Making sense of sensemaking: What it is and what it means for pandemic research
R.J. Cordes is a researcher focused on Narrative and Memetic Warfare, Complex Systems, Knowledge Management Systems, and Cybernetics. He founded the Cognitive Security and Education Forum and contributes research to a variety of working groups and committees across DoD, IEEE, and the Private Sector on topics like Gray Zone Warfare, Knowledge Management Technology, Optimization of Human Learning, and Decentralized Systems.
If this is the first time you have seen the phrase “sensemaking,” embrace this moment of uncertainty with rigor and intention. While we should endeavor to never define a word by using the word in its own definition, we find a rare exception in sensemaking, for in our attempt to define the phrase is an opportunity for the phrase to define itself. Sensemaking is what you are doing at this very moment as you concurrently interpret dozens of symbols by shifting spatial attention at a rate of up to five times per second in order to parse and integrate the information encoded in those grouped symbols using your brain’s complex adaptive network of over one hundred trillion synapses (McGilchrist 2009, Astrand et al. 2015; Spence and Squire 2003). If that last sentence was harder to parse than the one prior, it is because the first was designed with rhyme, meter, and “antimetabole” —in other words, it is built with structure and pattern that builds expectation and reduces complexity, making it far easier for the brain to interpret and make sense of (Leith 2012). The second of the two sentences stresses our sensemaking, as it does not compartmentalize ideas or scope well, instead building an endless scaffold with just enough objects and descriptors to move beyond the capacity of our short-term memory (Benjamin 2007). Our brains are active inference systems like no other, but just as a fish is blind to water until it is stressed by exposure to the surface, we are often blind to our incredible, expansive, awe-inspiring ability to make sense of our environment—until we no longer can.
Just as we cannot fully understand the neuroscience of aggression without first understanding the neuroscience of fear (Sapolsky 2004), we cannot fully understand the neuroscience of sensemaking without understanding the neuroscience of surprise and expectation. Sensemaking is our brain’s response to novel or potentially unexpected stimuli as it integrates new information into an ever-updating model of the world. The brain’s model is generative: we don’t “see” reality—we “see” our visual cortex’s model of the world as informed by memory and sense data. This is the reason we don’t have “blind spots” within our perceived range of vision: even where there are no mapped neurons, our mind fills in the blanks (K. J. Friston, Parr, and de Vries 2017). The principles of model-based, surprise-adjusted inference are fundamental and by no means unique to humans, this being the case, it may be of some value to consider how these principles are used in systems much simpler than the human brain: machine learning and artificial intelligence.
If we look under the hood of most machine-learning technology, there are networks performing what is called “iterative gradient descent,” which is an attempt to take predicted outputs (expectations) and compare them with actual outputs (reality), and then use the deviation between the two to select and adjust the segments of the model that most impacted the deviation between expectation and reality (Bisong 2019). This adjustment intends to increase the clarity of the model’s perception of an event in the interest of reducing surprise when encountering similar events in the future (K. Friston 2010; Heylighen 1993). When this simple process is iterated across large networks with many millions of connections, it can enable computers to reliably detect complicated patterns. Underneath the mystified technologies associated with self-driving cars, chess bots, and automated social media trolls is the application of this very simple, abstract process of gauging and adjusting expectations against contacts with reality, at scale, over a variety of network patterns. The brain does something similar in fine-tuning the connections among its neurons, except the infrastructure and network patterns are guided by billions of years of evolution.
