#GoodTechChoices: Addressing unjust uses of data against marginalized communities
The Atlantic Council GeoTech Center’s #GoodTechChoices Series seeks to provide public and private sector leaders insight into how technology and data can be used as tools for good. In this analysis, the GeoTech Center examines two case studies in which data was weaponized against communities of color and recommends that data be re-envisioned by elevating marginalized voices and creating data review institutions.
Data can be a tool for good, but it can also be used for irreparable harm. For a data-driven and prosperous society, public and private sector leaders must:
- Account for the fact that they cannot use data in truly objective and unbiased ways;
- Identify how data has been collected, aggregated, analyzed, and applied throughout history by leaders in the public, private, nonprofit, and academic sectors to create systems of oppression;
- Create institutional and innovative mechanisms for data usage that elevate underrepresented voices and bring to light the modern-day context of data usage; and
- Address unjust uses of data to including addressing past and current injustices associated with data use and marginalized communities.
Introduction
Data sits at the center of power, from guiding the decisions of policymakers to serving as the lifeblood of the wealthiest companies. Today, data and its analysis are taking an increasingly prominent role. Steven Pinker, professor of psychology at Harvard, illustrates the vision best:
“Numbers, after all, aggregate the good and the bad, the things that happen and the things that don’t. A quantitative mind-set, despite its nerdy aura, is not just a smarter way to understand the world but the morally enlightened one.”
Data by itself is actionless and static. However, the interpretation and use of data by people, particularly for matters related to governance and management, can pose exceptional danger to society. Data-driven approaches to problem-solving and policymaking, which suggests altruism and competence, have also been used to oppress and persecute billions of people. While data and its analysis certainly support acts that are “good,” data can also be used maliciously and immorally to harm people and their communities. For example, consider how data can be weaponized in various applications of artificial intelligence. One need only take a cursory glance at the latest articles about the Chinese Communist Party’s vision of a social credit system that blacklists dissidents to see how the use of data can cause harm.
In this analysis, we explore two cases detailing how data has been weaponized in history for systematic oppression, leaving behind legacies that continue to harm communities of color. The first study discusses the way data was collected and used in the United States after the Civil War to criminalize Black communities. The second study assesses the use of aggregated data inside passbooks in apartheid South Africa and the way data was weaponized to restrict the geographic mobility of Black people. These examples illustrate how historical uses and abuses of data still influence society and must be considered when thinking about the use of data today.
