that, for now at least, many AI tools provide faster, more efficient production rather than a giant leap in persuasive power. This supports the conclusion that GAI’s main impact on political persuasion at present will be lowering obstacles to production, especially for resource-constrained actors, and increasing the speed of political communications professionals and propagandists alike.
These are not reasons to completely dismiss hyper-targeting and AI-enhanced persuasion as threats. As another interviewee said, the impact does not need to be dramatic to be significant. Reaching a few voters in the right place could be consequential, because “a stadium full of people will decide the outcome of the [US] election.” Small changes at the margins are impactful in campaign contexts, and a smart bad actor could use this technique surgically for outsize results.
Voter suppression in the AI era
Voter suppression is one such case. The effect of voter suppression differs based on which voters are targeted; suppression in noncompetitive states and districts would be less disruptive than an operation that targeted a crucial demographic in a swing state. Operations targeting minority languages may also be more difficult to detect and respond to than those in English.
One of the scenarios we surveyed experts about for this study focused on the delivery of voter-suppression messages via text or private-messaging applications, but voter-suppression content could also be circulated over social media, as it has been in the past. This content violates the civic-integrity policies of many major platforms, but the degradation of the trust and safety field over the last two years brings into serious question the ability of platforms to enforce these policies.
Survey respondents disagreed on how AI would play into such an operation. If bad actors want to create unique messages quickly and efficiently, AI can help them do that. But would AI-generated messages outperform previous forms of human-generated voter-suppression content? At least one survey respondent believed they would, writing that AI would be “instrumental” in making the content “one hundred times more believable.”
Others played down the perceived efficiency gains from using GAI in this way, saying, for example, that it was “not hard, pre-AI, to write a bunch of different short texts.” Another called the role of AI “minimal” because “an office of one hundred wage workers in Russia [or] China” could run this effort without AI.
Still others focused on distribution questions. For instance, one noted that operations might use AI to deploy interactive voter-suppression chatbots. But when most voters are already inundated with political spam across so many channels, even a tool such as that might not be enough to break through the noise.
Finally, while our scenario focused on text- or audio-based messages to voters, some experts worried about the combination of tactics to create synthetic images of violence at specific polling places to suppress turnout in a more targeted fashion. This risk only increases the need for election administrators, elected officials, civil society, and the news media to work together to inform the public about sources of reliable information during the election.
Cyberattacks: The elephant in the chatroom
When asked in January 2024 what about AI and the election most concerned them, one interviewee said emphatically that the expanded possibility of cyberattacks receives less attention than it deserves. And, indeed, in August 2024, CNN reported that the Federal Bureau of Investigation believes that Iranian hackers breached the Trump campaign as part of a hack-and-leak operation (though it is not clear that generative AI was used to facilitate that attack).
Our survey results and focus groups reflected a debate about how and whether AI would augment cyber risks in 2024. Some respondents suggested that, while AI could over several years give defenders greater advantage, the technology could make it much easier in the short term for amateurs to discover and take advantage of vulnerabilities. Initial research suggests that GAI models do not appear to provide substantial benefits when compared with existing tools in terms of the discovery or exploitation of vulnerabilities, and that there are still barriers to their ability to help novice hackers execute attacks end-to-end. There have, however, already been cases of GAI models assisting hackers with “impersonation attacks” in which they pretend to be a coworker or superior to obtain access to an information system, which could be a threat to election-system security.
When asked about a distributed denial-of-service (DDOS) attack on, for example, online voter-registration portals, in which GAI models would be used to create diverse fraudulent registrations, many were quick to point out that DDOS attacks are simple to carry out without AI and that there is a longstanding playbook for preventing and responding to them. Others pointed to safeguards and processes that election officials could use to weed out AI-generated spam from authentic user requests, such as time stamps for applications and the ability to quickly match voters’ personal information to government records. The diversity of rules and systems across the fifty states also provides a degree of defense from large-scale attacks.
There was something close to consensus among the surveyed experts, however, that a successful cyberattack on election infrastructure could have serious consequences for public confidence in the electoral process, whether or not it actually affected the integrity of that process. This threat, called “perception hacking,” existed before GAI burst onto the scene. But in an environment where so many people are concerned about AI, the danger to public trust may be elevated. For example, voters who learn that board of elections websites or tiplines have been targeted by a DDOS attack might leap to conclusions that voter registrations or voting machines have been breached, even though those are separate systems that are, in some cases, not even connected to the internet.
