What policymakers need to know about artificial intelligence
Watch: Decoding artificial intelligence
Table of contents
- Defining artificial intelligence
- How artificial intelligence works
- Machine learning
- Deep learning
- Global competition and controversies
- Dangers, myths, and misconceptions
Despite an abundance of books, articles, and news reports about artificial intelligence (AI) as an existential threat to life and livelihoods, the technology is not a grave menace to humanity in the near term. Undeniably, the comments of deep learning pioneer Geoffrey Hinton, who resigned from Google, are concerning. “I have suddenly switched my views on whether these things are going to be more intelligent than us. I think they’re very close to it now and they will be much more intelligent than us in the future. How do we survive that?” Hinton said in a recent interview. Hinton fears that AI may come to intentionally or inadvertently exert control over humanity, a hypothetical scenario known as an “AI takeover.” He is also worried about the potential spread of AI-generated misinformation or the possibility that an oppressive leader may attempt to use AI to create lethal autonomous weapons systems (LAWS). But AI programs have no agency to act on their own. Generative AI language models currently operate only within the controlled environments of computer systems and networks, and their capabilities are constrained by training datasets and human uses.
The generative transformer architecture that is powering the current wave of artificial intelligence may reshape many areas of daily life. OpenAI CEO Sam Altman has been making a global tour to engage with legislators, policymakers, and industry leaders about his company’s pathbreaking Generative Pre-trained Transformer (GPT) series of large language models (LLMs). While acknowledging that AI could inflict damage on the world economy, disrupt labor markets, and transform global affairs in unforeseen ways, he emphasizes that responsible use and regulatory transparency will allow the technology to make positive contributions to education, creativity and entrepreneurship, and workplace productivity.
At present, however, Altman’s generative AI is most useful in improving natural language processing and machine translation. Generative transformers are flexible and scalable models that outperform recurrent neural networks—which made voice-activated assistants on smartphones possible—in certain tasks, such as capturing relationships between different words within long documents and answering questions about them. Examples include GPT, BERT, T5, and LaMDA. They do not on their own possess independent capabilities associated with artificial general intelligence or superintelligence, and it is unlikely that a truly versatile human-like cognitive AI will become a reality before 2050. Even an ultrasmart AI program may never bootstrap itself into consciousness. And there is almost zero chance that—as in the Roko’s Basilisk thought experiment—a spiteful and malicious AI will emerge that rewards those humans who assist it and punishes any who dare attempt to stop it. As Sam Altman puts it “GPT4 is a tool not a creature.”
What is certain is that AI tools and methods will be crucial for confronting a slew of slow-motion catastrophes unfolding across the world. COVID-19 has claimed almost seven million lives worldwide, and based on excess mortality likely many more. Strife, stress, and conflict endangers democracies on both sides of the Atlantic. Unschooling and remote work movements mingle with cultural and political divisions and societal disruptions. Climate change brings extremes in heat, drought, and wildfires along with melting ice caps and sea level rise.
Machine learning (ML) is able to tackle these issues head-on. Admittedly, it has a long way to go before it’s a feasible tool for pandemic control, but AI is attaining good performance in the diagnosis, evaluation, and prognosis of infected individuals; predictions of pandemic spread; and COVID-19 drug discovery as well as vaccine development. Responsible applications of AI are strengthening communities and empowering democracies. Over the past few years, this technology played a particularly powerful role in knitting humanity together virtually amidst the spread of disease, snarled traffic, scarce fuel, and the high cost of living. AI-enabled technologies are also monitoring the world’s climate, agriculture, and economies, as well as providing solutions to feed and clothe the world without further damaging the environment. They can facilitate many paths to sustainable planet-wide development. Green AI technologies representative of the convergence of social innovation and technological change include ecobots, biodiversity and ecosystem services, and renewable energy solutions.
