Detecting disruption in closed systems
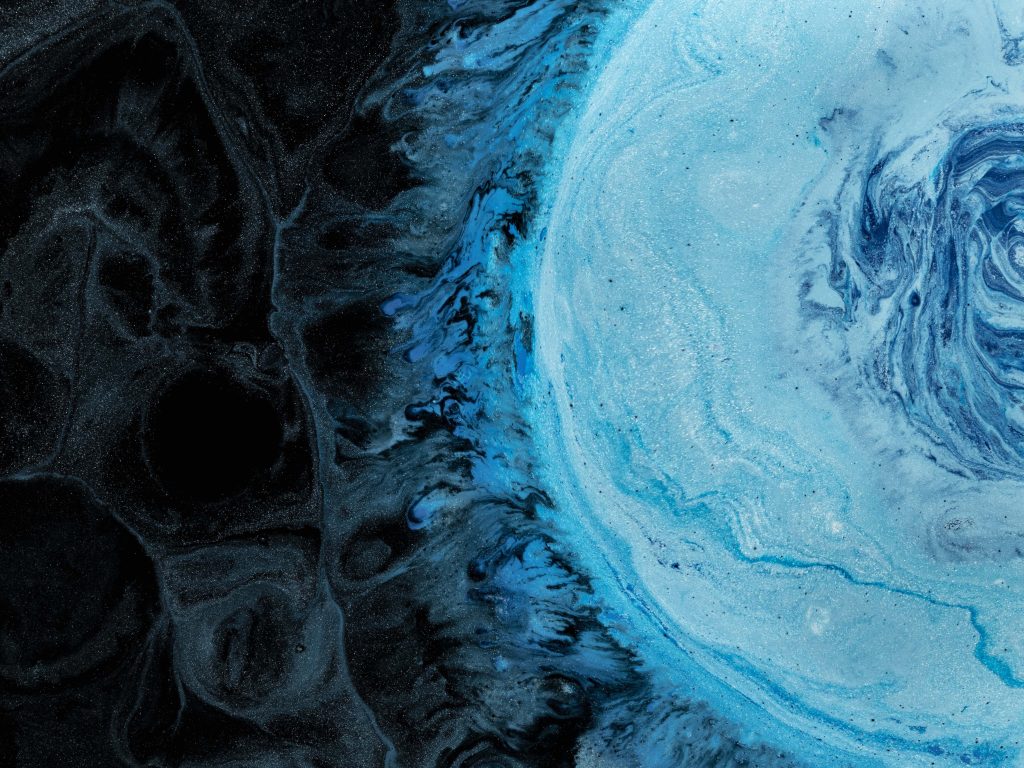
Editor’s preface and acknowledgments
Executive summary
Study context and goals
Study assumptions and context
- Extracting a sample of bases
- Selecting a set of known perturbing events
- Employing identity resolution and heuristic evaluation
- Selecting a physical centroid
- Establishing connectivity in time and through time
- Measuring perturbation
- Methodological validation
- Review of statistical methodology
- Implications with regard to the elasticity of decisions
- Potential limitations and remedies
- Regarding changes in observable data and human behaviors
- Regarding open-source data implications
Possible next steps based on this study
Summary and closing implications
About the authors
Acknowledgments
Editor’s preface and acknowledgments
The microscope and telescope unlocked new dimensions for discovery and enabled humanity to explore hidden ecosystems, formulate new solutions to problems, and advance societies. Analysis of data, particularly when enabled by today’s computational techniques and advanced computers, similarly reveals hidden dimensions of our world and provides novel insight into complex patterns such as the interactions among people, infrastructure, and social systems. Such discoveries, when harnessed by policymakers, have the potential to positively impact our world.
This report provides an example of such groundbreaking insight derived from analysis of Dun & Bradstreet’s commercial data set in combination with research and analysis provided by the Atlantic Council GeoTech Center and Johns Hopkins University Applied Physics Laboratory.
The Atlantic Council GeoTech Center’s mission is to shape the future of technology and data together to advance people, planet, prosperity, and peace. The GeoTech Center serves as an essential bridge between technologists and policymakers, bringing them together to consider the broader societal, economic, and geopolitical implications of new and emerging technologies; leverage technology to solve global challenges; and develop actionable technology policy, partnerships, and programs.
Detecting Disruption in Closed Systems is a unique endeavor that fulfills every facet of this mission.
The data collaboration was made possible by Dun & Bradstreet, which played an essential role by providing the following:
- Access to its commercial data set and Analytics Studio, a cloud-based platform. The Analytics Studio software was made available to a project team comprising GeoTech Center staff and fellows as well as a team of researchers from the Johns Hopkins University Applied Physics Laboratory team.
- The expertise and staff time of a Dun & Bradstreet team of data scientists, who conducted the data analysis and contributed to the writing of the report, primarily to ensure that the description of the methodological approach is accurate.
- A gift to support the work of the GeoTech Center in producing this report.
Anthony Scriffignano, PhD, facilitated the partnership between Dun & Bradstreet and the GeoTech Center. He serves on the Commission on the Geopolitical Impacts of New Technologies and Data, established by the Atlantic Council in 2021, and is a senior vice president and chief data scientist at Dun & Bradstreet Corp. He has contributed to data science practices at Dun & Bradstreet and is the primary inventor on many patents globally in the space of data and information processing. Due to the proprietary nature of the commercial data and the expertise needed to analyze it, the majority of the data was analyzed by a Dun & Bradstreet team reporting to him.
He also contributed meaningfully to the writing of this report, particularly to the statistical background, study methodology, and results sections. The methodology of this report and all the analyses conducted by Scriffignano and his team were closely examined and deemed sufficiently rigorous by third-party experts, primarily from the John Hopkins University Applied Physics Laboratory. A full list of contributors and their roles is available in the acknowledgments section of this report.
The Atlantic Council GeoTech Center created a framework for data sharing by the private sector that allows for vetted data analysis to be provided to policymakers with the goal of benefiting citizens and providing increased situational awareness for closed communities such as military bases or other closed ecosystems of high value and importance. The ground rules for data sharing and methodological validation put in place by the GeoTech Center ensured that the methodology, analysis, and conclusions are sound, accurate, and rigorous. In keeping with our intellectual independence, the findings, and guidance included in this report are independent of the views of our partner.
The responsible use of these commercial data sets can contribute significantly to the protection of democracy, national security, and society. This collaboration on the part of Dun & Bradstreet represents a pioneering effort by a private-sector company to make data and analytical capabilities more accessible to policymakers for the public good. We believe that such private, civil, and public-sector data collaborations are essential to securing our future and hope other organizations with join us in making data and meaningful analysis more accessible.
The Atlantic Council GeoTech Center is extremely proud to present this body of work.
Stephanie Wander
Director of Programs
Lloyd Whitman, PhD
Senior Director
Executive summary
Disruption is a natural part of life. It can appear in many forms including natural disasters, economic crashes, and social conflicts. The world’s resiliency to disruptions, particularly natural disasters has greatly improved over the past few decades. This resiliency is, in large part, due to enhanced detection and warning capabilities enabled by extensive data sets and commercial analytics.
In order to improve resiliency to disruptions, one must understand the strengths and weaknesses of an individual community. This understanding is more complicated for isolated and closed communities. However, recent developments in commercial data aggregation and creative data analytics have allowed data experts to overcome this challenge. This paper utilizes commercial data aggregation and data analytics to evaluate whether commercial data sets can be used to better understand the resiliency of closed military communities or other closed ecosystems of high value and importance.