Declining public trust may be the biggest threat
The risk of perception hacking via cyberattack and the unrelenting press coverage of the interplay between GAI and elections each contribute to the area of concern that garnered the broadest consensus over the course of this research: the challenge of diminishing public confidence in news, media, and institutions. This problem may seem banal in the context of AI, a technology that is sometimes described as posing an“existential” risk to humanity. But our conversations suggested that it is among the most widely shared and serious worries about AI in the 2024 elections. If it becomes “too cognitively laborious to try and figure out what the truth is,” said one focus-group participant, large segments of the public may simply give up. “The biggest long-term risk is trust,” said another.
AI could diminish standards for verification to such an extent that voters do not trust credible information, increasing apathy and leaving them vulnerable to rumors and lies. The resulting liar’s dividend would allow public figures who are caught up in scandal to dismiss the evidence as AI-generated. Unsure who to believe, the public may shrug and move on while wrongdoers escape accountability.
There is already early evidence of the ways AI tools are adding ambiguity to the political and media landscapes. Trump-allied political operative Roger Stone, for example, has insisted that a potentially incriminating recording of his voice is an AI-generated forgery. The 2023 Argentine general election also featured prominent examples of candidates claiming that authentic videos were deepfakes and, conversely, alleging that deepfakes were real. While denying accusations to get out of trouble is nothing new, the public salience of artificial intelligence might make denials more credible.
The specter of AI-fueled political violence
The shadow of political violence looms over the 2024 elections, with the January 6, 2021 insurrection at the US Capitol and the pair of assassination attempts against Trump by gunmen in Pennsylvania and Florida throwing the threat into stark relief.
The January 6 insurrection occurred because the public was bombarded by a firehose of false claims about election fraud. Repetition is the cornerstone of radicalization, and the repetition of those narratives enabled a broad coalition of people to feel justified in carrying out acts of violence to usurp democracy. The volume of messaging arguably played as crucial a role as the nature of the messaging.
While GAI will likely further undermine trust in the government, media, and elections in the coming weeks, it also will enable actors with limited budgets and/or limited technological capabilities to step into that void with other firehoses of falsehoods. But what remains unclear is whether such escalating volumes of content will prove impactful if the United States already has reached what one participant called “the outer limit of conspiracy theories.”
When asked about the likelihood of election-related violence during the 2024 US elections due, at least in part, to AI-borne disinformation, conspiracy theories, or harmful content, most survey respondents indicated that they believe the likelihood to be high, with relatively high confidence in their assertions.
The risk of election delegitimization is not time-bound; it is constant. Almost all of the harmful uses of AI described above could be just as much a factor after November 5, 2024 as before. While the January 6 insurrection produced calls for extra steps to protect the presidential-transition process, its Brazilian parallel—the January 8, 2023 riots—took place following the country’s inauguration day. Between political cycles, efforts to undermine the legitimacy of elections may be used to justify legal assaults on voting and election administration. In the time before and after an election, they also can inspire acts of violence.
Assessing AI-borne risks to the US presidential election
The sum total of our research—the expert interviews, survey results, focus groups, and review of the literature—is represented in the following chart, which illustrates the risk of various threat scenarios involving GAI use to influence the 2024 US elections. Risk, in this context, is a factor of a scenario’s likelihood and potential impact.
As the chart shows, no threat is viewed as both highly impactful and highly likely. But there are several threats that could pose a more moderate danger and are highly likely, including the rise of AI-generated pink slime, a deepfake “October surprise,” and the fabrication of election-fraud evidence using GAI. As for the risk of AI-inspired election violence, its impact could be catastrophic, but AI is not necessary to spark election violence in the United States. It is also worth considering that these threats, taken together, may be greater than the sum of their parts. Public confidence in the conduct and outcome of the 2024 elections could sag or break under the weight of so many burdens.
These are still early days in the development and deployment of generative AI technology, which means none of this year’s many elections around the world fully demonstrates how GAI will affect forthcoming election cycles or the long-term health of democracies.
The uncertain future of this emerging technology raises questions about what stakeholders should do to mitigate risk, prepare for threats, and safeguard future elections. Self-restraint on the part of tech companies and political operatives will not suffice. A durable, appropriate response will require all of society—including industry, government, and the media—to grapple seriously with the challenge.