Importantly, we do not discuss many communities in the United States and South Africa that have also been oppressed, including Indigenous, Brown, Latinx, Coloured (a large South African multiracial ethnic group), LGBTQ, and other communities. Both case studies offer a starting point for a conversation about the weaponization of data. This analysis concludes with recommendations for repairing the harm inflicted through the weaponization of data by elevating marginalized voices, creating Data Review Institutions, and reimagining the purpose of data.
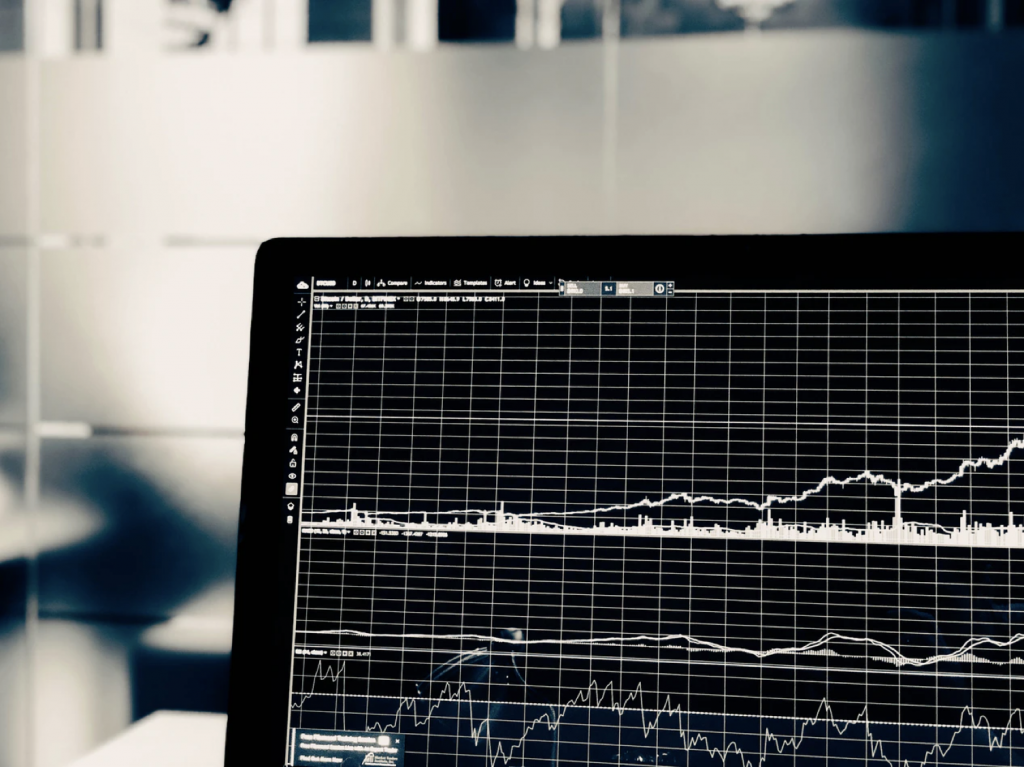
Humans weaponize data
It is critical to distinguish the human and institutional applications of data from data’s existence as a descriptive tool. While “good” people can collect and use data for innocuous or benevolent reasons, prejudiced people and oppressive institutions can do the same maliciously. Datasets collected in these circumstances are incomplete, biased, or misleading in the way they represent the world. Data is weaponized whenever it is used to inflict harm, well-intentioned or not.
As such, users of data must take responsibility for its effects. Data in and of itself cannot be blamed for violence inflicted against people. People determine how and when data is collected, cleaned, manipulated, contextualized, and translated into programs and decisions. If people collect data through oppressive practices or manipulate data in misleading ways, they create flawed datasets that either do not accurately represent the world or fail to convey crucial context about the information they contain. As a result, the de-weaponization of data requires changing institutions in power and human behavior itself, as well as the way data is collected and used—whether that be by adding additional data points to better reflect the world or choosing not to use a dataset at all. Data should be used for the service and empowerment of all, especially the most marginalized. The following two case studies should be viewed in this context.
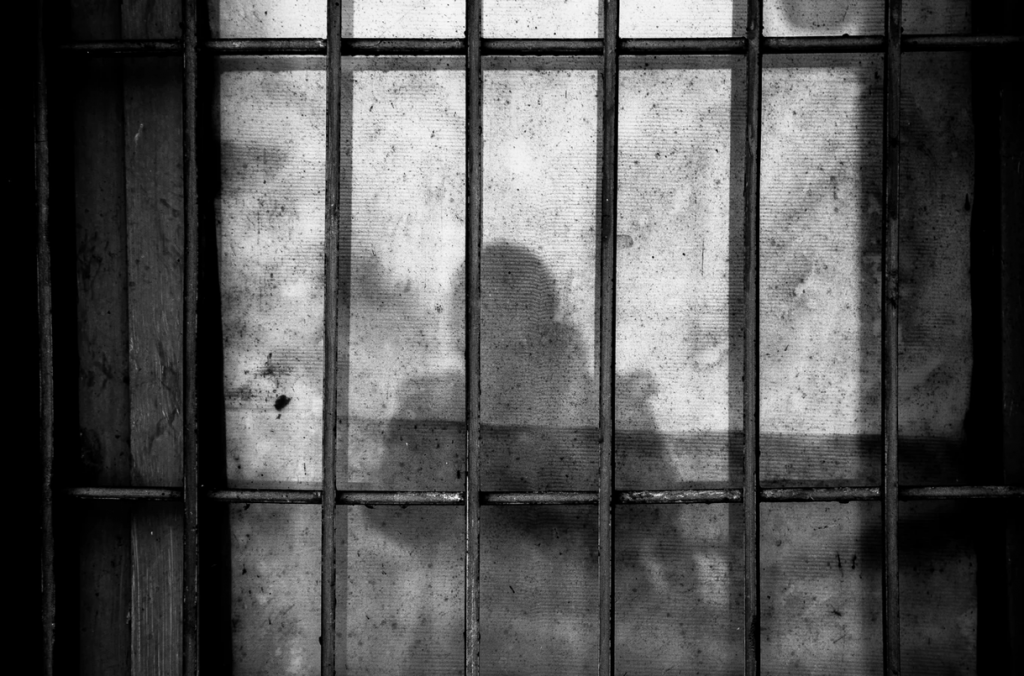
Post-Emancipation America and the racial data revolution
Emancipation and the defeat of the Confederacy in 1865 threatened to upend the social order of the United States. At the center of the upheaval was doubt: could newly emancipated Black people carry forward American prosperity? Many believed that Black people were incapable of navigating American society—either due to their perceived innate inferiority or a lack of education and wealth stemming from their enslavement. In The Condemnation of Blackness, Khalil Gibran Muhammad details how, following the United States Civil War, a new era of “data-driven” researchers studied what was considered the “Negro Problem” and “hoped that post emancipation demographic reports, with their tallies of births, deaths, morbidity, and prisoners, would prove to be key indicators of black fitness or lack thereof.”