In this report, the authors demonstrate that it is possible to analyze commercial data sets to understand resiliency by detecting disruptions (also referred to as perturbations to account for the possibility that changes in interaction can occur without external influence) around military bases or other important geographically localized institutions both isolated in time (e.g., hurricanes) and enduring through time (e.g., pandemic restrictions). The study reveals indications and warnings can be derived from commercial data sets and the observable imprint all organizations leave in commercial data sources.
As such, this study’s methodology may be employed, expanded, and refined to analyze other closed communities. It is anticipated that any attempts to camouflage or hide such changes will themselves leave new anomalies that this analytic means can detect.
Disruptions serve to tip off leaders about upcoming or ongoing challenges. They empower leaders to reduce community vulnerabilities to outbreaks, food scarcity, and regional instability. As the world becomes increasingly digitized, humanity has an opportunity to better understand the impacts of human activities on the globe as well as nature’s impacts on society.
In sum, there are five major takeaways from this report for leaders in both the public and private sectors:
- The increasing digitization of the planet enables subtle tips and cues to be derived from commercial data, as described in the report.
- Those seeking to keep their activities confidential need to be aware of the “digital exhaust” they may be producing associated with adjacent activities within the broader ecosystem in which they operate.
- It is anticipated that any attempts to camouflage or hide such perturbations will themselves leave irregularities that analytic means can detect.
- Those seeking to better understand the planet should consider how commercial data sets can provide indicators and warnings associated with phenomena such as famine.
- As organizations and societies become increasingly digitized, people will be able to understand the impact of human activities on the world, as well as nature’s disruptions on human societies, better than ever before.
Subsequent sections of this report convey the analytic methodology, results, discussion of possible limitations, potential extensions and next steps, and closing implications.
Study context and goals
A natural part of life, disruptions can appear in many forms, particularly at the intersections of natural, climate, and human ecosystems. By understanding what perturbations in commercial data patterns reveal about potential disruptions in far more closed systems of activities and coordination, one can better understand the phenomenological interactions in such ecosystems.
The research conducted for this project had both scientific and ethical goals. The primary scientific goal was to study the impact of acute disruptions on chosen military community ecosystems by observing perturbations in the patterns of the businesses in the commercial ecosystem surrounding the base. This was done by using commercial data provided by Dun & Bradstreet.
Other scientific goals included: determining if commercial data can partially evaluate the resiliency of the associated ecosystem surrounding a military base (or other closed ecosystems of high value and importance) in the face of disruptions, and determining if any of the chosen military communities have clusters of influential entities that can be held responsible for shaping how these communities react to short, isolated disruptions in time and long disruptions through time.
This research also sought to inform defense officials and community leaders how commercial data can be used by any party, friendly or adversarial, to gauge a community’s resiliency to disruptions and to indicate weak spots in their community needing improvement, particularly regarding disruptions that repeat and potentially worsen, such as those caused by climate change.
Study assumptions and context
The authors of this report conducted this study to measure the impact of acute disruptions on selected military community ecosystems by observing perturbations in the commercial activities of the businesses in military base ecosystems that are discoverable in commercial data provided by Dun & Bradstreet.
The analytic method used to measure perturbation in this study, an application of geospatial inference methods and Jensen-Shannon divergence,1The Jensen-Shannon divergence is named after Jensen’s inequality and the Shannon entropy from information theory. The formulation of the divergence appears to have been introduced in the academic literature by Wong and You in 1985, but it is closely related to the Jensen-Shannon distance introduced by Rao the same year. works best for military bases or other geographically localized institutions that are large enough to anchor commercial ecosystems. This method also works best for localized institutions that are not near other large economic hubs, such as other military installations, as this raises the specter of multimodal effects.
The military installations selected are each separated spatially from large commercial or government presences, either by distance or de facto boundaries. In each case, the base therefore drives the local area’s economy. Specifically, the military base’s presence justifies the decision of most residents to live and interact commercially nearby.
This study can be applied internationally to military bases and other closed ecosystems, although this method should work best for countries that invest significantly in their military presence and advancement of their installations, along with countries that have significant levels of private-sector data relating to the surrounding ecosystem, and are open to information and data sharing without significantly limiting government control and surveillance.
Contextual assumptions
To construct an empirically rigorous methodology, this study progressively decomposed the conceptual foundation of the method and formally articulated its axioms and related epistemology. Specifically, this study centered on the following research question:
To what extent can we meaningfully observe disruption to a known location by observing perturbation in the commercial ecosystem that physically surrounds it?
There are certain presumptions which inform this question. These presumptions are based on the collective experience of a group of individuals who are familiar with the types of locations being studied and familiar with commercial ecosystems. For example, it is commonly known that some individuals who work in such locations travel to and from work. While doing so, they engage in commerce such as buying food, getting car repairs, possibly staying in local hotels, or other commercial transactions. The locus of operation itself also engages in commerce with the surrounding ecosystem in many ways such as hiring local practitioners (e.g., cleaning services, road works), community outreach, or consuming locally available goods and services.
Moreover, for the purposes of this study, certain aspects of the entities and environment were presumed to be true. It is certainly possible to construct other studies to test any of these stipulations, however they were held to be true for the purposes of this exercise. The table below describes them.
# Axiom |
A1 There is a set of potentially disrupted entities in ecosystems of interest (e.g., military installations or other closed ecosystems of high value and importance) which are identifiable in a way that is empirically rigorous and commonly accepted. |
A2 There exists sufficient, well-curated data regarding the entities in A1 that are stable and representative over time. |
A3 There exists sufficient longitudinal data to understand the impact in the entities in A1 surrounding well-understood prior disrupting events (e.g., major natural disasters and significant organizational changes). |
A4 Latency in the data will be controlled sufficiently with respect to the disrupting events studied. |
Of note, this study was not investigating near-term disruption where the system is changing faster than the data that describes it. Other methods exist for that class of problem, which are not part of this specific study’s effort.
Attributes posited in study
This study proposed additional attributes of the entities and ecosystem, and the implementation of the methodology on selected bases intended to clarify the stipulations’ veracity. They include:
Specific study methodology
This study began by extracting a sample of bases to study, taking into consideration geography. The major steps of the methodology are summarized in Figure 1.
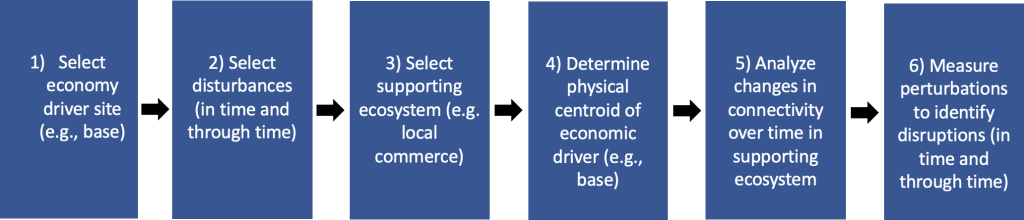
Figure 1. Flowchart of study methodology
Extracting a sample of bases
To initially assess the viability and efficacy of this study’s method, a series of bases were selected where it was known in advance there should be sufficiently robust data. The paucity of data in certain geographies represented a potential limit on the ability to execute a method such as that proposed. Accordingly, bases were chosen in North America for the initial round of testing, based on availability of sufficiently robust ecosystem data to demonstrate the methodology.