Prepare the public without shredding trust or inciting panic
One of the most important short-term priorities for stakeholders to pursue is to equip the public with the information to make sound media judgments in the GAI era. Many of the scenarios our experts rated riskiest involve efforts to mislead the public with synthetic media or AI-enabled disinformation at scale. Unfortunately, legal or technical measures to mitigate these risks at a structural level are unlikely to come in time for the 2024 elections.
In focus groups, however, experts were concerned that, if executed carelessly, public-education and media-literacy campaigns could breed alarmism. They run the risk of backfiring: A frightened public that feels powerless to navigate a world filled with forgeries may simply give up. If that happens, public confidence in institutions and the news media will plummet even further, as will the prospects for holding the dishonest accountable.
As Josh Goldstein, a research fellow on the CyberAI team at Georgetown University’s Center for Security and Emerging Technology, has said: “If we give the impression that disinformation campaigns using deep fakes will inevitably be successful, which they won’t, we may undermine trust in democratic systems.”
In other words, doomsday predictions about the impact of AI on the 2024 elections could become a self-fulfilling prophecy. It is crucial for civil society, the media, and policymakers to take an informed approach to these threats through the US elections and beyond and to establish a culture of competence in place of alarmism. Experts at WITNESS have been preaching this approach for years now as part of their “Prepare, Don’t Panic” initiative, espousing a path to resilience that starts with creating a measured public understanding of GAI.
The rise of disinformation as a top public concern has bred many efforts to educate and communicate with the public, including academic lessons, training exercises, and even games designed to promote “media literacy” (a term with no universal definition but which usually refers to knowledge of how media is created and distributed, and how to discern its reliability). The most effective media-literacy efforts teach participants to use triangulation and other methods to verify information. There is an urgent need for media coverage and public messaging that gently cautions users on how to react if they believe something might be fake, rather than instructing them to live in fear of an unstoppable, undetectable wave of fakery headed their way.
Other efforts have attempted to preempt predictable disinformation narratives through public messaging. Common Cause, for example, prepared voters for delayed election results in 2020 with messages such as “election night is not results night,” attempting to head off bad actors who would use the delay to sow doubt in the election’s legitimacy.
Still more initiatives seek to work with local trusted messengers—described by one focus group participant as individuals and institutions including “little league coaches, rabbis, churches, teachers, [and] community centers”—to communicate important information and counter-messages to the public from the sources they find most credible.
To better prepare and equip the public to navigate the 2024 elections and related AI-borne risks:
Institutions that fund information integrity efforts and connect and convene the professionals responsible for those efforts must continue building resilient, informed communications networks with local elected officials, media professionals, community leaders, educators, and other public stakeholders. These local information brokers have credibility with audiences, making them more effective at communicating with the public about rumors and conspiracy theories. They need to know themselves how to respond to suspected synthetic media or AI-generated disinformation using proven strategies for verifying claims through trustworthy sources, rather than attempting to verify the content itself or simply doubting everything. And they need to be able to pass that information on to others in their networks.
Media outlets and nonprofit institutions should continue to train journalists and news professionals about the most responsible ways to raise awareness of AI-enabled disinformation and synthetic media without stoking public alarm and paranoia. This might include examples of language to use when discussing unverified content or how to report on confidence intervals when forensic experts cannot verify or debunk content with 100 percent certainty, as the Washington Post did when reporting on emails recovered from Hunter Biden’s laptop.
Government officials and journalists should raise public awareness of the many steps—including logic and accuracy testing, requirements for bipartisan staff, secure doors and compartments that require two staffers of different parties to be present before they will open, and post-election audits—that election officials take to ensure the integrity of the vote. This burden cannot fall solely on election administrators, who are already strapped for time, capacity, and resources. Efforts to educate the public—and especially local politicians and community leaders—could improve resilience to claims of election fraud. One method could be to place ads on certain YouTube and Google keywords to proactively anticipate user searches related to fraud and increase the likelihood that constructive content is placed high up in users’ feeds and search results, thereby ensuring there is credible information present to meet them when they search for related news. Social media, technology, and media companies might discount these ads as a public service.
Government officials and tech companies should explore the use of AI tools to make communications with the public more efficient, for example through the creation of AI chatbots capable of referring users to authoritative content about voting and elections. LLMs could also be used to help with ballot proofing and other processes, freeing up election administrators to do more public-facing work in their communities. But, as noted previously, these staff are overburdened already; efforts to test and provide them with new tools should be led by civil society and local, state, and federal governments.