Muhammad identifies actuary Frederick Hoffman as a key figure in the racial data revolution. In 1896, Frederick Hoffman published Race Traits and Tendencies of the American Negro, which quickly became a seminal work of the time. Compiling crime statistics, Hoffman weaponized data to argue that the higher rates of arrest and incarceration of Black people proved a naturally violent tendency. Instead of accounting for the underlying circumstances of Black life reflected in the data and the way it was collected—a history of enslavement, continued racism in policing and laws, excluding white crimes (e.g. lynching), and the constant systematic theft of Black people’s resources—Hoffman began his assessment of crime data with the a priori belief of Black inferiority. He intentionally aggregated flawed data to paint Black people as inferior and crafted a neatly packaged story that connected crime data with racial inferiority.
Hoffman’s assessment of crime statistics gained traction at the expense of other more qualified writers—those who offered the truth many white researchers were unwilling to acknowledge. The first to write a book on Black crime and an actuary for the Prudential Life Insurance Company, Hoffman marketed himself as a race-relations expert, but also as a German foreigner unbiased by the American history of slavery. Other researchers turned to and built on Hoffman’s work in pairing crime statistics with racial hierarchies. University of Chicago anthropologist Frederick Starr, for example, wrote in his review of Hoffman’s work, “The desire to turn black boys into inefficient white men should cease.”
In contrast, Ida B. Wells was the first Black scholar of the time to defend Black communities from charges of innate criminality. An antilynching activist and investigative journalist, Wells compiled statistics to challenge the prevailing belief that lynching was the result of rape committed by Black men. She published her findings in 1894 (just two years before Hoffman), which demonstrated that lynching was not a response to Black crime, but a tool to oppress Black communities.
Harvard trained sociologist W. E. B. Du Bois, another prominent Black scholar of the time, immediately called Hoffman’s work an “absurd conclusion” with an “unscientific use of the statistical method.” Du Bois saw Black crime as a symptom of a history of enslavement and in 1899 published The Philadelphia Negro, a comprehensive sociological study of the conditions of Black life in America. Despite the substantive work of Wells, Du Bois, and other Black scholars, researchers and foundations largely ignored their findings and focused primarily on Hoffman’s publications and the work of other white “race experts.” Their disregard illustrates how, while data in the same time period could be used to paint opposing narratives, the final decisions about its use were made by those in power.
Hoffman’s Race Traits had profound implications on American policy for the next century. By coupling crime statistics with race, Hoffman fostered new white supremacist research promoting the view that Black people were naturally violent and needed to be dealt with harshly. The research was used to justify the convict lease system, Jim Crow laws, and, more recently, brutal policing methods as part of the war on drugs. The result today is a criminal justice system that targets and oppresses communities of color, creating new, flawed data that ripe for further weaponization.
The history of crime data in the United States, beginning with Hoffman, showcases the damage inflicted upon Black communities. The interpretation of data fuels and is fueled by narratives of Black inferiority rooted in America’s violent and racist past. The United States continues to operate with this view, and, as a result, certain technologies today, including surveillance and risk assessment tools, are being built on racist interpretations of datasets collected by discriminatory practices to create algorithms that Princeton sociologist Ruha Benjamin calls the New Jim Code.