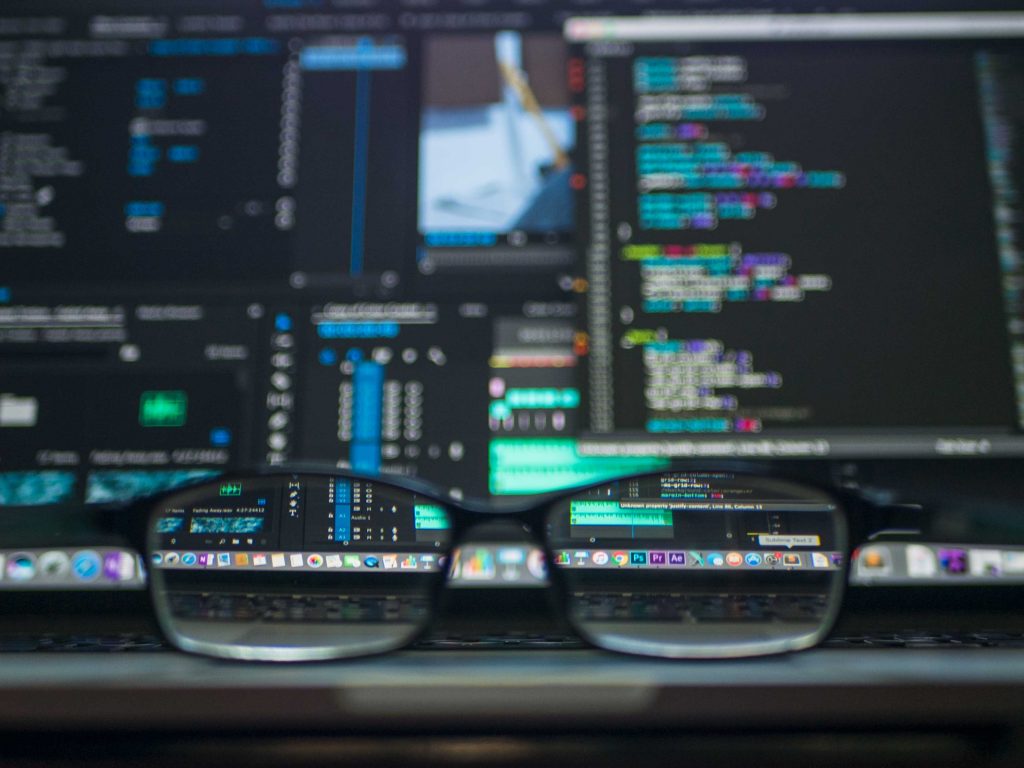
Our brain is performing this gauging of expectation against reality at all times and at a variety of levels, asking simultaneously, “What word am I looking at? What does this sentence mean for my understanding of the world?”. At just the physical level, visual data comes in at a rate and in dimensions that transcend our ability to measure using traditional methods, like frames-per-second, and is accompanied by a notable spatial readjustment at around four or five times per second, and this says nothing about audio (echoic), smell and taste (olfactory), or touch (tactile) streams (Astrand et al. 2015; Spence and Squire 2003). The word “levels” likely does no justice to what it intends to represent, as our body is not a hierarchy but an interconnected, complex, distributed system of systems making sense of multiple non-linear information streams simultaneously. The amygdala is processing physical and hormonal data to interpret threats, and areas of the prefrontal cortex are moderating that sense data based on feedback from multiple memory streams. The kidneys, the pancreas, the hypothalamus, the liver, and the spine are all receiving and providing sense data to facilitate rapid, continuous sensemaking in response to the millions of little surprises the body experiences and creates on a daily basis (Spence and Squire 2003; Sapolsky 2004).
This all being said, while abstract definitions can sometimes become complicated in their attempt to reduce complexity, the abstract definition of “sensemaking” given by Karl Weick in his 1995 book, Sensemaking in Organizations, seems to be quite grounded and down-to-earth:
While the word sensemaking may have an informal, poetic flavor, that should not mask the fact that it is literally just what it says it is.