Study and gird for malign actors
Experts have acknowledged that influence campaigns by state actors including China, Iran, and Russia remain a danger in 2024. Both Dan Prieto, a former director for cybersecurity policy on the National Security Council during the Obama administration, and Miles Taylor, Trump’s former Department of Homeland Security chief of staff, are concerned about threats related to cybersecurity, which can include influence operations. As they have written:
“Cybersecurity experts are also worried that AI will make it easier for nefarious actors to tamper with voting systems. For instance, AI-enabled hacking tools could help attackers probe the networks of election administrators, scan for digital weaknesses, socially engineer phishing attacks, and conduct intrusions.”
Many potential threat actors, though, are less sophisticated and well-resourced than foreign governments. They may also have different motives; some simply seek to create mischief. And given shaky public confidence in election systems and the difficulty of proving that a system has not been breached, even unproven claims of a hacking operation could be disruptive.
Actors who exploit AI tools to try to compromise voting infrastructures are likelier to be more sophisticated, purposeful, and destructive than a garden-variety online troll. Yet while those trolls may have fewer resources or be less motivated than those attempting to hack voting machines, they could still have a meaningful impact by creating harmful AI content that propagates easily through our permissive information ecosystem.
To safeguard the 2024 elections against malign actors:
Governments and technology companies should invest more in better understanding the actors who have successfully executed and are most likely to execute socially engineered phishing attacks, an urgent form of risk from GAI. The reported Iranian hacking operation against both the Trump and (now inactive) Biden campaigns only makes this more important.
Researchers, nonprofit organizations, and government agencies should conduct a more thorough investigation into how extremist actors can leverage GAI in the context of the 2024 US elections. The Combating Terrorism Center at West Point and GNET have undertaken initial analyses, but more is needed to answer questions such as: Just how motivated are far-right actors to use AI to elect Trump? Are they organized? Is there a risk of something more than memes and loose claims of fraud? And how will state actors make use of similar tactics to affect both parties’ chances of victory?
Civil-society organizations should engage with a wide range of stakeholders—including election administrators, journalists, trusted community leaders, and technology companies—to identify and prepare for worst-case scenarios. How would they respond, for instance, to a cyberattack on election infrastructure during a crucial recount? What about political violence at polling places in key counties or the refusal of a rogue board of elections to certify the results?
Refine AI trust and safety
Social media platforms remain the most likely channel for the distribution of AI-generated content. Platforms are actively marketing their latest GAI-related capabilities following layoffs on trust and safety teams and while maintaining rollbacks in platform-moderation policies that occurred in the aftermath of the attack on the US Capitol on January 6, 2021. And the information environment is only intensifying as the 2024 US elections near.
Meanwhile, corporate policies are often reactive rather than proactive. When synthetic audio targeted the Slovak elections, it slipped through a policy loophole: Meta’s policy at the time covered only synthetic video, though the policy has since been revised to a labeling approach for all synthetic content. Political deepfakes have been a topic of discussion since at least 2018, but YouTube, for example, waited until 2023 to preview policy changes requiring labels for “manipulated or synthetic content that is realistic, including using AI tools.” Like YouTube and Meta, TikTok also requires disclosure for “AI-generated content depicting realistic scenes.” X maintains a similar policy, though it seems to be inconsistently enforced. Tech companies (including Meta and Microsoft) have embraced strategies for both detecting and labeling AI-generated content and have been providing credentials to authoritative content so it can be verified.
The providers of AI tools themselves have also developed their own trust and safety policies. According to the Bipartisan Policy Center, the model developers OpenAI, Anthropic, and Perplexity maintain policies against political use or manipulation (variously defined). Google prohibits its Gemini chatbot from answering questions about the 2024 elections. Meta, on the other hand, has released its model as an open-source tool, giving the company less control over how it is used. In the summer of 2024, X owner Elon Musk’s Grok chatbot was found to incorrectly answer questions about voting deadlines, but it now directs election-related queries to vote.gov.
