Rapidly improving artificial intelligence (AI) capabilities will help accelerate the energy transition. Both established and emergent AI capabilities—such as large language models (LLMs)—can be applied to an array of strategic, technical, financial, and policy challenges posed by decarbonization. It is critical for energy transition stakeholders to monitor, understand, and carefully apply these capabilities to their unique decarbonization challenges, while also addressing the risks involved.
The most consequential new class of AI, generative AI, is able to analyze and create text, audio, code, and even molecular design—doing so faster and often with higher quality than human-created counterparts. Generative AI uses extraordinary volumes of training data and novel data-processing mechanisms which require unprecedented computational power. Data center load growth, driven by a range of factors, is forcing utilities across the United States and Europe to revisit system planning needs. Indeed, this added demand is—in some regions—delaying the retirement of coal-fired power plants. To ensure that climate targets are met, data center growth must coincide with transmission upgrades, energy efficiency improvements, and new low-carbon generation capacity. More broadly, policymakers must also consider how to harness the potential from generative AI while managing complex uncertainties, from inaccurate outputs and data leakage to AI-enabled cyberattacks on critical infrastructure. The deployment of generative AI will require rigorous human oversight, particularly in the early stages.
STAY CONNECTED
Sign up for PowerPlay, the Atlantic Council’s bimonthly newsletter keeping you up to date on all facets of the energy transition.
Given the capabilities of generative AI, integration into organizational workflows can help energy stakeholders in multiple ways—for example, lower regulatory compliance costs, consider strategic planning options, and evaluate the financial risk around their low-carbon investments, among others.
1. Strategic planning
Recent demonstrations of generative AI capabilities are impressive. Generative AI can already outline, summarize, and draft documents cheaper and faster than many humans. It can also help humans conduct strategic tasks more effectively. A study by Harvard Business School examined the effects of GPT-4—the model behind ChatGPT—on knowledge workers’ productivity, finding that GPT-4 significantly improved workers’ abilities to generate effective ideas and develop implementation plans. Another study from University College London found that a collection of LLMs could give strategic recommendations at a comparable level to human experts. As strategic planning use cases are systemic and across industries, improvements in productivity would apply across the decarbonization value chain.
2. Regulatory compliance
Some generative AI use cases will directly enhance clean energy project developers’ ability to manage cumbersome regulatory processes. As generative AI capabilities are integrated into institutional workflows, they will assist on tasks ranging from simple emails to complex, costly, and time-consuming regulatory processes. The Pacific Northwest National Laboratory, as part of its PolicyAI, initiative, recently found that LLMs could streamline the public comment-review process under the National Environmental Policy Act (NEPA), which is burdensome for many renewables firms.
Importantly, generative AI may aid regulators by accelerating reviews of a variety of environmental impact studies. For instance, after New York State attempted to ease traffic and pollution by passing traffic congestion pricing, an exhaustive environmental review took five years and more than 4,000 pages of analysis. By streamlining portions of these document-intensive regulatory tasks, generative AI can speed up environmental reviews, giving infrastructure projects a quicker go/no-go decision.
3. Decarbonization investment analytics
A range of AI tools, using both existing techniques and generative AI, are being developed to assist with financial and economic modeling, a critical but resource-intensive task for renewable energy projects. While still at the early stages, generative AI tools may be able to partially or even fully build financial models or propose complex scenario plans. In addition, AI is already being used to enhance corporate due diligence by detecting anomalies in financial statements, summarizing earnings call transcripts, or rapidly analyzing trade press. These capabilities will continue to assist both investors and corporate mergers-and-acquisitions teams in their decarbonization investments.
4. Energy asset management
Financial and economic modeling tools overlap with another essential aspect of decarbonization: advanced energy asset management. Currently, communications with energy asset field operators are typically executed via middle management and dashboards with both planned and ad hoc analytics. Generative AI may enable more simplified analytics and communication with the workers physically assessing and repairing assets. At the energy asset management level, generative AI tools could deliver improvements in compiling, summarizing, and communicating asset performance in a customized manner for financial managers.
5. Wildfire risk assessment
In parallel to generative AI, another area of quiet yet significant advancement has been machine-learning (ML) models for weather forecasting, which have produced some extraordinary results. Further advances in weather forecasting could help mitigate the climate change-driven fire season. Wildfires themselves exacerbate the climate crisis—global fires produce emissions of about 2 gigatons of carbon dioxide equivalent per year, equal to 4 percent of total global emissions. These fires can also force large populations indoors for weeks due to health risks and poor air quality. Further investment in AI/ML-based modeling could help manage these risks by predicting the probable location and magnitude of potential wildfires and improving real-time surveillance of smoke, enabling firefighters to combat the over 80,000 wildfires that occur in the United States alone every year.
Despite the current AI hype cycle and the early-stage risks around generative AI, improving the broad range of AI models will be integral to developing a low-carbon economy. The magnitude and pace will be difficult to predict, as models are integrated into institutional workflows. Human oversight, particularly around critical infrastructure, must remain comprehensive. If managed appropriately, these emergent capabilities will yield important advances in regulatory analysis, environmental management, strategic planning, and an array of challenges essential to achieving net-zero emissions.
Joseph Webster is a senior fellow at the Atlantic Council Global Energy Center.
Shaheer Hussam is a partner at Aetlan, an energy advisory and analytics firm.
MEET THE AUTHOR
RELATED CONTENT
OUR WORK
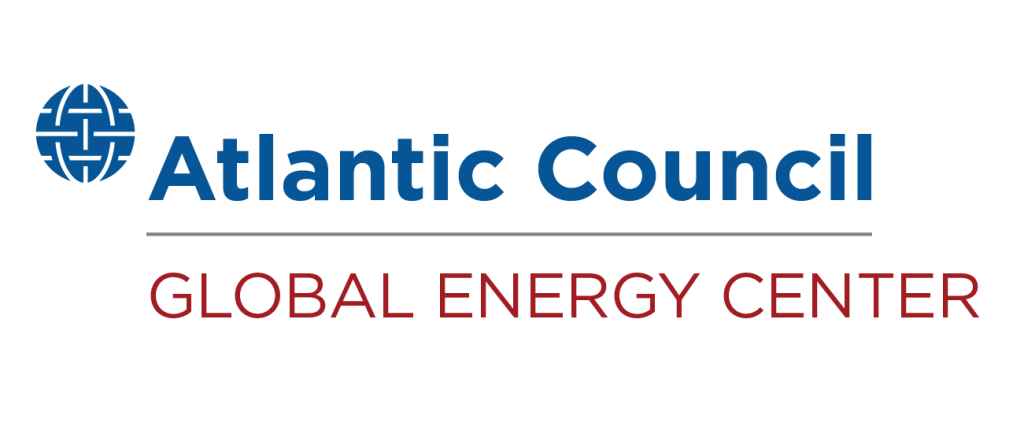
The Global Energy Center develops and promotes pragmatic and nonpartisan policy solutions designed to advance global energy security, enhance economic opportunity, and accelerate pathways to net-zero emissions.
Image: AI chip. (BoliviaInteligente, Unsplash, Unsplash License, https://unsplash.com/license)