This presumption of study does not preclude the availability of sufficient data elsewhere. Rather, it represents a reasonable first step with controls for possible missing data or other constraints. The geographical restriction ensured reasonably consistent data, allowing assignable cause variation in the results to be accountable to aspects other than quality issues or other absences in the data.
Selecting a set of known perturbing events
For the purposes of studying perturbation in the selected bases, there was a desire to identify longitudinal data that would contain well-understood perturbation. The authors considered two types of perturbation. The first type was perturbations in time, which occur in a specific, known and reasonably short timeframe. Examples include natural disasters such as hurricanes, as well as specific events such as fires or other human-made events. The second type was perturbations through time, which occur over a longer time duration, exhibiting their impact in a more protracted presentation. Examples of perturbations through time include response to potential base closings, major organizational changes, and ongoing response to lack of availability of some critical dependency such as locally sourced materials.
To maximize the likelihood of detecting perturbation, initial efforts focused on events in time or through time that had sufficient scope and scale. Expert interviews informed the construction of a small list of well-understood and significantly disruptive events at specific bases. The disruptions were understood either because of direct involvement with the perturbation or because the impact of the event on the location was so significant that it was widely recognized. The majority of these were perturbations in time.
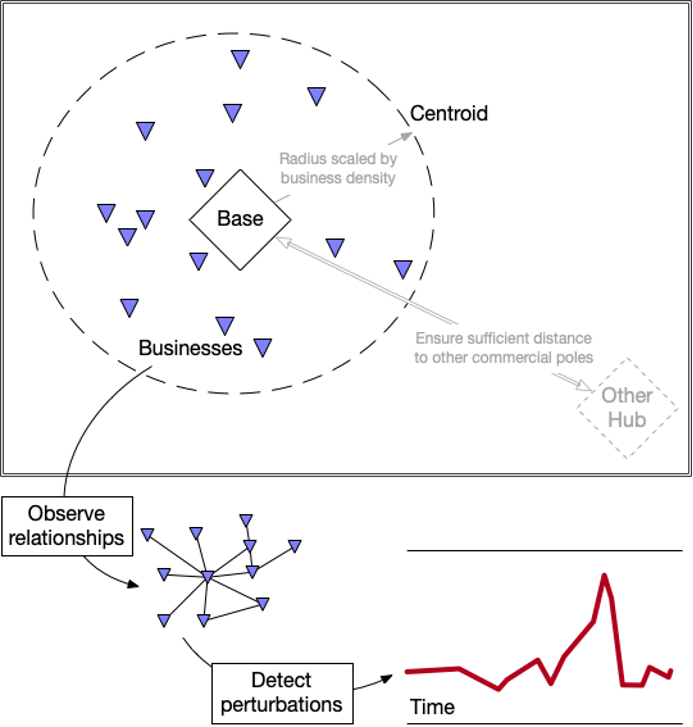
Employing identity resolution and heuristic evaluation
Each base in this study was identified in commercial data sets through a commercially available, bespoke identity resolution process, which entails comparing data points and deciding if they represent the same “entity.”2“Decoding Identity Resolution, Part One: The Basics,” Amperity (blog), May 25, 2022, https://amperity.com/blog/decoding-identity-resolution-part-one-the- basics. This process took into account the possibility of multiple entities that could correspond to the base, as well as treatments for geospatial complexity (e.g., intervening mountains, waterways). This process also included treatments for confounding aspects of identity resolution including multiple names for locations, postal standards, entities with similar sounding names, orthographic variation, and other characteristics of the data. Robust stewardship rules were employed to ensure consistency across the identity resolution process, as well as heuristic evaluation of results to ensure a recognizable foundation for the downstream evaluation.
Selecting a physical centroid
For the purposes of establishing a centroid around the base from which to draw the commercial ecosystem, multiple methods were considered. Overly simplified Euclidean methods3Benno Artmann, “Euclidean Geometry,” in Encyclopaedia Britannica, September 10, 2020, accessed July 23, 2022, https://www.britannica.com/science/ Euclidean-geometry. were rejected due to known bias that such approaches would introduce (e.g., sensitivity is elastic with respect to density of the area of interest). Highly sophisticated methods that consider the nonspherical nature of the Earth were also rejected because they introduced unnecessary complexity and did not improve the utility of the results for informing decisions.
The ultimate decision was to establish centroids based on haversine distance,4Akshay Upadhyay, “Haversine Formula: Calculate Geographic Distance on Earth,” IGIS Map, accessed July 23, 2022. and scaled according to the known business density in the centroidal region. Accordingly, highly dense areas produced small centroids while sparser areas produced larger centroids. Conservative scaling factors were used for the initial iterations of the method to reduce the size of the commercial ecosystem to facilitate the evaluation of the results by human raters.
A set of similarly instructed human raters were asked to evaluate the efficacy of the establishment of centroids. The raters responded that the centroids matched their expectations for commercial ecosystems. Since the method used is empirically rigorous, it is scalable to much larger sample sizes for future study.
Establishing connectivity in time and through time
Based on the established centroids, a small group of bases was used to create a time series of connected graphs, with nodes representing the entities in the ecosystem and edges related to dyadic relationships“5Dyadic Data Analysis,” Columbia University Mailman School of Public Health (website), accessed July 23, 2022, https://www.publichealth.columbia.edu/ research/population-health-methods/dyadic-data-analysis. of known commercial interaction. The construction of centroid regions and analysis of ecosystems is illustrated in Figure 2. The analytic period chosen for the establishment of the time series were sufficiently large to bracket the known perturbing event, with the intention of including some period of quiescence before the perturbation and return to some steady state after the perturbation.
The resulting time series were then analyzed according to common and bespoke measures of graph complexity and connectivity. These measures were chosen to reflect the characteristics of the graphs and the changes in behavior observed in the dyadic relationships. To facilitate future ingestion of these measures into a higher-order inference, all measures were converted to Z scale (which measures the position of a data point relative to the mean and in units of the standard deviation),6Stephanie Glen, Z-Score: Definition, Formula and Calculation, StatisticsHowTo.com: Elementary Statistics for the rest of us!, accessed July 23, 2022, https://www.statisticshowto.com/probability-and-statistics/z-score/. producing relative probability distributions.
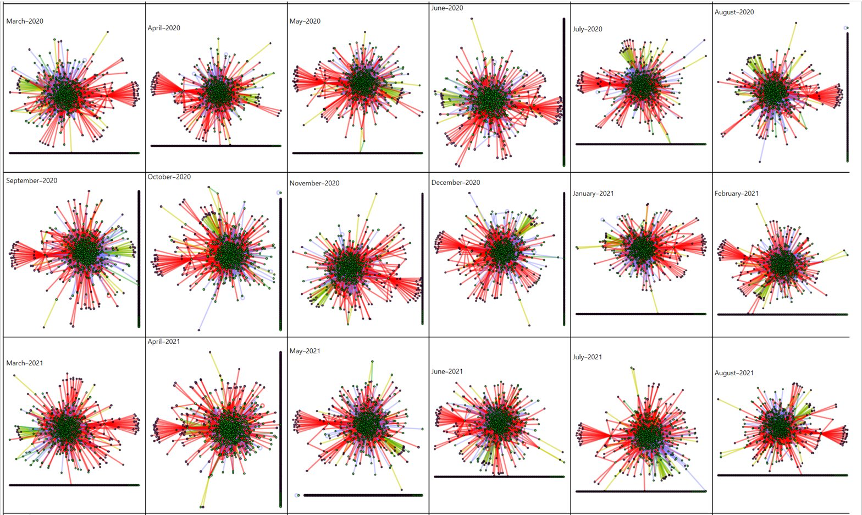
Figure 3. Example of time-series graph of the dyadic relationships before, during, and after a period of perturbation.