Nonetheless, humanity is living in uncertain, complex, and ambiguous times. But as Sun Tzu explained in The Art of War, in the midst of chaos, there is also opportunity. It is not surprising that the current generation has invented AI and social media-fueled empathy scorecards intended to replace or supplement credit scores, prescription video games and other “calmtainments,” and AI-assisted chatbot therapists (Woebot and Wysa). AI is being brought to bear against the labor squeeze and workers’ demands for higher wages, supply chain disruptions and volatilities in manufacturing, and the omnipresent threats of wars of occupation.
Defining artificial intelligence
The ultimate goal of AI is to emulate human-like thinking or perform tasks that normally require human activity. John McCarthy, Marvin Minsky, Nathaniel Rochester, and Claude Shannon, in their original proposal to bring together mathematicians, cyberneticists, and information processing innovators for a formative 1956 summer research workshop on AI at Dartmouth College, contended that “every aspect of learning or any other feature of intelligence can be so precisely described that a machine can be made to simulate it.” AI can be subdivided in several different ways, but the major branches are usually described as artificial narrow intelligence (ANI), artificial general intelligence (AGI), and artificial superintelligence (ASI).
ANI is the branch where the overwhelming majority of AI-inclined developers work. ANI thereby represents the state of the art in AI. It is a category that includes all useful applications of AI to specific problems such as calculating risks and reducing errors, handling repetitive or boring tasks, making informed recommendations or decisions quickly, or automating job duties that are difficult or dangerous. ANI developers are rarely concerned about whether their AI systems are capable of true cognition, awareness, metacognition, or affectivity. Instead, they are content to regard them as models for understanding intelligent behavior and building useful tools. Common areas of activity in ANI are speech recognition, natural language processing, chatbots, search engines, recommender systems, digital assistants, computer vision, image recognition, and machine translation. Every sort of machine intelligence encountered in daily life is limited to specific tasks and knowledge domains that are not readily translated into other tasks or domains irrespective of how sophisticated they are.
On the other hand, AGI and ASI have as their shared goal the achievement of a complete or comprehensive range of human intelligence capabilities, including perhaps even consciousness. In AGI and ASI, the model is the mind; the map is the territory. Should the goal be reached, some researchers believe ASI will surpass human intelligence, with cognitive capacities well beyond those of the smartest people and in a wide variety of domains. An ASI’s “mind” would not just be different in degree from the human one; it would be different in kind. Indeed, some researchers assume that any potential candidate for ASI would require a self-modifying property. Many people, including, most famously, Bill Gates, Stephen Hawking, and Elon Musk, have spoken out about AGI/ASI safety and control. Stuart Russell and Peter Norvig, authors of the leading textbook on AI, note that “[a]lmost any technology has the potential to cause harm in the wrong hands, but with AI and robotics, we have the new problem that the wrong hands might belong to the technology itself.” Others have been more sanguine. Neuroscientist Anthony Zador and computer scientist Yann LeCun, for example, suggest that there is no reason why a machine would develop a self-preservation instinct or evolve into a dangerous competitor.
How artificial intelligence works
The typical AI developer writes code—sometimes employing the assistance of context-aware intelligent code-completion software like IntelliSense or Copilot. At the core of all AI systems written today are intelligent agents. The classical approach to AI is in the form of sense-think-act: agents perceive their environment using sensors, consider choices and make decisions, and react using effectors. They may be physical (robots) or virtual (software) and are often both. Agents now have all sorts of different abilities, goals, preferences, knowledge representations, and memories of past experiences. Humans themselves are considered very complex intelligent agents by AI developers, albeit biological ones. This is why it is sometimes said that the “holy grail” of AI is to understand man as a machine.
Agents are ubiquitous in everyday life. Siri, Cortana, and the Google Assistant are agents, as are tabletop smart home appliances like Amazon Echo, Google Home, Samsung Bixby, Xiaomi Xiao Ai, and Apple HomePod. Agents are also embedded in many autonomous and semiautonomous robotic devices like the Roomba vacuum cleaner, Tesla driver-assistance system, and General Atomics Gray Eagle Extended Range unmanned aerial military drone. Large, pretrained language models are the foundation of the latest—potentially disruptive and transformative—conversational agents like Google Bard, Jasper Chat, OpenAI’s ChatGPT, and Microsoft’s Bing chatbot.