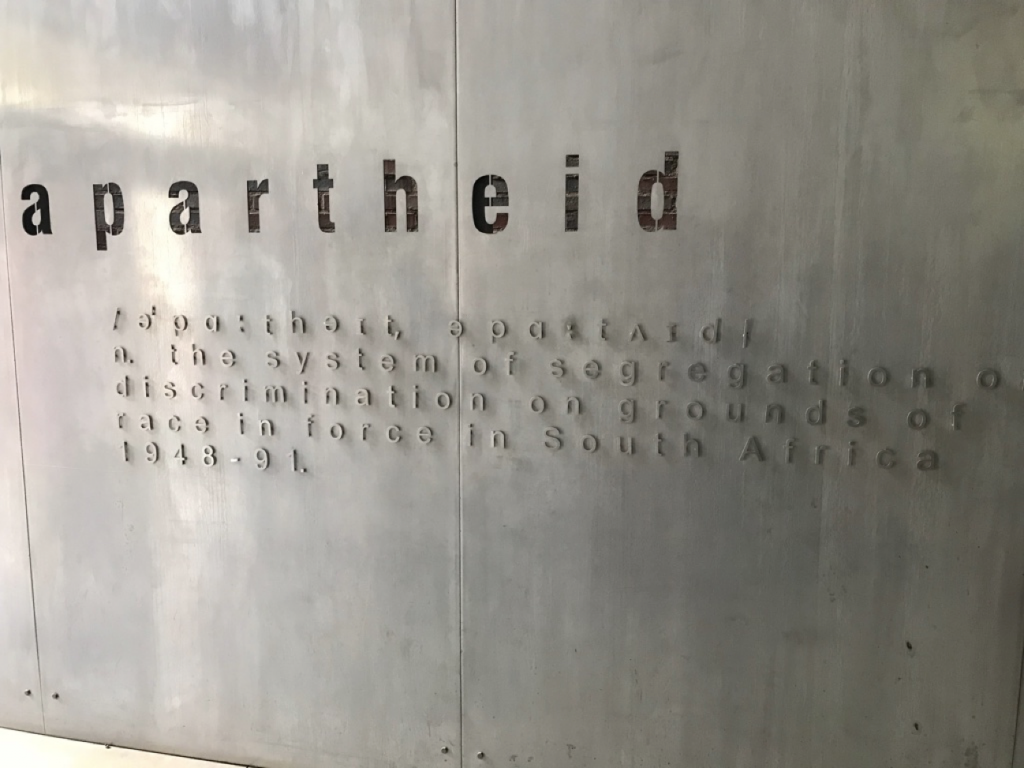
Apartheid and passbooks in South Africa
In South Africa, the gathering and use of data on a specific race was also used to establish a system of inequality. What is striking in both examples is how seemingly innocuous data was leveraged to inflict harm.
Most people are familiar with the brutal system of apartheid formally established in 1948 by the South African government. Discrimination based on race, however, was nothing new to South Africa. In fact, its roots lie in the colonization of the region by the Dutch over a century prior, when a white minority sought to establish power over a native Black majority.
A key enabler of this system of discrimination was the ability to track and control the movement of people within the borders of South Africa. Various South African laws, notably those passed and amended in 1923, 1945, and, of greatest consequence, in 1952 with the passage of the “Natives Act,” created the framework for this discrimination. The tactical purpose of these laws was to control the flow of Black labor in and out of predominantly white urban centers while, at the same time, providing a security construct for the white minority in power.
These laws called for and enabled the use of passbooks that contained key demographic information, including a photograph, fingerprints, place of birth, place of employment, employer remarks on work performance, and, most importantly, permission to be in certain geographic areas of South Africa. The data succinctly described the passbook’s holder and allowed the government to monitor and track the holder’s movements, as the passbook had to be carried at all times and presented to government officials and employers upon request. Lacking the correct approvals to be in a certain area or simply forgetting or losing one’s passbook could result in arrest and expulsion from the area where one worked. The purpose of having aggregated data in a passbook was to discriminate and promote a race-based caste system. The use of passbooks kept Black people largely relegated to rural and impoverished areas of South Africa, while white South Africans remained in the bustling urban centers.
