#GoodTechChoices: Addressing past and current racism in tech and data
The Atlantic Council GeoTech Center’s #GoodTechChoices Series seeks to provide public and private sector leaders insight into how technology and data can be used as tools for good. In this analysis, the GeoTech Center examines how tech and data communities can accidentally be racist in the products and services they provide, and what tech and data leaders who care can do to re-envision more inclusive, diverse, and just practices.
This article examines what the data and tech communities can do about racism, i.e. differential treatment based on how people present, such as their skin color, name, and other markers of not being what ‘white.’ Leaders should care about stopping racism because it limits all the systems it touches: it limits individuals. Racism in tech and data also limits teams and technology markets and makes companies and countries weaker.
Introduction
Racism can be viewed as a system problem, but it’s also very much about people. Racism is everyone’s problem, but to address it, we should start by listening to Black people and acknowledging that white people have to do a lot of the work. Some of the excellent BIPOC (Black, Indigenous and People of Color) people working on the intersection of racism and tech include Charlton McIlwain (“Black Software” and an interview) and Anna Everett (Digital Diaspora) on Black people’s relationship with the Internet and other technologies; Minda Harts (“The Memo”) on business strategies; Ruha Benjamin (“Race after Technology”) and Safiya Noble (“Algorithms of Oppression”) on how technologies encode and deepen racist policies; Claude Steele (“Whistling Vivaldi”) on the damages that stereotypes do; and Clyde Ford (“Think Black: A Memoir”) on his father’s and his experiences of being Black in a large tech company.
A hacker, in the old sense, is someone who takes apart systems, thinks about how they succeed and fail, and puts them back together again in new ways. So in a time flooded with articles about what racism is, its history and failures, we must think about the underlying system. Racism is at heart an ethical problem, where ethics is defined as the coded behaviors by which people live within communities (Singer’s Practical Ethics is a good guide to preference utilitarian ethics). Ethics can be codified as a risk problem: there’s a system, and there are harms, likelihoods of harms, and affected populations—in other words, who does what to whom and how likely, and bad is it? When considering racism from this lens, we need to ask the following: what is the system, what are its harms, what are their likelihood, who is affected and how, and how do we address each part of these risks?
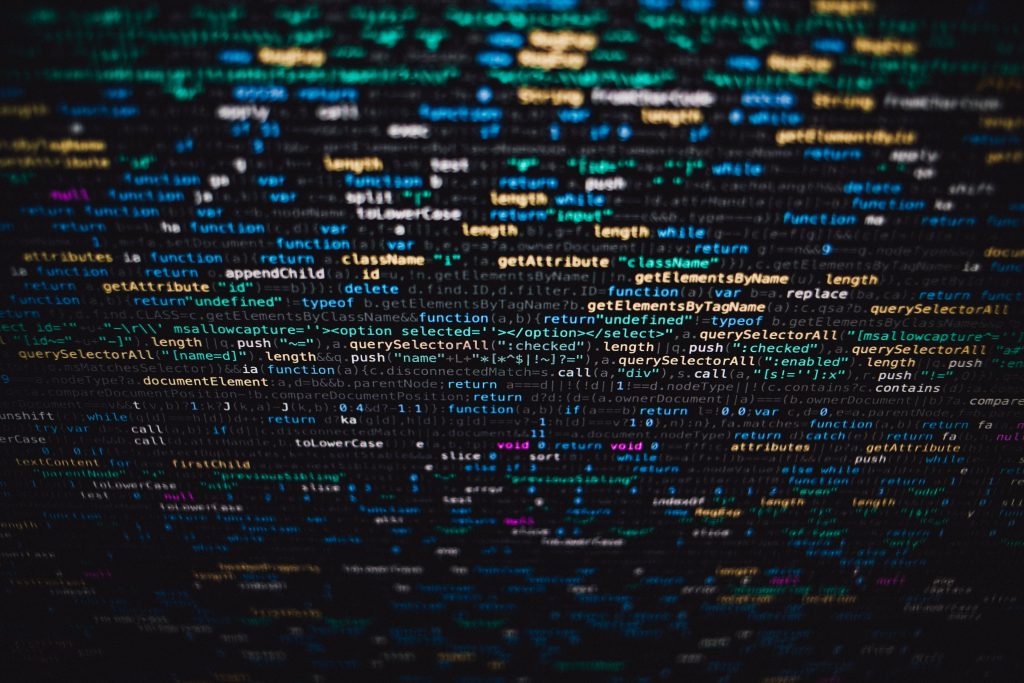
Recognizing racism in data and tech
First, what, exactly, is racism in data and tech? Racism is not just white women calling the police because Black children are walking near their house. It’s not just something individuals do and can be corrected for. It includes concepts like structural and institutional racism, in which the way people are treated differently is built into the systems around them, usually so subtly that dominant groups don’t notice it, including in the ways that they define racism itself. Kennedy Mitchum recently called out Merriam-Webster’s definition of racism, adding “prejudice combined with social and institutional power. It is a system of advantage based on skin colour.” I’m going to use that system definition here.