Practitioners of symbolic AI, a dominant early approach to simulating humanlike cognition, compared the brain to a sophisticated computer program. From the mid-1950s and continuing into the 1980s, computer scientists created general and specific problem solver programs. Software developers also created general inference engines upon which specialized rule bases could be applied interchangeably. These so-called expert systems consisted of heuristics or rules of thumb developed from direct interviews with experts and professionals (e.g., physicians, lawyers, mechanics, and chemists). Heuristic programming assumed as a given that an expert is a specialist.
Expert knowledge, however, is rarely fixed. Indeed, it is regularly updated through new discoveries and experience. Heuristic systems struggle to keep up with all but the most predictable definitions and structured reasoning methods. AI researchers describe this as the “knowledge acquisition bottleneck.” Training an AI program to serve as a clinical decision support system, for example, is only feasible if there is a reasonably efficient way to keep up with an exponentially growing reservoir of medical knowledge and know-how. Often, the domain expert and the programmer find it difficult to maintain their systems and keep them current.
Knowledge engineers argue among themselves about whether the right approach is to carefully simulate the reasoning abilities of experts in models of human information processes or rather to discover entirely new methods for weighing evidence that can only be accomplished using computers. Ironically, as expert system prototypes proliferated, they became more specialized, limited in scope, and fragmentary. The history of expert systems has proven that machines, like humans, perform better in specialized domains. Exceptional general-purpose thinking is rare among machines, and perhaps also among human beings.
Expert systems gave way to directly mining the data of extremely large numbers of cases. Data mining requires figuring out how to represent knowledge and extract useful patterns through automatic or semiautomatic analyses so that they might be used effectively. Data mining techniques include cluster analysis, anomaly detection, and association rule mining. This movement away from the primacy of experts has been likened to the demise of the Greek Oracle of Delphi.
By the 1990s, connectionist approaches featuring artificial neural networks (ANNs) eclipsed symbolic AI in popularity. The metaphor for the connectionist approach to AI is the brain as a collection of billions of neurons that both wire and fire together. The application of neural networks to AI also dates to the 1950s but had fallen out of favor until resuscitated by Hinton, the cognitive psychologist who recently left Google, and others who described a new procedure called “backpropagation” for training multilayered neural networks. The connectionist approach became even more exciting as advances in computing hardware and schemes for handling large volumes of structured, semi-structured, and unstructured data (“big data”) made it possible to improve the efficacy of neural networks.
Machine learning
ML today is a subset of AI which relies on both the symbolic and neural network approaches. The synthesis of neuro-symbolic AI and development of hybrid architectures is relatively new. Computer scientists use ML and data analytics to train algorithms and neural networks with statistical methods to discern patterns, make classifications, predict outcomes, and uncover significant insights from available masses of information. In ML, models of learning are used to dexterously organize the capabilities of intelligent agents as they improve themselves using data extracted from online systems or the environment. ML is divided into roughly three categories—supervised, unsupervised, and reinforcement learning.
In supervised learning, labeled data are used to train algorithms. The computer is “taught” to recognize general rules using “training data” (labeled inputs and desired outputs). Supervised learning algorithms may engage in active learning to label new data points with desired outputs, classification to organize data into relevant categories, regression analysis to investigate relationships between independent features or variables and dependent variables or outcomes, or similarity learning, where the goal is to measure the resemblance or relatedness between things.
In unsupervised learning, the algorithm discovers structure, features, and insights from unlabeled data. Unsupervised learning is helpful where common properties of the dataset are unknown or poorly understood. Additionally, unsupervised learning is helpful in solving clustering and association-type problems. Clustering algorithms group data based on similarities and differences. Marketing companies often use clustering and demographic segmentation of customers to identify and group households that are similar to one another in wealth, buying behavior, or lifestyle. These clusters are given names like Married Sophisticates, Penny Pinchers, Skyboxes and Suburbs, Summit Estates, Shotguns and Pickup Trucks, Rolling Stones, Single City Struggles, Aging Upscale, and Timeless Elders. Association algorithms find interesting relationships between variables. Association rule learning can be useful in market-based analyses of customer purchases, allowing retailers to recognize relationships between items that customers frequently buy together and predict the likelihood of purchases of an item based on the occurrence of other items in an individual transaction.
