Answer: Ground Truth: A picture of a plantain.
If you are like most people, you thought that the picture was that of a banana. Actually, in this case, you would be wrong (i.e., based on ground truth). This picture is actually a picture of a plantain, a very close relative to the typical banana that you would find at a grocery store. Still not convinced? Please see the side-by-side comparison of a banana and plantain.
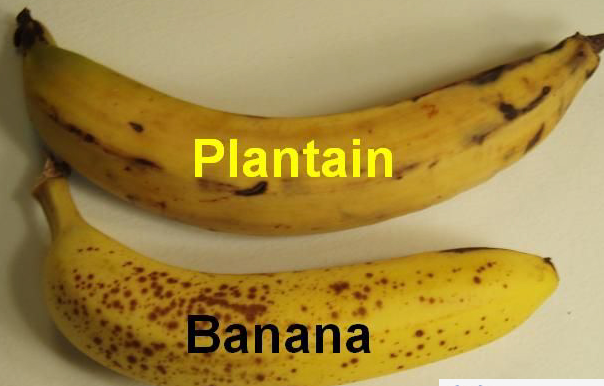
This simple exercise in passing on “truth” highlights the societal and policy challenges pertaining to the classification of what truth is. If misinformation is very close to the truth (e.g., similar to how a plantain has a very close resemblance to a banana), should there be policies that guard against it? From a policy perspective, is slight misinformation just as detrimental as a flat out lie (e.g., trying to convince someone that an apple is a banana)? What if an individual is convinced that what they are communicating is indeed truth (e.g., most people are convinced at first that the plantain is indeed a banana), should there be policies that silence their right to share “their truth”?
These complexities are further exacerbated by the fact that machine learning today primarily relies on the ground truth data provided by humans (for now). Typically, training data that is provided to machine learning models takes a “majority vote” approach to determining what is true. However, in the plantain example above, would more people making the same error have resolved the fundamental challenge of determining what “truth” is? We see that some countries are moving towards policies that hold the information dissemination platform provider accountable for misinformation that is communicated on their platforms. Given the sheer magnitude of information being disseminated on these information dissemination platforms, algorithms are needed to automatically search for and filter potentially fake/inaccurate information. Which brings us back to our quest to train a machine learning model to learn what is true, partially true, and fake. For now, our models are only as good as the data with which they are used to train.
This blog is the second in a series exploring the implications of different policies on the development and deployment of artificial intelligence, machine learning, and cognitive systems. The first blog looked at how cognitive systems may inadvertantly discriminate and what that means for healthcare providers and society as a whole.
Dr. Conrad Tucker is currently serving as a science and policy fellow in the Foresight, Strategy, and Risks Initiative at the Atlantic Council’s Brent Scowcroft Center on International Security. Dr. Tucker holds a joint appointment as associate professor in engineering design and industrial and manufacturing engineering at the Pennsylvania State University. He is also affiliate faculty in computer science and engineering. Dr. Tucker is the director of the Design Analysis Technology Advancement (D.A.T.A) Laboratory. His research focuses on the design and optimization of complex systems through the acquisition, integration, and mining of large scale, disparate data.