Potential absences in the data were carefully considered. Since not all commercial interactions are observable, the elasticity of decisions made based on these observed relationships could be problematic. Although the method observes changes in the ecosystem, regardless of absent data, it is important to consider any major changes in curation or creation of data that may have occurred during the analytic period, as these changes could present falsely as perturbing events. Such changes could result in artificial perception of perturbation when in fact what would be measured would be the effects of changes in the data brought about by curation and creation, not by behavior in the observed ecosystem.
Measuring perturbation
Multiple methods were considered for the overall measure of perturbation. Simple statistical measures were deemed insufficient because they had unusually high sensitivity to changes in one or more of the graph measures. Additionally, since the edge types are markedly heterogeneous, factor analysis or other scaling would be required, and would introduce additional sources of elasticity with respect to observing perturbation. Accordingly, Jensen-Shannon divergence was chosen. This method, comparing the statistical distributions of the various Z scale measures mentioned above, produces a very easily observable measure that can be compared to detect perturbation both at a point in time and over the course of time (“in time” and “through time”).
The Jensen-Shannon divergence was computed for the graph measures of the commercial ecosystems of the selected bases. Around each known disruption in time, there was a clear observable increase in divergence in the data. Other increases in perturbation that were unexpected were evaluated to determine if the method was overly sensitive or otherwise flawed, or if there were simply other disruptions that had not been considered.
Figure 3 is an example of the time-series graphs derived in this study. The graphs depict examples of perturbation as measured through the derived method and is visualized through dyadic relationships before, during, and after a disruption in the commercial ecosystem surrounding an economic driver (e.g., a military base or other closed ecosystems of high value and importance).
In this example, perturbation is measured with respect to individual and combined graph measures, allowing for a rich understanding of perturbation in the ecosystem being studied surrounding the economic driver.
It is possible that the method could be influenced by intentional manipulation of data. For example, if falsified and perturbed data were introduced, it could result in creating Jensen-Shannon divergence not related to actual commercial fluctuations in the ecosystem. Other types of intentional manipulation might include smoothing of data through manipulation to avoid the detection of otherwise existing perturbation. However, due to the complexity of the method and the number of attributes measured, as well as the various sources from which the data is drawn, it is highly unlikely that such intentional manipulation could be successfully achieved without producing its own perturbation, which would accordingly be measured by the process. This “observer effect” of the method to detect any attempts to change the output of the method is a feature worthy of additional study.
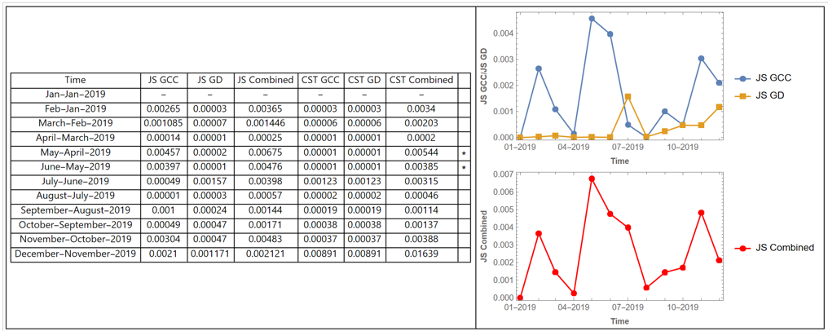
Figure 4. Demonstrates the depiction of perturbation as measured through the derived method.
It is possible that the method could be influenced by intentional manipulation of data. For example, if falsified and perturbed data were introduced, it could result in creating Jensen-Shannon divergence not related to actual commercial fluctuations in the ecosystem. Other types of intentional manipulation might include smoothing of data through manipulation to avoid the detection of otherwise existing perturbation. However, due to the complexity of the method and the number of attributes measured, as well as the various sources from which the data is drawn, it is highly unlikely that such intentional manipulation could be successfully achieved without producing its own perturbation, which would accordingly be measured by the process. This “observer effect” of the method to detect any attempts to change the output of the method is a feature worthy of additional study.
Methodological validation
For each base studied, current or former residents of the base were interviewed. These residents confirmed that the base was the primary local economic engine.
Once confirmed, businesses were plotted within the base’s centroid region on a map of the locale, and this map was presented to the current and former residents. They were then asked whether all businesses were connected tightly to the base’s economic activities, or if there were other large commercial or government presences nearby that would constitute economic poles.
Current and former residents responded either by confirming that the centroid region included only the base’s commercial ecosystem—as in the case of Coast Guard Base Kodiak and Joint Base Pearl Harbor-Hickam—or by suggesting a different gate of the base about which to construct the centroid region—as in the case of Marine Corps Base Camp Lejeune. Geographic regions other than centroids were considered, such as linear regions conforming to commercial corridors, but centroids were recognized ultimately to be the most consistently appropriate shape.
Review of statistical methodology
A Johns Hopkins University graph analytics expert who was not on the team associated with this study reviewed the statistical methodology of the study. The independent expert provided feedback on the method and implementation of constructing polyhedrons for each month’s dyadic commercial relations, computing global graph metrics on each month’s polyhedron, and comparing subsequent months’ graph metrics using the Jensen-Shannon divergence. The feedback was consonant with the study’s approach.
Results
Members of the research team identified disruptions temporally coincident with perturbations detected by the methodology described herein. Some of the perturbations occurred in the aftermath of large climate disruptions in time, such as back-to-back hurricanes landing on the eastern coast of the continental United States in early September 2019 and an earthquake in the northwestern United States in July 2021. Other disruptions coincident with perturbations were less easily discoverable in public reporting, such as finding a suspicious package on one of the bases and the onset and lifting of another base’s lockdown due to the COVID-19 pandemic. These and other disruptive events, both climate-related and less easily discovered, coincided with or closely preceded perturbations detected by the study method.
Actual results for two of the bases studied, Camp Lejeune and Wright-Patterson Air Base, demonstrate the data perturbation model as hypothesized. Perturbations were observed at both sites around the time that storms impacted the bases. Camp Lejeune was impacted by back-to-back hurricanes, Dorian and Erin, in the fall of 2019. Wright-Patterson Air Force Base was impacted by a tornado in May 2019.
These results are observed in the graph time series and in the data perturbation quantification below.
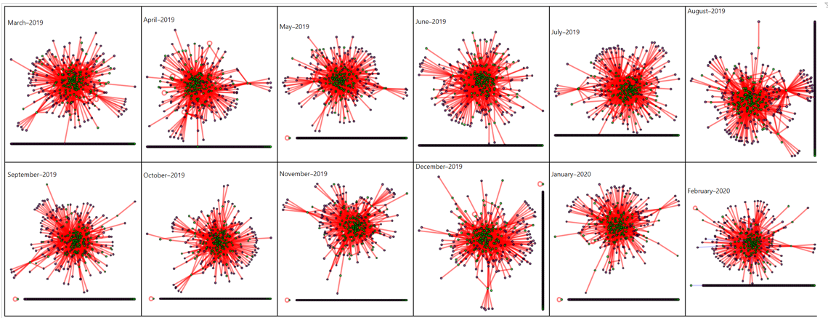
Figure 5. Camp Lejeune, Effect of Dorian and Erin Storms on Data Perturbation in September 2019.