A computer performs reinforcement learning when it learns through interaction with the environment and feedback to achieve a predefined goal or maximize a reward. In reinforcement learning, the AI improves by first making mistakes. Reinforcement learning has applications in teaching self-driving cars to avoid obstacles and stay on the road, training AI non-player characters in video games, and instructing caregiver robots on how to grasp common household objects.
Deep learning
Deep learning is a type of ML that depends primarily on ANNs and training data. The neural networks train by imitating the natural neural interconnectivity of the brain using layers of nodes and connections. These nodes are composed of various inputs and weights, a given threshold, and an output value. When the output value surpasses the predefined threshold, it “fires” like a biological neuron, activating the node and passing data along to the next layer of the network. AlexNet, one of the pioneering technologies in the field of computer vision, was designed by Hinton and his students. This deep learning tool used to analyze visual imagery is composed of eight layers—five convolutional layers, two hidden layers, and one output layer. AlexNet was trained on graphics processing units (GPUs). It outperformed all other challengers in the 2012 ImageNet Large Scale Visual Recognition Challenge. Deep neural networks and platforms are employed in many contexts today; they promote cybersecurity (Deep Instinct), predict criminal recidivism (COMPAS Core), make early diagnoses in oncology (Behold.ai), teach next-gen driverless cars (Tesla, Waymo, Nvidia), and boost the creativity of artists (DALL-E, Stable Diffusion) and writers (GPT-4, Charisma). Generative transformer models are a prime example of deep learning, and they are revolutionary in their ability to quickly find relationships and capture context across large datasets.
Computational creativity is one subfield of AI that has been dramatically reshaped by deep learning. Computational creativity applications attempt to generate original ideas and artifacts. These “generative AI” applications are transforming our understanding of machines as helpmates to humans and altering bedrock conceptions of novelty. Is the goal to replicate human storytelling or to create new media for storytelling? Can an AI agent create a real emotional connection with a person? Can a machine have an original thought or imagination? How would an AI program recognize that something is imaginary? In a world of computational creativity, some common tropes and normative modes of seeing, hearing, and knowing may have to be unlearned.
All sorts of possibilities are being explored with generative AI. The annual National Novel Generation Month (NaNoGenMo) contest is the brainchild of computer programmer and internet artist Darius Kazemi. NaNoGenMo is the artificial spiritual twin of the National Novel Writing Month (NaNoWriMo), a nonprofit organization that encourages human authors to find their voices by banging out drafts of fifty-thousand-word novels in November. Programmers following Kazemi’s rules instead write code that generates fifty thousand words of machine-made fiction. NaNoGenMo provides a standard corpus of public domain lists and texts for rapid prototyping, but participants use all sorts of public domain writings to train their AIs. In the NaNoGenMo submission The Seeker, the intelligent agent is at once algorithm, agent, protagonist, and narrator. The Seeker reads differently each time because the code randomly shuffles in a new selection from its corpus to parse, deconstruct, and reconstruct.
Today, humans and artificial intelligences have joined forces to tell prize-worthy stories like The Day a Computer Writes a Novel, which passed the first round of screening for the Hoshi Shinichi Literary Award in Japan, and 1 the Road published by Jean Boîte Éditions. The author of 1 the Road, Ross Goodwin, was a speechwriter in the Obama administration. Goodwin trained a Long Short-Term Memory Recurrent Neural Network (LSTM-RNN) with three different sets of texts (science fiction, poetry, and “bleak” writings) totaling sixty million words. 1 the Road is particularly interesting because the AI’s input is supplemented using sensors—a video camera, microphone, GPS device, and clock timer—exposed to the sights and sounds of a road trip from New York to New Orleans. Typically, large language models are trained on massive amounts of textual data and can be tens of gigabytes—even petabytes—in size. Researchers are concerned about running low on this kind of data to train models, which means that accessing data from other sources such as audio dialogue, images, spreadsheets and databases, and video clips will become increasingly important.