Next, what is the boundary? When we discuss racism, data, and tech, what do we mean? I think of these things within technologies:
- Technology that’s accidentally racist because it’s designed and tested in a monoculture or from within a system that doesn’t know it’s structurally racist.
- Technology and data science reinforcing racist biases but being treated as neutral because it’s technology.
And these things within technology organizations:
- Technology companies treating people working with them differently because of the way those people present.
- Technology organizations creating a different experience for people interacting with them and their technologies because they contain structurally racist design elements.
None of this is good, and attempts to fix parts of these systems have been made over the years with varying success, reversals, and recriminations.
Accidentally racist technologies usually happen because their design teams were poorly trained or didn’t think or care about adverse effects on non-dominant populations. These are problems of knowledge and power: the teams with design authority aren’t knowledgeable enough or diverse enough to notice problems before shipping, or they don’t have the power to advocate for inclusive designs.
Examples include consumer sensors not recognizing Black skin, camera film tuned to white skintones, speech recognition not understanding underrepresented accents and speech patterns, and white skin as the default or only option in emojis.
We are going to need to a) avoid creating technologies like this, and b) recognize when it’s happening so we can call it out. We can fix knowledge gaps by making demographics more visible to designers – simple solutions such as:
- Better training for the people who create technology. Consumer equipment designers knowing basic race differences (e.g. that different skin types have different reflectance properties on different sensor types) either as part of their training, as product checklists, or encoded in product acceptance checks and regulations. This requires checklists of known race interaction differences: a first set could be created from existing examples of failure.
- More diverse teams. An easier way to have designers know about and test on basic race differences is to build more diverse technology design teams.
- Better feedback loops for when things do go wrong.
Addressing the power dynamic is more difficult: a product company might decide to ignore the needs of 10 percent of its consumer population. This usually means introducing new company goals alongside its market goals, either by building diversity into their systems or by meeting regulations that force this. Regulations are a difficult subject: finding an acceptable set of regulations to build anti-racist systems is going to be a delicate art.