The impact of passlaws and the passbooks they created still linger, crippling Nelson Mandela’s vision of a united South Africa. At the end of apartheid, white South Africans maintained control of the majority of land and assets in the country, while the Black populace remained in poor townships outside larger metro areas, rarely owning the land on which they lived. The geographic and economic differences between white and Black South Africans remain today. Additionally, while the South African government guarantees every citizen a home, these facilities have been developed in the outskirts of cities because homes and land in urban centers are already owned by white people. Furthermore, myths of a white genocide spread by right wing groups demonstrate how the underlying sentiments that created passbooks persist—the fear of Black South Africans taking land from white South Africans.
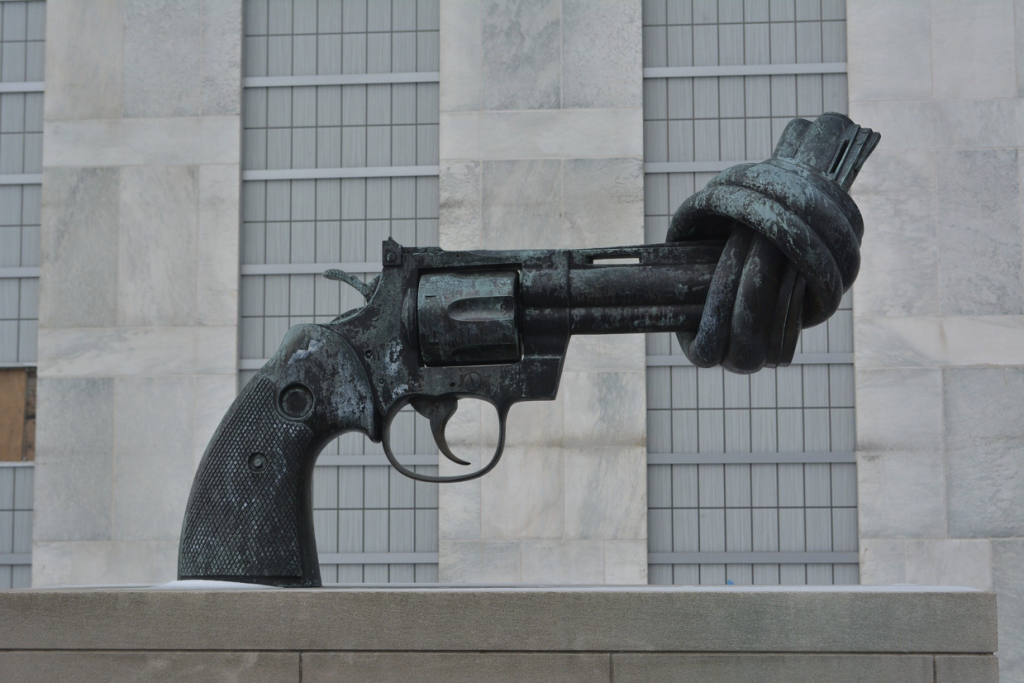
Creating institutional frameworks to address the weaponization of data
Weaponized data, as seen in the histories of the United States and South Africa history, continue to harm marginalized communities today. To de-weaponize data, new frameworks and systems are needed that target and change the way data is collected, aggregated, interpreted, and used.
Elevating voices
The communities harmed by the weaponization of data in the United States and South Africa then and now are, by and large, not part of the contemporary conversation about righting past wrongs. As a result, data cannot be fully leveraged for positive ends. The voices of marginalized communities are excluded by the same institutions and leaders who claim objectivity in their use of data, without understanding its effects “on the ground.” At best, these decisions are ignorant and well-intentioned, but, like the use of passbooks in South Africa, many also intentionally inflict harm, as argued by civil rights lawyer and writer Michelle Alexander in TheNew Jim Crow about the US war on drugs.
The weaponization of data is inextricably linked to the data user. Those developing and researching new technologies should “listen to, amplify, cite, and collaborate” with the stakeholders who have faced direct harm, writes Stanford AI researcher Pratyusha Kalluri. Data cannot be interpreted and used without partnering with and empowering organizations like Data for Black Lives and others actively seeking out and elevating underrepresented voices. Data causes harm when used by decision-makers who either fail to understand its flaws and implications or are motivated to use it maliciously. To de-weaponize data, those making decisions that have caused harm must be replaced with people from the communities that have been harmed.