In this networked world exposure to content is constant. Generative AI promises to exponentially increase the amount created annually. Generative AI applications today are spiritedly responding to an apparent “creativity crisis” among human beings as measured by a thirty-year decline in scores on the Torrance Tests of Creative Thinking, a prominent test for human creativity. Generative AI has manufactured all sorts of objects, discoveries, and/or performances. However, some examples of computer-aided creativity are quite old. One precedent is Alan Turing’s imitation game. Another is the general problem solver of AI pioneers Herbert Simon, Allen Newell, and John Clifford Shaw.
In 1958, Simon and Newell wrote that “within ten years a digital computer will write music that will be accepted by critics as possessing considerable aesthetic value.” This prediction has now been fulfilled by the subfield of generative music and algorithmic composition. One of the most famous examples is David Cope’s Experiments in Musical Intelligence (“Emmy”). Emmy is an algorithmic composer capable of analyzing existing musical compositions, rearranging and recombining them, and ultimately inventing new works that are indistinguishable from those of Johann Sebastian Bach, Frédéric Chopin, and Wolfgang Amadeus Mozart. Shimon at Georgia Tech University is a marimba-playing improvisational jazz-bot musician. DeepMusic.AI, OpenAI’s MuseNet, and the Magenta Music Transformer are all online tools for creating music with deep learning and generative AI. Recently, two programmers have been trying to make music infringement lawsuits obsolete by securing copyright to every combination of eight quarter notes in the C major scale using tones generated with the Musical Instrument Digital Interface (MIDI) standard electronic music protocol. And, similar to NaNoGenMo, a song contest has sprung up that is exclusively for artificially generated music. The first AI “Eurovision Song Contest” winner, the Australian group Uncanny Valley, sampled kookaburra bird calls and koala grunting noises. Additionally, there are AI painters, Dungeons & Dragons dungeon masters, journalists, filmmakers, dancers, stunt performers, and theater players.
Global competition and controversies
A number of countries have established national strategies, initiatives, and funding mechanisms to promote AI innovation and adoption. Former US President Donald J. Trump established the American AI Initiative by signing an executive order in 2019. The order did not allocate any direct federal funding, but it highlighted the significance of employing AI in a responsible manner and taking action to respond to significant investments made by other nations. In 2020, the US Congress passed the National AI Initiative Act. The National AI Initiative (NAII) establishes a coordinated program that spans the federal government. It is aimed at expediting AI research and development (R&D) to strengthen the country’s economic growth and national security. The act provides almost $6.5 billion in funding over five years for R&D, education, and standards related to AI. The National Science Foundation, the Department of Energy, the Department of Commerce, the National Aeronautics and Space Administration, and the Department of Defense will jointly oversee a nationwide network of interdisciplinary AI research institutes.
The US government’s efforts are partially motivated by China’s substantial investments in AI technology. The New Generation Artificial Intelligence Development Plan, announced in 2017, is the Chinese government’s national strategy for AI R&D. China hopes to overtake the United States by 2030 and establish the country as a global leader in the production of AI technology and talent. The major port city of Tianjin in northern China has declared its intention to establish reserves totaling ¥100 billion (equivalent to $15.7 billion) to bolster the AI industry, as well as a separate ¥10 billion fund to advance intelligent manufacturing. China passed a national law aimed at addressing ethical and regulatory concerns related to AI in 2021. In April 2023, the Cyberspace Administration of China issued regulations mandating that content generated by AI must align with the fundamental principles of socialism.
The Russian Federation also has a National AI Development Strategy designed to bolster investment in AI research, education, and industrial development. Somewhat surprisingly, the 2019 Russian AI strategic decree does not mention national defense, though it does emphasize the importance of AI for economic development and healthcare. The decree also does not mention budget, deadlines, or enforcement mechanisms. Due to the recent military conflicts in Libya, Syria, Nagorno-Karabakh, and Ukraine, it is anticipated that Russia will allocate significant resources toward developing AI systems for unmanned aerial drones, counter-drone technologies, and AI-powered surveillance systems.