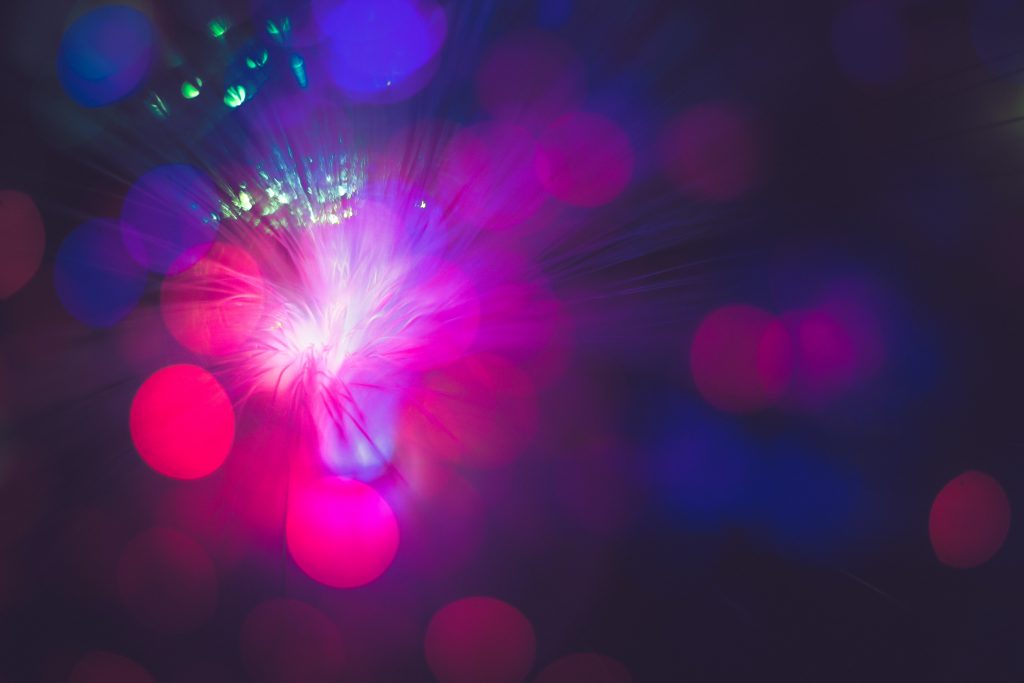
Technology encoding and reinforcing racist human biases
Technology can reinforce existing racist biases in two main ways: either by encoding them in new algorithms (e.g. machine learning systems fed biased input data) or by enabling interactions that are negatively based on race based on the way that data and technology interfaces are presented. New technologies in particular generally go through a hype cycle in which people distrust them because they’re new, then over-trust them, then distrust them again because that over-trust failed, and eventually learn to work with them as tools but not replacements for wisdom. Many everyday technologies like the internet, mobile phones, and the apps and other interactions built on them) are relatively new and still in the early stages of that cycle.
One of the surprises concerning artificial intelligence (AI) research has been how the technologies we build, and specifically the algorithms that we build, reflect the historical and societal biases in the datasets that we train them on. Examples of this include the way historic redlining has made mortgages harder to obtain for people whose ZIP codes are in areas with larger Black populations, Microsoft’s Tay chatbot descent into sexist and racist responses after being trained on social media data, and racist image labels used in machine learning algorithms. Crime is a particularly difficult area. Racist biases persist in systems that calculate recidivism (the probability that someone sentenced will reoffend) and in predictive policing algorithms based either on police practices that have disproportionately targeted people of color, or on falsified data. Class imbalances (e.g. having too few training images of people of color) in AI systems that are then used by police who are unaware that class imbalance biases system results can also cause issues, in police and other systems. These practices create feedback loops in which racist policing and sentencing practices create biased datasets that are used in crime prediction and change policing and sentencing outcomes.
The ways that systems present data and choices to users can also trigger and amplify peoples’ implicit racial biases (e.g. software defaulting detained individuals’ race to ‘Black’). Different word choices can also trigger biases, ranging from the words used to describe slavery to the power-based word choices (e.g. master-slave) in technical designs.
Technology has been used to weaponize race and attack specific communities, as seen in disinformation campaigns focused on Black Americans. Even measures added to reduce racism can be problematic. For instance, Black people are more likely to be blocked than white people on some social media systems, including in their own conversations about race. (It is unclear why—are filters picking up keywords or a style difference? Subconscious bias from editors? Or, biased users reporting posts from Black people more often than posts from white people).
There are solutions here too:
- Stop basing the prediction systems that affect peoples’ lives on racially flawed data. The responsibility here will probably fall on the people who create technology. It can be difficult to judge, so designers could research past solutions and explore ideas like creating checklists of ways to sample and test for bias such as listing potential proxies for race like ZIP codes, names, and stated race, and checking system outputs against base population demographics. Examples of this in practice include NIST’s Face Recognition Vendor Test (FRVT).
- Raw input into systems must be critically examined. For ‘standard’ datasets that affect peoples’ lives, either as inputs to commercial or government system, check and correct for racial biases if possible, and drop the datasets if not. This can be done: I worked on image processing for decades before the much loved but very sexist ‘Lena’ image was dropped from academic work. Again, we have examples of failure: 80 Million Tiny Images and others that we can start to build checklists from. A shortlist would include things like the image labels returned for non-white-appearing faces, differences in data returned with non-white-appearing accounts (e.g. different adtech and search results), where appearance includes visual images and names.