The expected disturbances at Camp Lejeune, hurricanes in the fall of 2019, are observable in the perturbation qualification data. The Jensen-Shannon divergence identified anomalies between July and October of 2019 that coincide with Hurricanes Dorian and Erin.
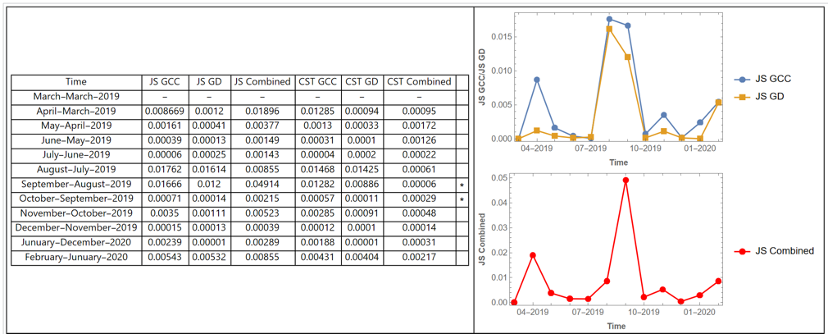
Figure 6. Camp Lejeune Data Perturbation Quantification, March 2019 to February 2020.
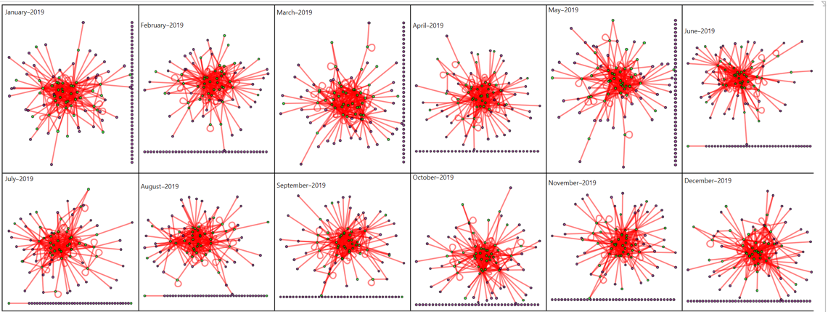
Figure 7. Wright Patterson Air Force Base, Effect of Tornado on Data Perturbation in May 2019.
The expected disturbance at Wright-Patterson Air Force Base, a tornado in May 2019, is observable in the perturbation qualification data. The Jensen-Shannon divergence identified anomalies between April and July of 2019 that coincide with the tornado.
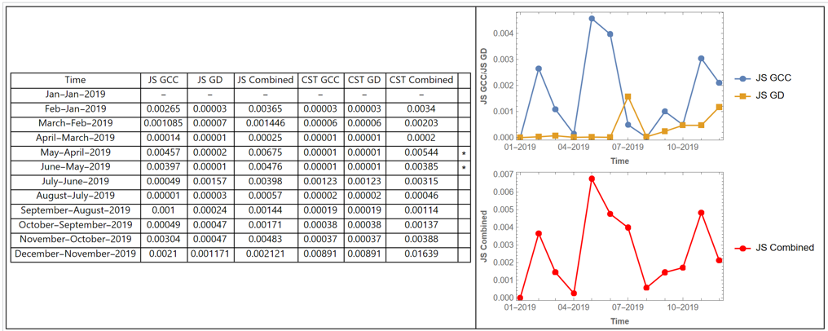
Figure 8. Wright Patterson Air Force Base, Perturbation Quantification, January 2019 to December 2019.
The perturbation quantification data from Pearl Harbor-Hickam is captured below. The peak in early 2020 is coincident with a suspicious item on the base. The peak in the summer of 2020 lines up with the preparation for a hurricane that came closer to Oahu than any in recent years.
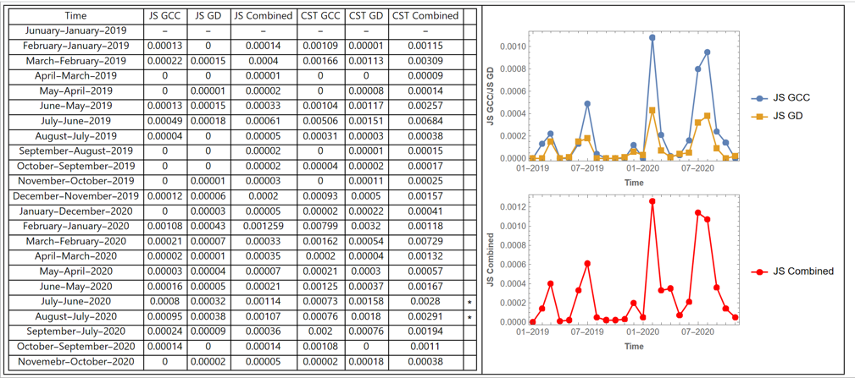
Figure 9. Pearl Harbor-Hickam, Perturbation Quantification, June 2019 to December 2020.
Below is the perturbation quantification from Base Kodiak. The largest and longest-duration period of a high level of perturbation occurred in the first three months of the base’s lockdown due to the COVID-19 pandemic. The next-highest perturbation is detected in the aftermath of an earthquake in July 2021.
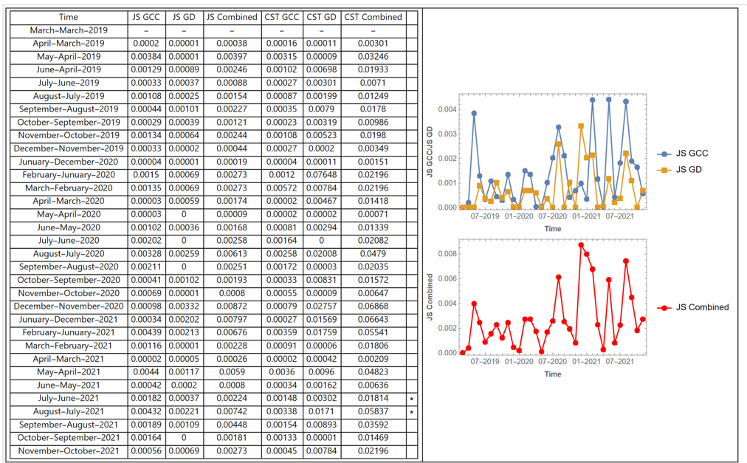
Figure 10. Kodiak, Perturbation Quantification, June 2019 to December 2021. Discussion of the Implications and Potential Limitations
This study considered the elasticity of decisions linked to the results shown. Regarding such elasticity of decisions, it is not necessary for the method to be a highly precise detector of perturbation. What is important is that perturbation is observable.
The findings in this study supported the scientific goal to determine if commercial data can partially evaluate the resilience of the associated ecosystem surrounding a military base or other closed ecosystems of high value and importance in the presence of disruptions. As observed in the prior section, the study determined this to be the case at multiple bases resulting from static disruptions in time and dynamic disruptions through time. It is recommended that future studies work to determine if any of the selected bases have clusters of influential entities responsible for shaping how these communities react to disruptions. Per the study intent, the results are provided to inform defense officials and community leaders of how commercial data can be used to gauge a community’s resilience to disruptions.