Significant and unheralded projects are also underway in Africa. The African Union has unveiled an Artificial Intelligence Continental Strategy for Africa, which is intended to facilitate the participation of stakeholders, initiate capacity-building efforts, and fortify regulatory frameworks for AI technology and data management. Artificial Intelligence for Development in Africa (AI4D) is a four-year initiative launched in 2020 by Canada’s International Development Research Centre and Sweden’s International Development Cooperation Agency. The objective of AI4D is to team up with Africa’s government and scientific communities to encourage AI research, innovation, and talent. The ultimate aim is to elevate the standard of living for people in Africa and beyond. African nations are particularly concerned with issues of machine bias and ethics and wary of patterns of manipulation and abuse in the form of automated imperialism, algorithmic colonialism, and digital extractivism.
Canada, Australia, Japan, South Korea, Germany, France, and the United Kingdom also have significant national strategies to address challenges posed by a future empowered by AI. Many of these nations are worried about the likelihood of global competition in AI leading to an arms race or authoritarianism fueled by information technologies. Entrepreneurs, politicians, and engineers warn of an impending “AI Cold War.” An AI arms race to create near-autonomous weapons systems is in full swing, despite being a topic of controversy. The banning of these so-called killer robots may not even be practical. Governments around the world have developed a number of other controversial applications of AI, such as image recognition and mass surveillance, predictive policing, deepfakes and misinformation campaigns, and social credit scoring.
Dangers, myths, and misconceptions
AI can be destructive even when used as an instrument for creative discovery. One of the dangers of unleashing computational creativity tools is being submerged by a culture of automation that dampens individual creative expression and dialogue with human audiences, participants, and partners. In 2022, an AI-generated artwork took first place in a fine arts competition at the Colorado State Fair, which outraged many. Only months later, an internationally acclaimed photography competition—the Sony World Photography Awards—was won by an image generated using AI. Getty Images and established art communities are refusing to accept AI-generated masterpieces. But in general, AI is valuable because it empowers humanity with tools that extend bodies and minds and mitigates risks and perilous circumstances.
Deep learning pioneer and serial entrepreneur Andrew Ng has said that worrying about AI is like worrying about overpopulation on Mars. Artificial agents will not need to be excused or incarcerated for crimes and misdemeanors that upon analysis and reflection can be traced to human error, indifference, or greed. Whole brain emulation, artificial consciousness, technological singularity, and AI apocalypse are all well over the horizon. The threats that remain are still significant. The chief near-term dangers of AI technology are pervasive and more subtle. They include risks such as over-optimization, weaponization, deception and distraction, complexification, moral and practical deskilling, amplification of competition and conflict, job losses due to automation, and harms to human uniqueness, privacy, and accountability.
What lies behind the hype and fear of AI is a fundamental misunderstanding of current objectives, as well as severe shortsightedness. Most AI is meant to supplement human intelligence, not replace it. AI is intelligence augmentation until—and only if—humanity commits and finds ways to entirely remove human beings from the loop as creators, controllers, and decisionmakers. “Exiting the loop” will prove difficult: Humans are extraordinarily skilled at handling ambiguous situations, such as intuiting the emotional state of other drivers on the road. AI will not become human-like merely because humans anthropomorphize it either. An AI program does not try to learn (although it can improve through reinforcement learning methods); it plucks statistical patterns and distributions from training data using pipelines, algorithms, and parameters unglamorously selected behind the scenes by programmers. ANNs are not reasoning the way brains do, and adversarial ML involves no clashing of titans. Thinking about the past, present, and future of AI is imperative. When IBM said that the Jeopardy!-winning Watson AI would also revolutionize medicine, it in effect denied a century of hard-won gains in health informatics R&D (and has yet to achieve its lofty promises). It is not possible to simply wave our hands and say that quantum computing, DNA data storage, and neuromorphic chips will pave the way for an AI-infused next industrial revolution. Real progress in AI comes much more slowly, albeit with occasional surprising leaps forward, and ultimately depends on the real wants and needs of human beings.