- Find ways to correct problematic parts of biased systems, e.g. identifying why systems have uneven class numbers and either create ways to correct for those imbalances or remove the systems containing them from use.
- Use known biased systems and historical data, fed into machine learning and AI systems, to learn more about human biases and the ways they manifest.
- Remove racial bias from the decision making parts of user-facing systems, or create user experience designs that nudge users away from it like Jennifer Eberhardt’s work on creating useful friction in Nextdoor reporting, and AirBnB’s Project Lighthouse work removing racial indicators from the earlier steps of the booking process.
Issues like class imbalance come with sub-issues. Data about people of color might not be available because of biases in the people collecting the original data. For example, data collectors might not be monitoring for and changing collection plans to address dataset feature gaps including race. Those datasets might also not be available because of reluctance of people of color to be included in data collection. There is history in the United States and other countries of egregious acts against non-white bodies—the Tuskagee syphilis study, the Puerto Rico contraceptive experiments—and what Safiya Noble calls “data disposable” people. There is also a long history of new products being tested in Africa before release in Western markets.
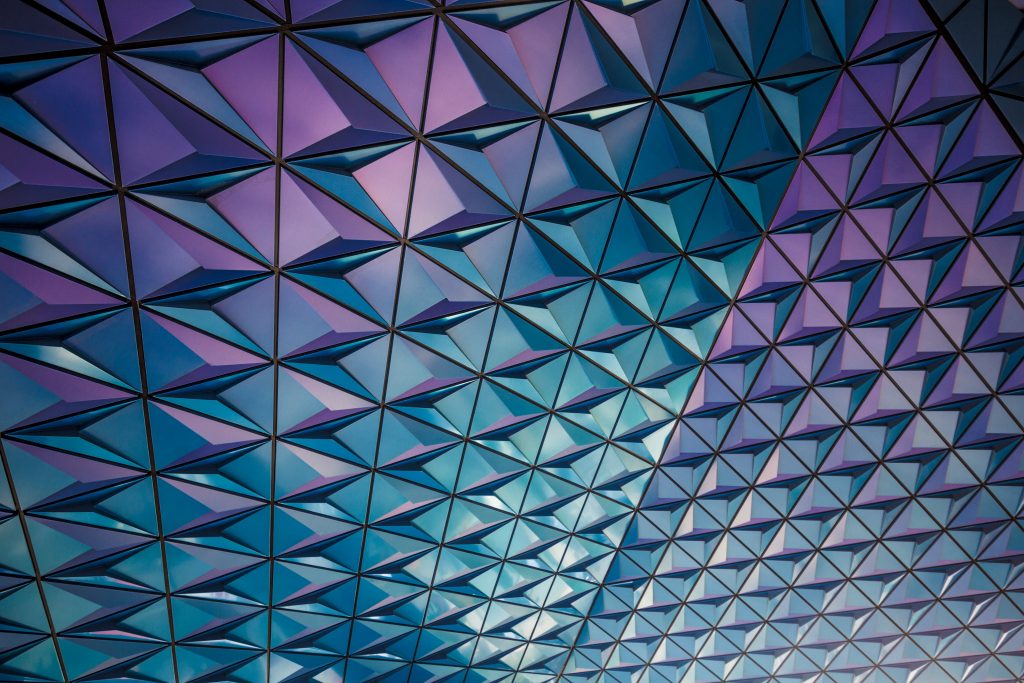
Racism in workplaces
Racism, like sexism, exists across the tech sector. It exists in hiring systems and pipelines into them (e.g. in tech education and computer science departments that feed into tech companies), in how people are treated differently while navigating both space and advancement systems (e.g. promotions and VC funding). Other examples include people with black-looking names getting fewer interviews than white-sounding names, sometimes because of biases in the applicant tracking systems, people of color being continually challenged on status with “you don’t belong here” signals like “bias in badging” (being stopped from entering an office based on appearance), under-representation in lead roles, lower chances of receiving venture capital funding, and discrimination based on imported racist systems like the Indian caste system. The tech industry’s belief that it is a meritocratic system is used to justify these behaviors, exacerbating the problem.