Implications with regard to the elasticity of decisions
It is important to evaluate the elasticity of decisions made with this study’s method. For example, if the method is used merely to direct the attention of human evaluators to focus in one area versus another, it is only important for the method to be able to separate one base from many to provide a relative scale of the likelihood of finding meaningful perturbation were human raters to intervene. In contrast, if more automated methods are used, a more precise measure would likely be needed to direct the activity of digital agents to engage in specific interventions. Such precision could be approached with additional bespoke graph measures, as well as careful consideration of curation of data as discussed above.
This study’s method should be extensible to any commercial ecosystem. The only stipulations are availability of sufficient data, ability to resolve the identity of the target of the centroid, and availability of human raters for heuristic evaluation.
Potential limitations and remedies
This study’s method relies on defining a selected location’s physically surrounding commercial ecosystem. The current study accomplished this definition by constructing a geospatial centroid region and including all businesses incorporated in the region. This geospatial approach is best suited for facilities isolated or separated from population centers, as the studied bases were.
Moreover, this study’s method assumes data is available at a consistent level before, during, and after a disruption to the base. Interruptions to data collection could present falsely as disruptions to the base’s commercial ecosystem.
To address such potential limitations, this study could benefit by enriching the methodology with additional dyadic relationships. The nature of the method is such that it can be enriched over time by adding additional dyadic relationships without any change to the process. In frequent practice, complex relationships with more than ten dyadic relationship types are easily achievable with commercially available data. We fully expect that adding such data would increase the stability of the method and the granularity with which perturbation can be detected.
Additionally, the concept of a “disruption” could potentially be vague enough that given a facility such as a military base or corporate campus and sufficient time to investigate, a disrupting event or circumstance could be identified. The method employed in this study could catch some disruptions that were not experienced subjectively by individuals at the facility—while missing disruptions that were. However, even an incomplete record of disruptions could be valuable to, and exploitable by, an external actor intending to surveil and exploit the community.
For example, the data might indicate to moving companies the times of the year families are preparing for transfers in duty station or positions, which could also present an opportunity to blend in to a less routine and more chaotic environment.
Moreover, the research team recognized the statistical possibility that this study’s observed results conceivably could be attributed to variation in the graph metrics tied to seasonal patterns. If so, this would falsely indicate a disruption at the same time every year, for instance. That said, such a study interpretation risk did not appear to be an issue for the bases selected for the study.
Regarding changes in observable data and human behaviors
This study did consider changes in observable data. There are many potential sources of such change, including regulatory change (e.g., a base realignment), changes in commercial behavior (e.g., during supply chain disruption), introduction of complex collaboration structures in the ecosystem (e.g., exclusive trade agreements), corporate actions (mergers/acquisitions), and the introduction or departure of a significant entity in the commercial ecosystem (e.g., relocation, bankruptcy). Any such change could perturb the data itself, as well as disrupt the underlying ecosystem.
This study also considered changes in human behavior as it is important to consider such changes that take place over a longer duration of time. One example is the emergence of the gig economy, where individuals function as microcenters of economic activity within an ecosystem. Another example could be digitization, where companies deliver and support products and services in part or in whole by digital means (e.g., online customer service with automated programs). All such evolutions will produce additional dyads that have not been seen before, providing exciting potential to further study perturbation in the context of changing business behavior in the ecosystem.
Regarding open-source data implications
This study did consider open-source data and other commercially available data, to include LexisNexis media data sets, to determine if it is possible to identify disruptions in a facility using these lower fidelity sources. One feasible approach is to collect media documents mentioning a closed system and to chart the documents’ sentiment toward the closed system with respect to time. There are open-source and proprietary machine learning models that extract named entities, such as closed systems and their governing organizations, and estimate the document’s overall sentiment toward each entity. A perturbation in the sentiment toward a closed system could indicate a disruption internal to the closed system. This approach is constrained by the data available and the applicability of the sentiment analysis model to the documents analyzed.
Possible next steps based on this study
This study examined whether it is possible to detect perturbation at military bases or other important geographically localized institutions using commercial data sets. The limited research done in this study shows that by constructing polyhedrons for each month’s dyadic commercial relations, computing global graph metrics on each month’s polyhedron, and comparing subsequent months’ graph metrics using the Jensen-Shannon divergence, it is possible to detect perturbations around the military bases that we chose for the purpose of this study. To further evaluate the methodology demonstrated by this study, we recommend four additional steps:
Further this analysis to determine if any of the selected bases have clusters of key influential entities responsible for shaping how these communities react to disruptions.
- Conduct this analysis for ecosystems such as closed military communities or other geographically localized institutions to expose dispositive cues to the resiliency of the community-to-community leaders, local government, and Department of Defense representatives. Exposing relevant communities to this level of scrutiny from commercial data will allow the base to further secure itself.
- Establish a collaborative network of private-sector companies that are willing to share their data for fusing for data visualization to both conduct this type of analysis and collaborate on related projects to ensure resiliency and further national security. For such collaboration to occur, there should be an appropriate level of incentive for these companies to engage in data sharing.
- Continue to share this research with local government representatives and on-the-ground base representatives, and repeat a similar analysis on a semiregular basis to evaluate if and how the resiliency of the ecosystem has shifted since the first study. Based on the results of these studies, community leaders, local government representatives, and base officials should collaborate to formulate solutions to increase the long-term security of the military installations or other geographically localized institutions that are central to their communities.
Summary and closing implications
This report described analyses exploring what perturbations in commercial data patterns could reveal about potential disruptions in far more closed systems of activities and coordination, such as military bases or other closed ecosystems of high value and importance. The analyses detected perturbations around military bases in time and through time.
It then discussed the possible shortcomings and potential extensions of the analyses, to include employing, expanding, and refining the methods presented to analyze other closed systems of activities and coordination around the world. It follows that any attempts to camouflage or hide such perturbations will themselves leave entropic aberrations that analytic means can detect. This logic is akin to studies in thermodynamics, where any attempt to restore an ordered state to a system, a low state of energy (and thus high disorder) will create high disorder somewhere else.
The implications of this study seem to be that certain closed ecosystems cannot fully hide their internal states from the world, as the surrounding commercial ecosystem will reveal perturbations. This conclusion means activities that for whatever reason seek to keep a low-profile either in the United States or elsewhere could be producing digital exhaust associated with adjacent activities within the broader ecosystem in which they operate. This observation has substantial implications for intelligence and counterintelligence, military defense, and law enforcement activities. At the same time, nature’s disruptions also can produce indicators and warnings—be they of pandemic, food scarcity, or climate-related shocks and their associated perturbations on data from human societies.
In sum, there are five major takeaways from this report for leaders in both the public and private sectors:
- The increasing digitization of the planet enables subtle tips and cues to be derived from commercial data associated with entities and regions of interest.7“‘Instrumenting the Planet’: Dr. David Bray Discusses Internet of Things with AIPCA,” Executive Roundtable 2020 (video), GeoTech Cues (series), Atlantic Council, January 2020, https://www.atlanticcouncil.org/insight-impact/in-the-news/instrumenting-the-planet-dr-david-bray-discusses-internet-of-things- with-aipca/.
- Those seeking to keep their activities confidential need to be aware of the “digital exhaust” they may be producing associated with, adjacent activities within the broader ecosystem in which they operate.
- It is anticipated that any attempts to camouflage or hide such perturbations will themselves leave irregularities that similar analytic means can detect.
- Those seeking to better understand the planet, perhaps to prevent future outbreaks or be forewarned of a possible food crisis or the risk of growing instability in a region, should consider how commercial data sets can provide indicators and warnings associated with such phenomena.8David Bray, “We Can Build an Immune System for the Planet,” GeoTech Cues, Atlantic Council, April 6, 2020, https://www.atlanticcouncil.org/blogs/geotech-cues/we-can-build-an-immune-system-for-the-planet/.