A Data Review Institution that issues guidelines on the harmful implications of data
An independent governing body that reports on the potential limitations and harms of certain datasets is needed. The Data Review Institution would issue guidelines for the gathering and aggregation of data by conducting its own internal research, collecting and consolidating the research of other activists, and accounting for the historical context of the data and its use, similar to ProPublica’s analysis of the the COMPAS recidivism algorithm. Any person or organization using datasets in the normal course of their business would use recommendations provided by the Data Review Institution that detail the specific risks of the dataset. The Data Review Institution could sit within an existing agency like the Government Accountability Office or operate as a separate entity entirely, like the International Corporation for Assigned Names and Numbers (ICANN). Through its efforts, the Data Review Institution would provide recommendations and guidance to developers and researchers and potentially even call for the dismantling or protection of certain datasets. Policymakers may eventually decide to give the Data Review Institution greater regulatory power to enforce its guidelines and recommendations.
To better understand whether and how data is weaponized, citizen “data juries” created under the auspices of the Data Review Institution could serve as neutral arbiters for data-related disputes. In this system, people could bring forward evidence demonstrating the damages of data-based decisions made by government entities and private sector organizations. The concept is not entirely novel, as a proposal on a sentencing risk assessment tool in Pennsylvania was passed after three years of public hearings. Based on the evidence presented, citizen data juries would make conclusions on the impact and harms of the use of certain datasets and provide recommendations for their use.
The decisions of data juries would be noted by the Data Review Institution in their final list of data guidelines. The purpose of these guidelines would be two-fold. At the very least, they would better inform developers, researchers, policymakers, and the public about the potential harms of certain datasets if used incorrectly. At their best, the guidelines would provide the ammunition for policy proposals, legislation, and social movements around cases in which data has been weaponized against communities.
Re-envisioning better uses of data to break systems of oppression
Data must be re-envisioned as a tool for inclusive prosperity that empowers the most marginalized. Data can reflect prejudices in its collection or existence, or it can be accurate and holistic. In both scenarios, however, data can be interpreted and used for decisions that inflict harm. Datasets derived from past violence, for example, should be repurposed and used for prosperity by illustrating the harm inflicted or by demonstrating the need for more comprehensive data collection.
For this to happen, those using data must examine its purpose, context, and negative externalities. Part of this process will be connecting the data’s past uses to present injustices and predicting its future negative effects in the public and private realms. Algorithmic Justice League, for example, is carrying out this work by researching the impacts of AI and equipping advocates with empirical research. Similarly, people and organizations will need to actively seek out ways to reduce and address harms inflicted on marginalized communities, despite the potential negative impact on profitability or lifestyles of more prosperous communities.
The de-weaponization of data, in the end, is achieved by changing how people use data. Flawed datasets can be used for good, just as perfect datasets can be used to oppress. Data for good requires reimagining data’s purpose with an inclusive vision to address past injustices and empower those who have been and continue to be marginalized. In some cases, this might even mean turning away from data and technologies that cannot be repurposed for uses beyond creating and supporting systems of oppression.
Conclusion
People weaponize data by pairing it with their prejudices or shortsightedness to make decisions that oppress and harm. The racial data revolution in the United States and passbooks in apartheid South Africa are clear examples of the weaponization of data against communities—in these cases, communities of color. Decision-makers must be mindful, purposeful, and aware of the historical injustices inflicted through weaponized data. Otherwise, they risk turning to data and making similarly harmful decisions.
Data can be de-weaponized by accounting for the current and past injustices inflicted upon oppressed communities. For this, public and private sector leaders must:
- Elevate, partner with, and empower marginalized communities to be part of and lead decision-making for public policy, technology development, and business;
- Actively step away from decision-making positions to make space for underrepresented voices;
- Design independent Data Review Institutions and data juries that issue guidelines for the collection, aggregation and use of datasets;
- Identify the negative effects of data-based decisions on marginalized communities and actively choose to not inflict harm despite the positive effects on revenue or social good for other communities; and
- Use data to make decisions that improve communities rather than ignore them or inflict further harm on them.
In an increasingly digital world, data and its analysis guide almost every decision today. That alone, however, does not lead to a better society. Achieving one requires a more just and equitable people.