It isn’t enough for an organization to talk about defeating racism, or to use Black and brown faces as part of its image (“blackfishing”). Fixing this is more than an equality statement, an unempowered diversity director, and a donation to Black Girls Code. An organization also has to be anti-racist in all parts of its practice (see for instance the experiences of Ifeoma Ozuma at Pinterest). There are many initiatives on equality, or rather equity, in workplace representation and agency. Some of these include listening to and acting on racial bias concerns and overhauling hiring practices to remove hidden bias, which- includes checking language used in job descriptions, outreach, salary history bans, and bias awareness tools. Advocacy and training groups include re:Power and Color of Change.
Groups focused on Black tech communities include Black Code Collective, Juneteenth Conference, BYP Network (“the Black LinkedIn”), and Frauvis, but again racism is a white-person problem whose consequences fall heavily on people of color, who are often asked to help solve these issues whilst they’re affected by them. We are all countries, and communities, regardless of how we present, but the job of working against racism in those, including in the workplace, should fall more heavily on white people’s shoulders, starting with simpler tasks and introspection.
Some countries, notably the United States, have complicated histories around race— racism there is largely created by white people, largely affecting Black people. This outer environment cannot be ignored in discussions on the interactions between race, technology, and data. It leaves traces in both systems and in the interactions of non-white people with those systems and the companies that build and work with them. These can be overt, or they can be subtle representation cues, such as walking into a reception and seeing only white faces in the pictures on the walls, which manifests as missing people and cultures.
The flip side is that data and technology can also help work to reduce structural racism. It can be used to highlight problems in perception (e.g. Color of Change’s analysis of police procedurals), empower communities of color, or check for racial bias in areas like COVID-19 treatment (e.g. The COVID Racial Data Tracker). As technologists, we can look at all parts of the systems above—people, processes, technology, culture, and power—working out how they go wrong and how to start fixing them or amplifying people already doing this work. We can look for and measure things like data voids and suggest how tech can either highlight or help fill those voids. Countries like the United States can use reverse innovation to learn from and reuse work in other places.
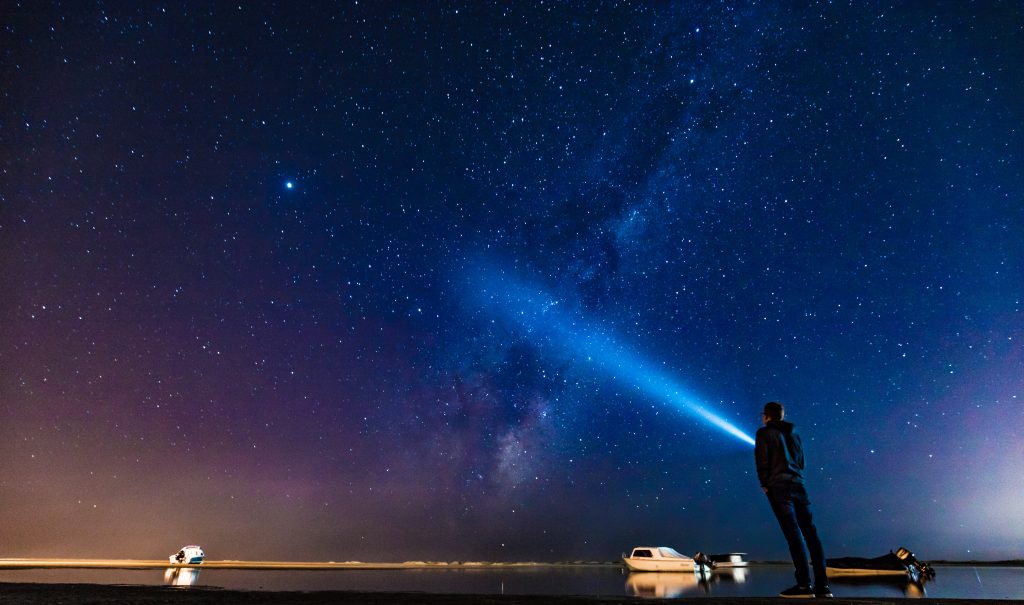
Where do we go from here?