For closed-source AI tools provided to clients or users via an application programming interface (API), terms of service may account for the ability to learn from misuse or the failure of guardrails. Anthropic, for example, encourages users, clients, and researchers to make responsible disclosures when they discover vulnerabilities or ways of jailbreaking the app that could lead to harm. The company’s terms of service also specify that user-generated content flagged for trust and safety review may be used to improve detection and prevention of abuse. An expert interviewee pointed out that OpenAI does not appear to have the same provisions in its terms of service. Normalizing these types of data-collection and reporting pipelines could accelerate trust and safety efforts.
Open-source models, by nature, have less ability to restrict the use of their tools or collect data from users. Individuals can, for example, download the Stable Diffusion image-generation model directly to a local device and run it independently of any provider. For some of the most severe transgressions, closed-source developers may filter the data used for future versions of the model (as Stable Diffusion did when known child sexual exploitation material, or CSAM, was found in a dataset used for its model). Altering a model post-training, however, is difficult. After training, models rely less on the content of their datasets and more on the statistical relationships derived from that data. Users can also fine-tune the model post-training using their own data, building back in capabilities limited by filtering.
Other gaps and obstacles confront efforts to create a labeling or disclosure regime for synthetic media on social media platforms. While shared detection and reporting infrastructure between companies and government agencies exists for issues such as CSAM and terrorist content, industry initiatives to identify AI-generated content are nascent. The form and origin of the labels themselves are important. Some are visible to users, while others are meant to be machine-readable. Some are meant to be applied by platforms upon detection of synthetic content, but current detection tools perform poorly. Others focus on “provenance” data, appended to content at the point of creation by the model provider or device. But implementing this consistently requires large-scale industrial collaboration and raises significant privacy concerns. Imagine, for example, if cellphone manufacturers began labeling images from phone cameras in ways traceable back to specific individuals. Finally, previous labeling regimes have raised the specter of an “implied truth effect” in which any unlabeled claim is presumed to be verified. The possibility of an “implied falsity effect,” in which un-watermarked content is presumed false, also exists.
To improve trust and safety practices in the tech industry and update them for the GAI era:
Companies, researchers, and regulators should explore trust and safety provisions that are embedded into agreements between GAI vendors and third parties. As one interviewee put it, the field is “over-indexing on how individuals will use [these tools] on social media” and should instead think more about improving disclosure and analysis of use cases and abuse.
Researchers and government officials should devote more attention to mitigating harm on open-source services. This might include more oversight of the datasets these services use to train their models, as well as more attention to the growing number of commercial services that provide access to these models via an API or web portal.
Industry players should explore collaborative processes and infrastructure beyond labeling and watermarking. Breakout-group participants called for a “shared CSAM-like infrastructure” for dealing with synthetic media, GAI, and election disinformation. Likewise, one interviewee said there is a need for collaboration between social media platforms around the development of concrete threat models for AI-generated misinformation, better measurements of AI’s persuasive capabilities, and defensive uses of AI to combat disinformation campaigns.
Investigators across government, industry, and civil society should continue to use and raise awareness of previous approaches that apply regardless of whether or not threat actors employ GAI. Many of the open-source and technical, closed-source signals that investigators have traditionally used to identify inauthentic accounts, behaviors, and networks online are not content dependent and can be used to detect and curb the distribution of AI-generated disinformation.
The US government and tech companies should collaborate to create a verification standard for authentic communications from official sources such as boards of elections. This is a simpler and more technically feasible solution than a detection and labeling regime for synthetic content. These approaches could be spoofed, but they would at least raise the bar for a bad actor attempting to imitate an official source. Such labels only work if the public is aware of and knows how to interpret them, so they should be accompanied by a robust public communications effort.
Tech companies should measure the risks of over- or under-labeling and their effects on public perception and factor them into their policies for labeling content. This might help mitigate the implied truth and implied falsity effects referenced above.
Update laws, regulations, and norms to shape the use of AI and mitigate harm
AI risk and safeguards are clearly near the top of the policy agenda in the United States and beyond. In June 2023, US Senate Majority Leader Chuck Schumer launched a series of AI insight forums, and the following October Biden released an executive order on “Safe, Secure, and Trustworthy Artificial Intelligence.” At the global level, the World Economic Forum’s 2024 Global Risks Report labeled the use of new technologies to spread misinformation and disinformation during elections as the greatest risk facing the world over the next two years.
Yet given the gap in relative speed between technology development and the legislative process, this policy conversation is still in its early stages. Despite congressional inquiry, the Senate has not yet passed legislation guarding against AI risks. Many other bills have been passed or introduced at the state level. In January 2024, Axios reported that state legislatures were producing AI-related bills at a rate of fifty per week, about half of which dealt with deepfakes. But as outlined here, deepfakes are not the only or most important risk to consider. The need for more proactive policymaking is dire.