The Atlantic Council GeoTech Center would like to thank Nikhil Raghuveera and Tom Koch for serving as lead authors for this report. Nikhil is a Nonresident Fellow at the Atlantic Council GeoTech Center. Tom is a Marshall Memorial Fellow at the German Marshall Fund of the United States.
We encourage you to discuss and share this post with colleagues, friends, and family members as stopping unjust uses of data and tech requires us all to take action together.
Further Reading
- “Pinker, Steven, “Harvard Professor Steven Pinker on Why We Refuse to See the Bright Side, Even Though We Should,” Time, January 4, 2018.
- Kobie, Nicole, “The Complicated Truth About China’s Social Credit System,” Wired, June 7, 2019.
- Muhammad, Khalil Gibran, The Condemnation of Blackness: Race, Crime, and the Making of Modern Urban America, Harvard University Press, 2010.
- Hoffman, Frederick, Race Traits and Tendencies of the American Negro, Franklin Classics, 2018.
- “Black Code,” Ferris State University
- Anderson, Carol, White Rage: The Unspoken Truth of Our Racial Divide, Bloomsbury USA, 2017.
- Wells, Ida Bell, A Red Record: Tabulated Statistics and Alleged Causes of Lynchings in the United States, 1892-1893-1894, Donohue & Henneberry, 1895.
- Du Bois, W. E. B., et al. The Philadelphia Negro: A Social Study. University of Pennsylvania Press, 1996.
- Hill, Kashmir, “Wrongfully Accused by an Algorithm,” New York Times, June 24, 2020.
- Larson, Jeff et al., “How We Analyzed the COMPAS Recidivism Algorithm,” ProPublica, May 23, 2016.
- Kearse, Stephen, “The Ghost in the Machine,” The Nation, June 15, 2020.
- Benjamin, Ruha, Race After Technology: Abolitionist Tools for the New Jim Code, Polity, 2019.
- “Pass laws in South Africa 1800-1994,” South African History Online.
- “Apartheid Pass Book,” BBC.
- Malala, Justice, “Why Are South African Cities Still So Segregated 25 Years After Apartheid?,” The Guardian, October 21, 2019.
- Chothia, Farouk, “South Africa: The Groups Playing on the Fears of a ‘White Genocide’,” BBC, September 1, 2018.
- Alexander, Michelle, The New Jim Crow: Mass Incarceration in the Age of Colorblindness. New York: The New Press, 2010.
- Kalluri, Pratyusha, “Don’t Ask if Artificial Intelligence is Good or Fair, Ask How it Shifts Power,” Nature, July 7, 2020.
- “COMPAS Recidivism Risk Score Data and Analysis,” ProPublica Data Store, July 2020.
- “Pennsylvania Commission on Sentencing’s Risk Assessment Tool: What Happened,” ACLU Pennsylvania, September 16, 2019.
- “Report on Algorithmic Risk Assessment Tools in the U.S. Criminal Justice System,” Partnership on AI.
- Larson, Jeff et al., “How We Analyzed the COMPAS Recidivism Algorithm,” ProPublica, May 23, 2016.
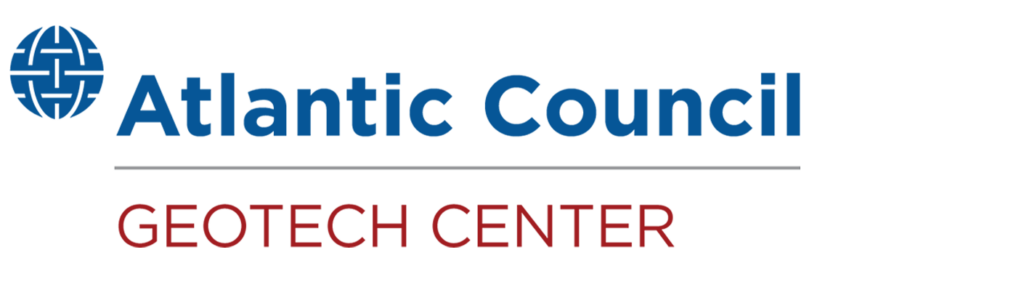
The GeoTech Center champions positive paths forward that societies can pursue to ensure new technologies and data empower people, prosperity, and peace.