Sensemaking is literally the act of making sense of an environment, achieved by organizing sense data until the environment “becomes sensible” or is understood well enough to enable reasonable decisions. Organized, Sensible, Understood, and Reasonable—this is the language that characterizes the information environment after good sensemaking has occurred. This definition can apply to all kinds of systems that attempt to model their environment as network patterns—patterns which adapt based on deviations between sense data and expectation. However, it is important to note that these adjustments and adaptations are made on the models for expectations, not on the environment itself. Thus, sensemaking is not decision-making or policy-making—it is the necessary precursor to effective action. Just as sensemaking is the necessary precursor to policy-making and action, a set of trusted streams of relevant, parsable sense data is the necessary precursor to sensemaking—and to collaborative sensemaking, we must add meaningful consensus. We often take these components for granted, and the impact of their absence is observable (Weick 1995; Hodgkinson and Starbuck 2008; Weick 1993; Weick and Sutcliffe 2015).
The last several months of policy and political whiplash of lockdowns, re-openings, and mandates and their accompanying visceral, degenerative public arguments should send a clear signal: the United States is having problems making sense at local and national scales. While some sources suggest we lack the information to make sense of COVID-19 and develop sensible policy, this narrative may not represent the whole truth. As of May, it was being reported that “scientists were drowning” in research on the subject, with an unprecedented growth in published scientific papers (Brainard 2020). Due to the expedited publishing of material already waiting to be reviewed (coronaviruses are not new), in May this growth was alleged to have exceeded four thousand published scientific papers, reports, and analyses in a single week (Brainard 2020). The White House Office of Science and Technology Policy helped organize with publishing houses and tech firms to create a collection of relevant research. As of June, it was deemed the largest collection available (Brainard 2020). Natural language processing on this collection was done by a proverbial dream team of organizations: Google, the Chan Zuckerberg Initiative, and others all in collaboration with the National Institutes of Health, and while the herculean effort was commendable, bibliometric experts—those who use statistical methods to analyze publications and analyses—noted it had critical shortcomings. Nearly 60 percent of the papers in the resulting database were likely only tangentially related to coronavirus, and search functions proved untenable and at times unhelpful. As powerful as AI is, it cannot reliably detect and organize heuristic meaning or perform sensemaking in the way that humans can. Relying on it to do so can sometimes delay or pollute sensemaking efforts more than they help, evidenced by the removal of critical helpful information by Twitter’s automated fact-checking systems. Beyond problems of meaningful analysis, there was also a problem of access: only 67 percent of the aforementioned papers were free to access, while the rest only offered an abstract without payment (Brainard 2020). We can’t make sense of what we can’t see, and we can’t see a needle in a haystack any better than we can see a needle behind one.
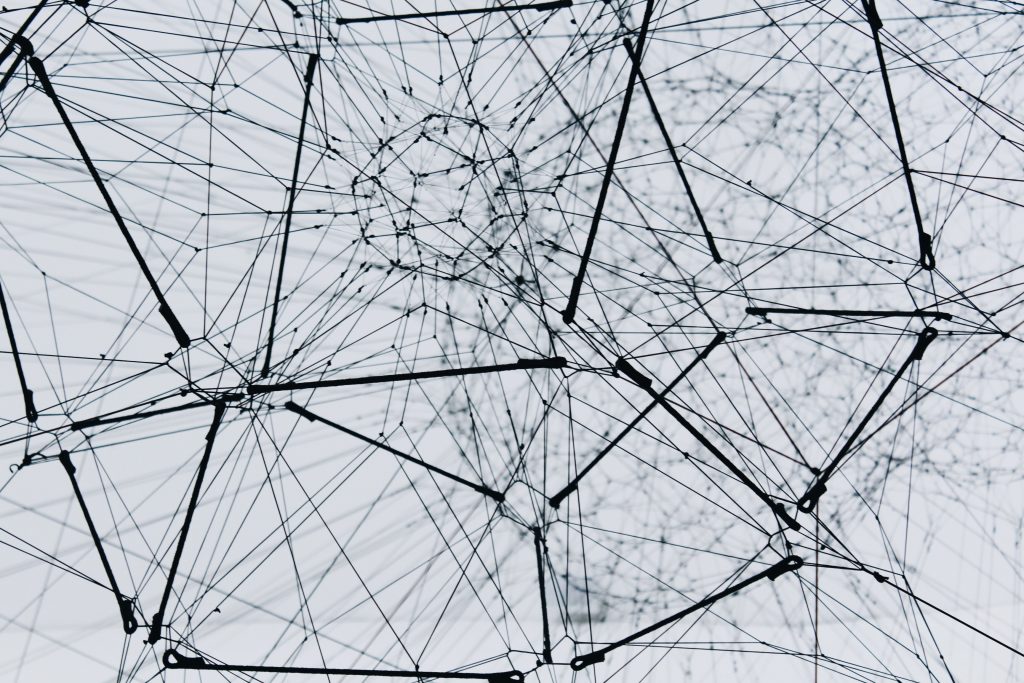