One of the best defenses against biased technologies is a diverse, empowered technology workforce. Homogeneous systems are fragile: racism hurts not just individuals but also the systems they live within. Society needs to understand and move away not just from racism but also towards a positive anti-racist culture. This will require built-in feedback cycles, policy changes, and introspection about how racism, data, and technology interplay. It will also require systems thinking around managing and reducing backlash against anti-racist behaviors and policies. Data and technology, and the companies and people around them, have a large part to play in this. Ways to make positive change now include:
- Check new technologies against a basic awareness checklist. For instance, if they use sensors, have they been checked with different skin types? If they’re making decisions on people, are they using proxies for race (e.g. ZIP codes)?
- Build and use tools like the Face Recognition Vendor Test, to surface any race-based issues in tech.
- Probe training datasets for biases (and build probe datasets for this if they don’t yet exist).
- Listen to people of color in the workplace, but don’t expect them to do the second job of managing anti-racism on top of their day jobs: either create new roles or use specialist organizations for this.
- Flip the script. Think about how data and technology could be used to help people and organizations become anti-racist.
Further reading:
1. General environment around racism
Defining structural racism
- Structural Racism, Keith Lawrence, Terry Keleher, 2004
- The Dictionary Definition of ‘Racism’ Has to Change, The Atlantic
- Understanding systemic racism and how to combat it, Interview with Eleanor Seaton
- 11 Terms You Should Know to Better Understand Structural Racism, The Aspen Institute
- Words, etymology,etymology, and why it’s hard: The meaning of BIPOC, as explained by linguists, Vox
- New Discourses
Where the United States has come from
- An Indigenous Peoples’ History of the United States (REVISIONING HISTORY Book 3)
- Facebook Post, America peonage history
- Myths within myths, the United Kingdom and the reinvention of the Edward Colston statue story
Where the United States is
- You Want a Confederate Monument? My Body Is a Confederate Monument, New York Times
- How A Mother Protects Her Black Teenage Son From The World, NPR
- Cops Fired for Using Extremely Racist and Violent Language, Media ITE
- Facebook Post, Racial Gaslighting 101
- Twitter Post, Symbols vs. Systems
- Atoning for Racism’s Toll on Older Black Americans – existing policies
- Facebook Post, Amazon Package Story
- Kicked off The Land, The New Yorker
- My Family Saw a Police Car Hit a Kid on Halloween. Then I Learned How NYPD Impunity Works. ProPublica
- Angela Davis vs the Liberal Reformers, Existential Comics
Where the United States is going
- For the first time, the majority of people under 16 in America are nonwhite and Hispanic, Fast Company
- McGirt v. Oklahoma
- Farming While Black
- ‘We’re tired of waiting’: GenZ is ready for a revolution, CNN
Things that might help
- In Germany, Confronting Shameful Legacy Is Essential Part of Police Training, New York Times
- What Are you Optimizing For? Building Better Recommenders, Partnership on AI
- Reset: My Fight for Inclusion and Lasting Change, Ellen Pao
- How To Overcome Race Barriers In Medical Training: First Acknowledge Them : Shots, NPR
- Black medical student creates a handbook to show how symptoms of disease appear on darker skin after he was only taught how to diagnose conditions on white patients, the Daily Mail