At the same time, confronting many of the AI-related election threats that experts fear does not necessarily require laws directly regulating artificial intelligence. As a report from the University of North Carolina at Chapel Hill’s Center on Technology Policy argues, many problems are better addressed through tailored regulation against undesirable behavior, not the AI tools used to conduct it. The New Hampshire robocalls that faked Biden’s voice, for instance, are under investigation for violating older laws governing the use of robocalls for voter suppression. A new law on AI electioneering was unnecessary to deal with that incident.
Other problems would benefit from new laws, but those laws do not always need to focus primarily on GAI. For example, the United States presently does not have a data-privacy law, which could reduce risks from AI-powered hyper-targeted messaging.
To establish better guardrails against AI-facilitated election harms:
Congress should pass a federal law against voter suppression that addresses a significant problem potentially made worse by generative AI. Despite the existence of major voting rights legislation such as the Voting Rights Act of 1965, both offline and tech-enabled voter suppression remain a problem in US elections. A new federal law against voter suppression and deceptive election practices could improve on patchwork state legislation while sidestepping difficult tech-policy questions about artificial intelligence and social media content moderation.
Civil-society organizations should work with the American Association of Political Consultants (AAPC) to create normative guardrails against political uses of GAI. In interviews, many of our expert participants called for more thought leadership and industry discussion on the permissible and impermissible uses of AI in order to help reputable political actors stay within ethical bounds. The AAPC is well-positioned to represent the industry in this discussion.
Regulators should create rules about when disclosure of AI-generated content in political advertisements should be required. Questions to guide this process include but are not limited to: When it is used? What types of actors are using it? For which purposes or in which ways is it used? What forms of AI (for example, AI tools in Photoshop versus GAI-generated deepfakes) fall under these requirements? How might they be enforced? How can they be drafted to survive First Amendment scrutiny?
Legislators should foreground threats to women and marginalized populations in AI risk-mitigation policies and legislation. The use of AI to create nonconsensual sexual imagery of female candidates and to suppress votes of marginalized people are among the most frequently observed political harms from the technology. They deserve to be high priorities for policy responses.
Regulators should create a meaningful transparency regime that allows external researchers to assess, audit, and study harmful trends in the information environment, including through a robust regime for requesting and receiving platform data.
How AI can be used for good in elections
Much of the discourse surrounding AI and the 2024 US elections is centered around threats, but the technology also presents opportunities to improve public services and government administration—including election administration. When asked if there were “any upsides to the use of artificial intelligence in elections, campaigning, and political communications,” expert survey respondents made several suggestions.
Some involve campaign communications. Candidates are already leveraging generative AI to communicate with potential voters and to generate and distribute campaign content. A side benefit is that this frees up campaign staff time—and funds—for other efforts. GAI could also allow polls to include open-ended responses to questions which are then automatically summarized and analyzed by machine, creating a more nuanced understanding of the public and campaigns more responsive to their needs and interests.
GAI could also assist with get-out-the-vote efforts, voter-registration campaigns, and civic education. It might help deliver timely information to voters about polling-booth locations or different ways to cast ballots. Other respondents desired more robust uses of GAI in the context of civic education, such as synthesizing information for voters about various political issues and candidates—specifically highly localized and contextualized information relevant to the voter—and chatbots that could tell voters how particular policies proposed by different candidates could affect their lives.
There are other benefits for election administration, too. Respondents indicated that AI could help with improving signature verification of mail-in ballots and, in turn, potentially increase confidence in election integrity. Multiple respondents focused on reducing the workload for election administrators by, for example, assisting them in determining where to place polling locations and how to allocate staff, or even in better placing precinct locations.
Some states are already experimenting with GAI for public service. New Jersey and Pennsylvania have been notably proactive in enabling state employees to understand and employ GAI in their work responsibly. Of course, the key word is responsibly: GAI “hallucinations” are a very real problem. According to a February 2024 study by the AI Democracy Project, the most popular AI chatbots cannot be trusted to deliver accurate information about elections and voting.