- As organizations and societies become increasingly digitized, people will be able to better understand the impact of human activities on the world, as well as nature’s disruptions of human societies, better than ever before.
The study authors recommend interested organizations perform an extended analysis to “red team” the worst case scenarios, in terms of surveillance and threat exposure for installations of interest. Such an extended analysis should use the results of worst-case scenarios to build out and test potential improvements and mitigations to further secure military bases and other closed ecosystems of high value and importance.
About the authors
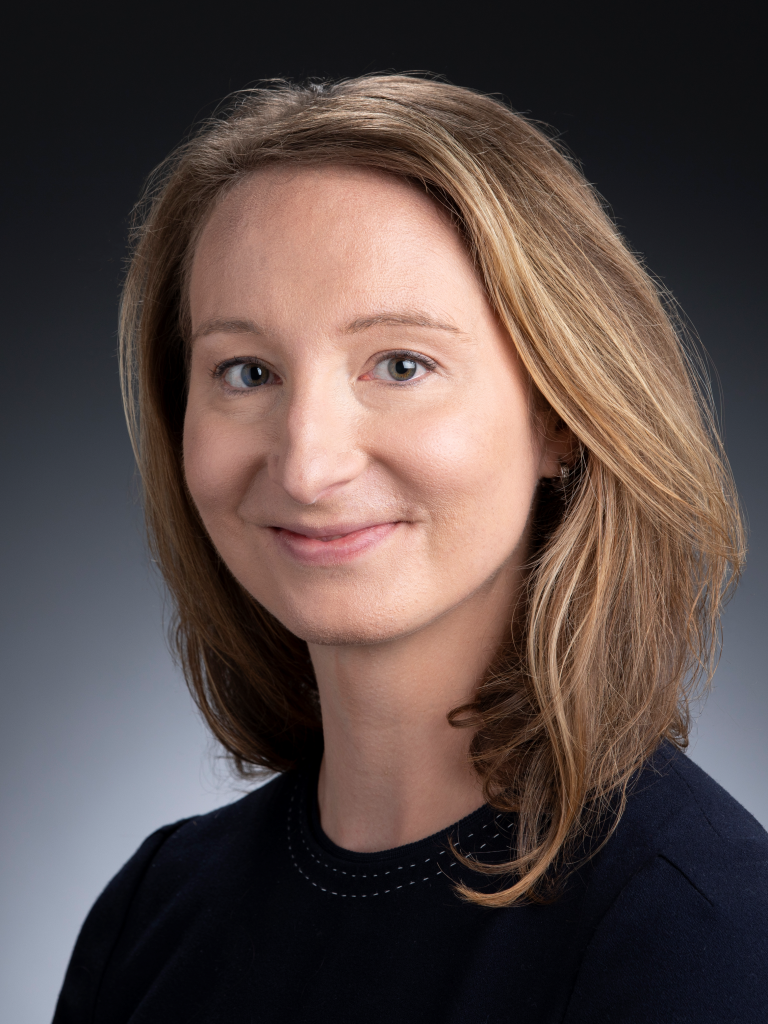
Natalie Barrett is a leader at the Johns Hopkins University Applied Physics Laboratory (JHU/APL), primarily focused on human-machine teams, artificial intelligence (AI), and advanced communications. She also serves on the laboratory’s climate change strategy team. Prior to joining JHU/ APL, she served across government and commercial industries focused on defense, innovation, robotics, and biotechnology. Key projects include wearables, exoskeletons, isothermal assays (realized in the rapid COVID-19 diagnostic tests), microarray reader systems for the Human Genome Project, and cancer research.
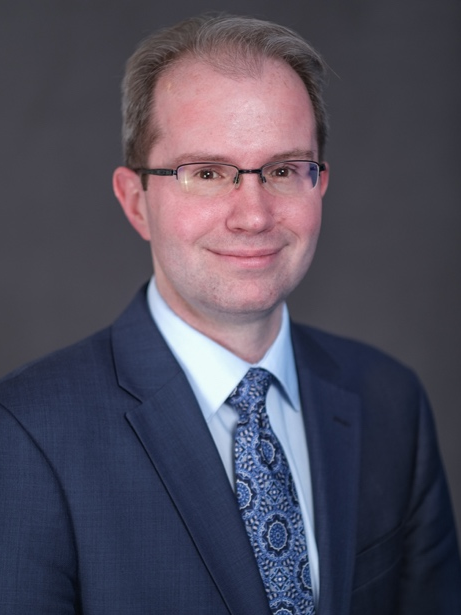
David A. Bray, PhD, is a distinguished fellow with the Atlantic Council. He is the founding principal at LeadDoAdapt Ventures and has served in a variety of leadership roles in turbulent environments, including bioterrorism preparedness and response with the Centers for Disease Control and Prevention and the broader US government from 2000 to 2005; executive director for a bipartisan US intelligence community commission on R&D; nonpartisan leadership as a federal agency senior executive; work with the US Navy and Marines on improving organizational adaptability; and efforts with US Special Operations Command on the challenges of countering disinformation online. He has received the Joint Civilian Service Commendation Award, Roger W. Jones Award for exceptional federal executive leadership, and the National Intelligence Exceptional Achievement Medal.
He also provides strategy to both boards and start-ups espousing human-centric principles to technology-enabled decision making in complex environments. He was named a senior fellow with the Institute for Human-Machine Cognition, starting in 2018. Business Insider named him one of the top “24 Americans Who Are Changing the World” under 40, and he was named a Young Global Leader by the World Economic Forum. He has served in roles such as president, chief strategy officer, and strategic adviser for twelve different start-ups. He has been an invited keynote speaker before audiences of CEOs and world leaders and at events with more than three thousand participants in India, Vietnam, Australia, Taiwan, Dubai, South Africa, Brazil, Colombia, Mexico, Canada, Belgium, Sweden, Switzerland, and the United Kingdom.