Setting aside problems with access, discovery, and overflow, there was also the issue of contact tracing—here, not of individuals exposed to COVID-19, but of papers, work, and ideas that were built on retracted COVID-19 papers and quality-poor information. The infamous and controversial paper, “Hydroxychloroquine or chloroquine with or without a macrolide for treatment of COVID-19,” published in The Lancet, delayed drug trials and research the world over due to its highly questionable data. The paper was retracted, but not before it infected other research. Many similar papers were retracted or stopped in review when audits on their raw data could not be performed to protect the researchers and institutions against similar retractions. The Lancet itself later retracted several other related papers for similar reasons, but not before many of them were cited. The citation is a tool to allow analysis of a publication’s connection to other research, but from the paper in question we can generally only look back to documents cited, not forward. We can only look forward when we limit our scope to work that was both published through traditional sources and added to centralized databases like Google Scholar, which update metadata as new documents cite old ones, but many documents are not added to such databases reliably. In the age of the internet, social media, and widely decentralized journalism, where more than 60 percent of adults in Western countries use social media as their primary source of news, the problems underlying the impact of the Lancet paper are certainly not exclusive to academic research (Hermida et al. 2012; Bergström and Jervelycke Belfrage 2018; McPherson, Smith-Lovin, and Cook 2001; David, San Pascual, and Torres 2019; Gross 2010).
It’s worth repeating that our brains are active inference systems like no other, but just as a fish is blind to water until it is stressed by exposure to the surface, we are often blind to our incredible, expansive, awe-inspiring ability to make sense of our environment until we are no longer able to do so. It may have been expected that our sensemaking capabilities would have grown lockstep with our advances in information technologies, but the pandemic has exposed this as false. The same requirements for collaborative sensemaking that were present in our hunter-gatherer ancestors are present today—requirements such as trust, meaningful consensus, the integrity and accessibility of information streams, wisdom, comparability of sense data, and a sense of “self-efficacy”, which is a confidence in or ability to make sense of and engage with our environment in a meaningful way (Bandura 1997; Weick and Roberts 1993; Weick 1993; Hodgkinson and Starbuck 2008; Weick 1995; Crichton, Flin, and Rattray 2000; Enya, Dempsey, and Pillay 2019). While the pandemic has highlighted the absence of these requirements, none of these problems are new, in fact, they were normalized prior to the pandemic. As Norman Maclean, the author of an analysis of the Mann Gulch Fire, an event that would be the subject of numerous analyses on breakdowns in sensemaking, once suggested: the ordinary can become monstrous given the right gust. (Bigelow 1992; Weick 1993; Maclean 1992)
As we move toward the end of the first quarter of the twenty-first century, we face complex environments plagued by wicked problems. New threats sit on the horizon—some, the likes of which may have no thematic equivalents in recorded history, while others are threats as old as humanity itself but wear new clothes. Our technology has developed far beyond what our biology has the capability to cope with in absence of radical collaboration, discipline, and responsibility. Yet, as far as this technology has come, it is not a panacea—we cannot externalize the responsibility of solving or making sense of complex problems to AI safely or effectively. For all of this doom and gloom however, it is essential to dispel any broad sense of hopelessness, helplessness, or “effective elimination”, which is a sense of feeling unable to meaningfully engage with the environment around us (Elias, Garfield, and Gutschera 2012; Cordes 2019). In order to do so, we can turn to the timeless words of the twentieth century inventor and systems theorist Richard Buckminster Fuller:
We are called to be architects of the future, not its victims.