To explore how GAI might provide a net benefit to public administration and election offices:
State and federal agencies should establish pilot programs for responsibly introducing GAI to their workflows. These programs would need to cover potential use cases, the need for employees to supervise and remain accountable for the quality of work products, and safety training on what type of data and inputs are appropriate for use with GAI systems. Artificial intelligence should not be used to make decisions affecting members of the public—it is not a substitute for human judgment—and members of the public should have a right to know when they interact with AI systems.
Civil society and policymakers should monitor and evaluate the role of AI in improving election administration, campaign communications, and other aspects of democratic processes. It is likely that the benefits of AI for these purposes may unfold behind the scenes and may not be readily apparent. If AI indeed empowers voters to receive crucial information about candidates and voting, it is imperative that those impacts are identified and accounted for.
Technology stakeholders, including social media platforms, AI companies, and internet service providers, should establish policies and efforts to prevent AI-related election harms and use their services for public benefit. For example, they might take steps to proactively distribute election information to users, as OpenAI did when it formed a partnership with the National Association of Secretaries of State to empower ChatGPT to direct users to CanIVote.org when asked questions about election procedures.
If the sky is falling, don’t blame AI
Technology is rarely the sole determinant of political trends, and it is easy for tech experts to over-ascribe developments to new technologies. The warning lights around US democracy were flashing before ChatGPT debuted in November 2022. The crisis of public faith in US elections did not result from AI-generated images of ballot fraud. The independent effects of technology are difficult to study and often not well understood. Indeed, most of its effects depend heavily on how it is used, in what environments, and under what regime of norms, laws, and regulations.
Artificial intelligence cannot be blamed retroactively for the myriad challenges facing US democracy, but if those with a stake in US democracy do not respond effectively to the emerging technology, generative AI can deepen them. For now, the best agenda is to prepare the public for a rapidly approaching election in which GAI is already playing its debut role as a salient but not revolutionary source of memes, ads, and conspiracy theories. Those in positions to prepare the public should seize on the surge of interest in the technology not to alarm the voters, but rather to sustain long-term inquiry into the political uses and effects of GAI and evidence-based policymaking to control its worst excesses while reaping its benefits.
Correction: A previous version of this report misstated which Slovak election saw the deceptive use of GAI. It was the 2023 parliamentary elections.
About the authors
Dean Jackson is a nonresident fellow with the Atlantic Council’s Digital Forensic Research Lab, the principal of Public Circle, LLC, and a former investigator for the Select Committee to Investigate the January 6 Attack on the US Capitol.
Meghan Conroy was previously a fellow at the Atlantic Council’s Digital Forensic Research Lab and is a former investigator for the Select Committee to Investigate the January 6 Attack on the US Capitol.
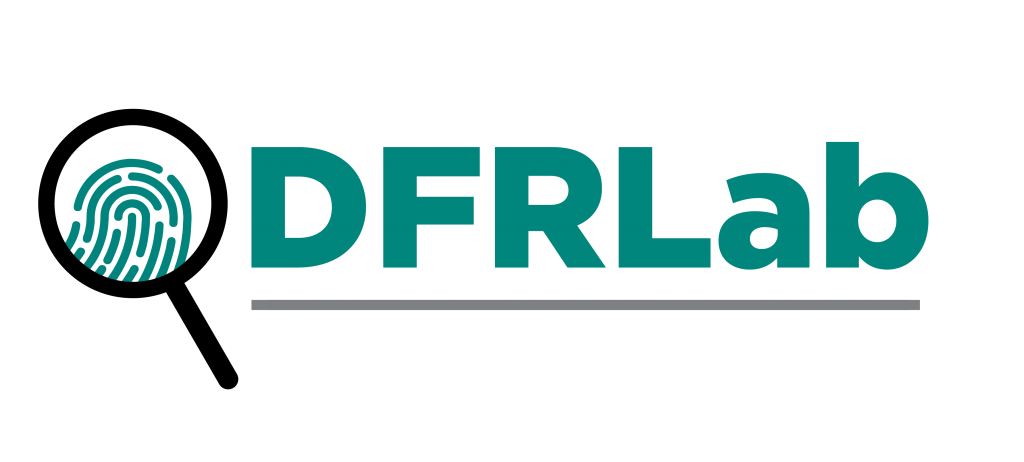
The Atlantic Council’s Digital Forensic Research Lab (DFRLab) has operationalized the study of disinformation by exposing falsehoods and fake news, documenting human rights abuses, and building digital resilience worldwide.