Philip L. Frana is an associate professor in the Interdisciplinary Liberal Studies and Independent Scholars programs at James Madison University. His scholarly interests focus on the social and cultural aspects of robotics, automation, and information technology.
Related content
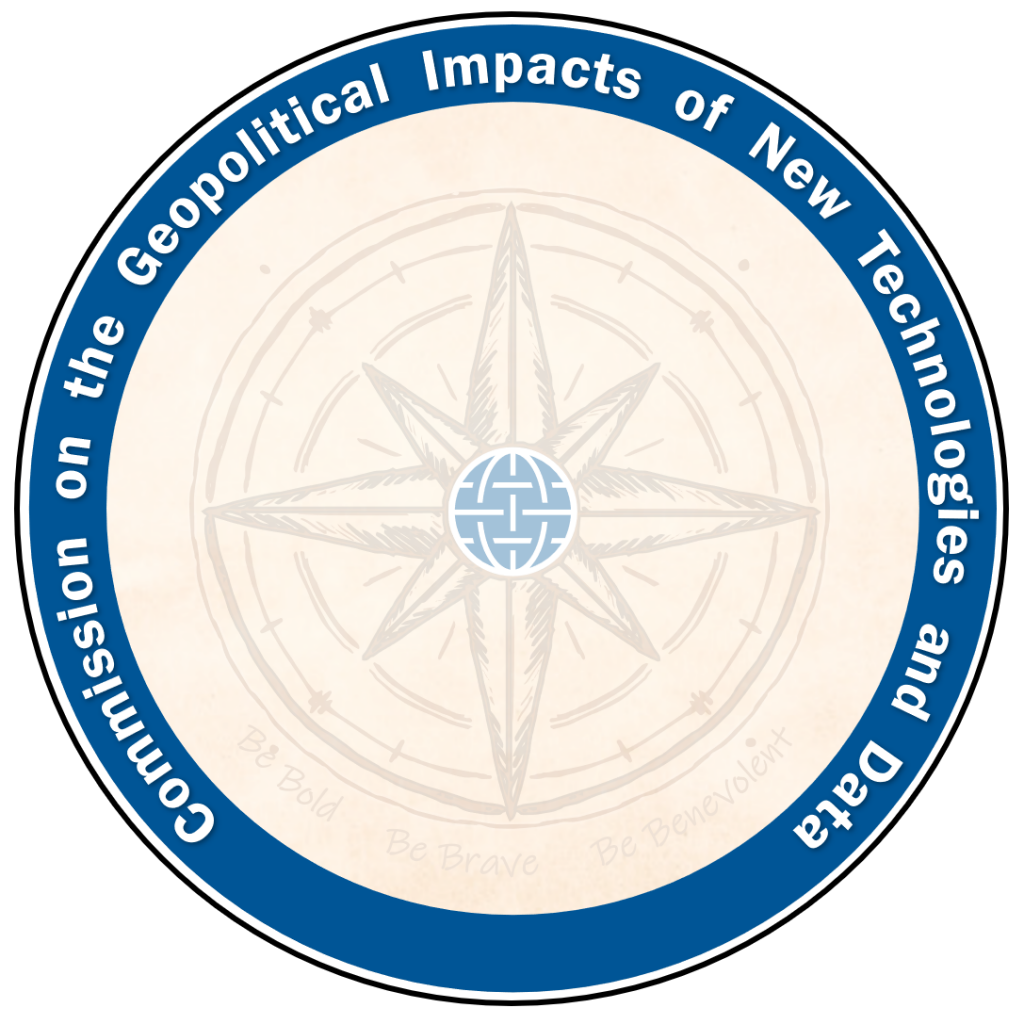
GeoTech Center
Championing positive paths forward that societies can pursue to ensure new technologies and data empower people, prosperity, and peace.