If we endeavor to architect such a future, to contribute to effective action in response to modern, complex problems such as food security, peer-adversary conflict, narrative and memetic warfare, and pandemics, we must begin by looking at the precursors to such effective action. We will have to better understand sensemaking: as Socrates once said, “Wisdom begins with the definition of terms.” We will have to make sense of this oft-overlooked root of dynamic human intelligence and its facilitation via technology in larger groups and within complex organizations to overcome a biology that is poorly adapted to the environment it has created. We will have to practice bricolage and always remain ready to improvise and adapt. We will have to accept sensemaking as a tradecraft, as a skill that we never finish learning, a skill that requires good tools, and a skill that we will never be able to fully externalize to machines. The essence of cybernetics, of human-tool interfacing, is not automation, but harmonious facilitation (Norman 2013; Weiner and Wiener 1986). Above all, if we endeavor in earnest to architect our future and to meaningfully engage with and steer complex systems rather than simply allowing ourselves to be subjected to them, then we must all accept the noble call to be agents of change.
Bibliography and further reading
- Astrand, Elaine, Guilhem Ibos, Jean-René Duhamel, and Suliann Ben Hamed. 2015. “Differential Dynamics of Spatial Attention, Position, and Color Coding within the Parietofrontal Network.” The Journal of Neuroscience: The Official Journal of the Society for Neuroscience 35 (7): 3174–89.
- Bandura, Albert,1997. Self-Efficacy: The Exercise of Control. Worth Publishers.
- Benjamin, Aaron S. 2007. Memory Is More than Just Remembering: Strategic Control of Encoding, Accessing Memory, and Making Decisions. Vol. 48. Elsevier Masson SAS.
- Bergström, Annika, and Maria Jervelycke Belfrage. 2018. “News in Social Media.” Digital Journalism 6 (5): 583–98.
- Bigelow, John. 1992. “Developing Managerial Wisdom.” Journal of Management Inquiry 1 (2): 143–53.
- Bisong, Ekaba. 2019. “Optimization for Machine Learning: Gradient Descent.” Building Machine Learning and Deep Learning Models on Google Cloud Platform. https://doi.org/10.1007/978-1-4842-4470-8_16.
- Brainard, Jeffrey. 2020. “Scientists Are Drowning in COVID-19 Papers. Can New Tools Keep Them Afloat?” Science. https://doi.org/10.1126/science.abc7839.
- Cordes, R. J. 2019. “A Closer Look at Effective Elimination: What Happens When Users Feel Incapable of Competing in or Using a System.” Medium.com. August 2, 2019. https://medium.com/@richardj.cordes/a-closer-look-at-effective-elimination-what-happens-when-users-feel-incapable-of-competing-in-or-e964a8eea580.
- Crichton, Margaret T., Rhona Flin, and William A. R. Rattray. 2000. “Training Decision Makers – Tactical Decision Games.” Journal of Contingencies and Crisis Management 8 (4): 208–17.
- David, Clarissa C., Ma Rosel S. San Pascual, and Ma Eliza S. Torres. 2019. “Reliance on Facebook for News and Its Influence on Political Engagement.” PloS One 14 (3): e0212263.
- Elias, George Skaff, Richard Garfield, and K. Robert Gutschera. 2012. Characteristics of Games. MIT Press.
- Enya, Andrew, Shane Dempsey, and Manikam Pillay. 2019. “High Reliability Organisation (HRO) Principles of Collective Mindfulness: An Opportunity to Improve Construction Safety Management.” In Advances in Safety Management and Human Factors, 3–13. Springer International Publishing.
- Friston, Karl. 2010. “The Free-Energy Principle: A Unified Brain Theory?” Nature Reviews. Neuroscience 11 (2): 127–38.