- Principles for Accountable Algorithms and a Social Impact Statement for Algorithms, Fairness, Accountability, and Transparency in Machine Learning
2. Tech racism problems
Biased tech
- Speech Recognition Tech Is Yet Another Example of Bias, Scientific American
- Over 1,000 AI Experts Condemn Racist Algorithms That Claim to Predict Crime, Vice
- Detroit police chief cops to 96-percent facial recognition error rate, Ars Technica
- Police across the US are training crime-predicting AIs on falsified data, MIT Technology Review
- MIT apologizes, permanently pulls offline huge dataset that taught AI systems to use racist, misogynistic slurs, The Register
Tech reflecting existing racist biases
- The Racial Bias Built Into Photography, New York Times
- Of course technology perpetuates racism. It was designed that way. MIT Technology Review
Tech being used to deepen racism
- Black YouTubers sue Google, CNet
- GENEROSITY AND CONVIVIALITY IN THE AGE OF ALGORITHMIC OPPRESSION, Open Data Manchester
- Control and Freedom, Wendy Hui Kyong Chun
- Digital Diaspora, Anna Everett
- Canaries in the Coal Mine: COVID-19 Misinformation and Black Communities, Brandi Collins-Dexter
Workplace racism in tech companies
- California sues Cisco for bias based on Indian caste system, AP News
- Twitter Post, Leaving Pinterest
- Black Tech Employees Continue to Face Workplace Racism
- Think Black: A Memoir, Clyde W. Ford
- Facebook while black: Users call it getting ‘Zucked,’ say talking about racism is censored as hate speech, USA Today
- Charles Bolden, NASA’s first Black administrator, speaks out on systemic racism, Space.com
3. Potential solutions
- Facebook Post, Replacing Words
- A new way we’re fighting discrimination on Airbnb, Airbnb
- In a U.S. first, California city set to ban predictive policing, Reuters
- “We’re in a diversity crisis”: cofounder of Black in AI on what’s poisoning algorithms in our lives, MIT Technology Review
Doing good with tech
Data used for good
- Confederate Legacy Tracker, Southern Policy Law Center
- List of Confederate Momunments and Memorials, Wikipedia
- Hollywood and the Police: A Deep, Complicated and Now Strained Relationship, Hollywood Reporter
Workplace: be a better ally
- Ibram Kendi, One of the Nation’s Leading Scholars of Racism, Says Education and Love are Not the Answer, the Undefeated
- Analysis | The Technology 202: Black tech leaders share reading recommendations for the industry, The Washington Post
- Instagram Post, How Am Addressing White supremacy?
- Facebook Post, Leadership Listens
Workplace: better yet, own the problem yourself
- Tech companies are asking their black employee groups to fix Silicon Valley’s race problem — often for free, The Washington Post
- Message to White Allies from a Black Anti-Racism Expert: You’re Doing it Wrong, Progressively Speaking
- There Is No Such Thing as a ‘White Ally’, Human Parts
- Who Told White People To Check In On Me?, Najaa Young
- Facebook Post, Doing the Work
- Finding Opportunities to Apply Behavioral Science for Good in the Private Sector, Behavioral Scientist
- Facebook Post, 20 Things You Can Do Right Now as an Ally
- Twitter Post, Be Active, Not Performative
Workplace: more and better diversity
- ‘I’ve been stared at in disbelief when I introduce myself’, BBC
- Twitter Account, Sherrell Dorsey, Black tech news platform founder
- How Entrepreneurs Can Address Unconscious Bias, Entrepreneur
Workplace: representation in tech groups
Workplace: representation, advocacy, and training groups
Tech organizations improving the system
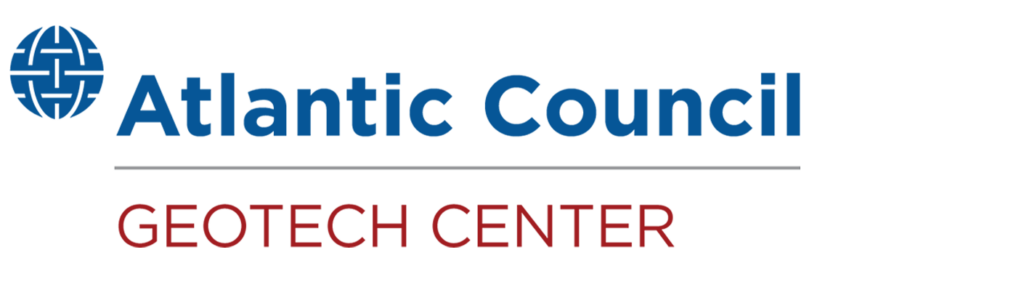
The GeoTech Center champions positive paths forward that societies can pursue to ensure new technologies and data empower people, prosperity, and peace.