- Friston, Karl J., Thomas Parr, and Bert de Vries. 2017. “The Graphical Brain: Belief Propagation and Active Inference.” Network Neuroscience (Cambridge, Mass.) 1 (4): 381–414.
- Gross, Doug. 2010. “Survey: More Americans Get News from Internet than Newspapers or Radio.” CNN. March 1, 2010. https://www.cnn.com/2010/TECH/03/01/social.network.news/index.html.
- Hermida, Alfred, Fred Fletcher, Darryl Korell, and Donna Logan. 2012. “Share, Like, Recommend: Decoding the Social Media News Consumer.” Journalism Studies 13 (5-6): 815–24.
- Heylighen, Francis. 1993. “Selection Criteria for the Evolution of Knowledge.” In Proc. 13th Int. Congress on Cybernetics, 524–28. Association Internat.de Cybernétique.
- Hodgkinson, Gerard P., and William H. Starbuck. 2008. The Oxford Handbook of Organizational Decision Making. Oxford University Press.
- Leith, Sam. 2012. Words Like Loaded Pistols: Rhetoric from Aristotle to Obama. Basic Books.
- Maclean, Norman. 1992. Young Men and Fire. The University of Chicago Press.
- McGilchrist, Iain. 2009. The Master and His Emissary: The Divided Brain and the Making of the Western World. Yale University Press.
- McPherson, Miller, Lynn Smith-Lovin, and James M. Cook. 2001. “Birds of a Feather: Homophily in Social Networks.” Annual Review of Sociology 27 (1): 415–44.
- Norman, Don. 2013. The Design of Everyday Things: Revised and Expanded Edition. Basic Books.
- Sapolsky, Robert M. 2004. Why Zebras Don’t Get Ulcers: The Acclaimed Guide to Stress, Stress-Related Diseases, and Coping (Third Edition). Henry Holt and Company.
- Spence, Charles, and Sarah Squire. 2003. “Multisensory Integration: Maintaining the Perception of Synchrony.” Current Biology: CB 13 (13): R519–21.
- Weick, Karl E. 1993. “The Collapse of Sensemaking in Organizations: The Mann Gulch Disaster.” Administrative Science Quarterly 38 (4): 628–52.
- ———. 1995. Sensemaking in Organizations. SAGE.
- Weick, Karl E., and Karlene H. Roberts. 1993. “Collective Mind in Organizations: Heedful Interrelating on Flight Decks.” Administrative Science Quarterly 38 (3): 357–81.
- Weick, Karl E., and Kathleen M. Sutcliffe. 2015. Managing the Unexpected. 3rd ed. Wiley.
- Weiner, Norbert, and Norbert Wiener. 1986. “Human Use of Human Beings: Cybernetics and Society.” Avon Books.
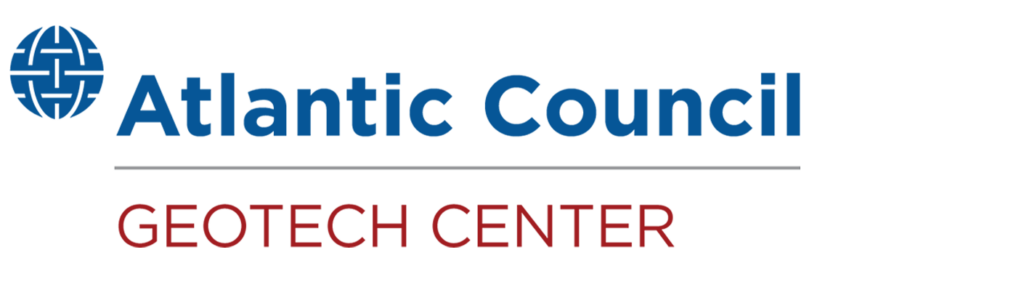
The GeoTech Center champions positive paths forward that societies can pursue to ensure new technologies and data empower people, prosperity, and peace.