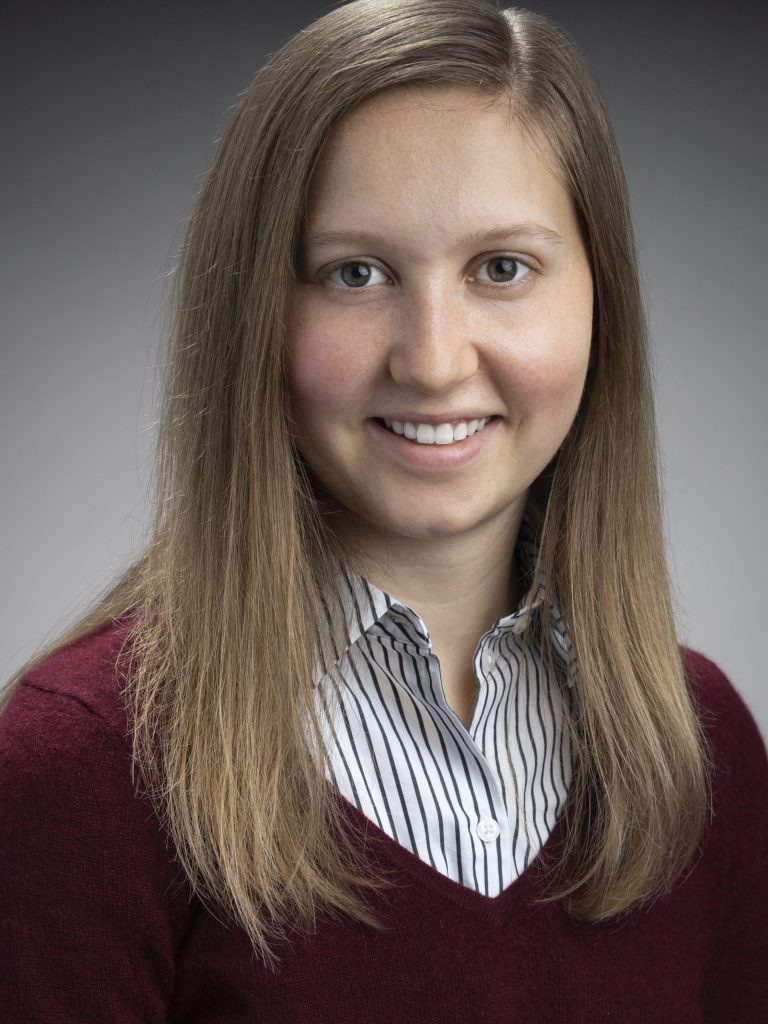
Mary Versa Clemens-Sewall is a data scientist at JHU/APL. She designs and implements data analysis methods, especially text analysis methods, for a range of applications. Prior to joining APL, she studied mathematics and Arabic at Dartmouth College. As of fall 2022, she is a PhD student in applied mathematics and statistics at Johns Hopkins University.
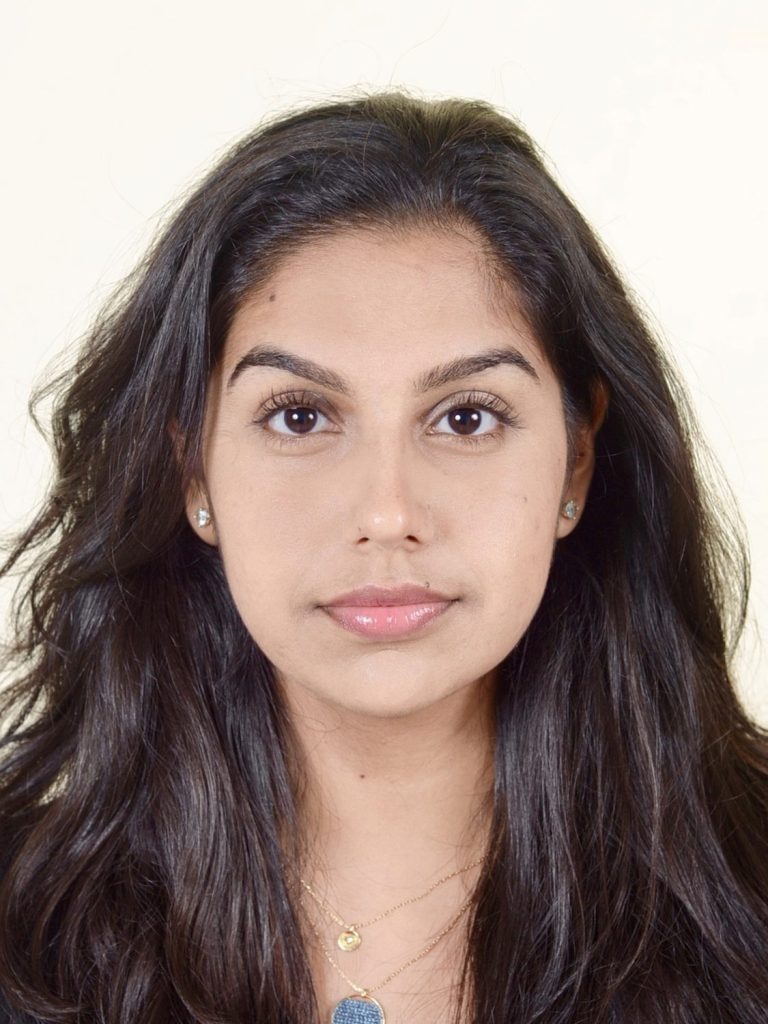
Kiran S. Jivnani is a program assistant at the Atlantic Council’s GeoTech Center. She manages projects at the intersection of geopolitics, security, climate, health, and agriculture. Prior to joining the Atlantic Council, she worked for the United Nations Academic Impact and Millennium Campus Network Millennium Fellowship, where she managed student leaders globally through mentorship on United Nations Sustainable Development Goal-based projects. She later worked for a former member of European Parliament and the Social Democrat Party vice president, Dr. Miriam Dalli. In this role she worked on legislative dossiers of the European Parliament’s Environment, Public Health, and Food Safety Committee; Industry, Research, and Energy Committee; and the Beating Cancer Committee. She holds a bachelor’s degree from Northeastern University in Boston, where she studied criminal justice, international affairs, and law and public policy.
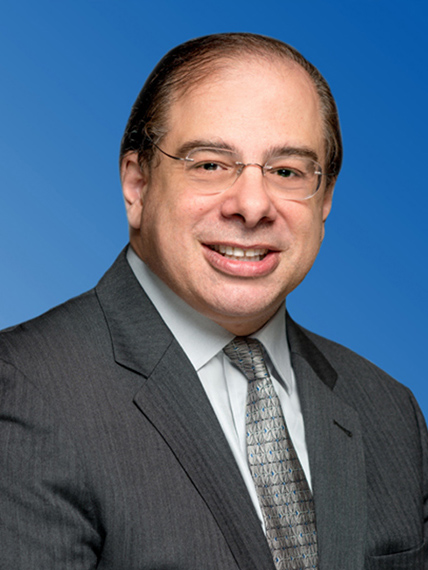
Anthony Scriffignano is Senior Vice President and Chief Data Scientist at Dun & Bradstreet Corp. He is an internationally recognized data scientist with experience spanning over forty years in multiple industries and enterprise domains. Scriffignano has an extensive background in advanced anomaly detection, computational linguistics, and advanced inferential algorithms, leveraging that background as primary inventor on multiple patents worldwide. Scriffignano was recognized as the US Chief Data Officer of the Year 2018 by the CDO Club, the world’s largest community of C-suite digital and data leaders. He also is a member of the Organisation for Economic Co-operation and Development’s Network of Experts on AI, a working group on implementing trustworthy AI. He has briefed the US National Security Telecommunications Advisory Committee and contributed to three separate reports to the president, on big data analytics, emerging technologies strategic vision, and Internet and communications resilience. Additionally, Scriffignano provided expert advice on private-sector data officers to a group of state CDOs and the White House Office of Science and Technology Policy.
Acknowledgments
The study authors would like to acknowledge the assistance of experts at Dun & Bradstreet, Johns Hopkins University Applied Physics Laboratory, and the Atlantic Council for their assistance in performing this study and preparing this report.
The authors would also like to specifically acknowledge the following persons for their editorial or analytic expertise and contributions to this report.
Editorial acknowledgments
Lloyd Whitman, PhD, Senior Director, GeoTech Center, Atlantic Council
Stephanie Wander, Director of Programs, GeoTech Center, Atlantic Council
Emily Sespico, Program Assistant, GeoTech Center, Atlantic Council
Analytic acknowledgments
Kamel Belkacem Boussaid, Senior Principal Data Scientist, Dun & Bradstreet
Ilya Meyzin, Vice President, Data Science Innovation and Operations, Dun & Bradstreet
David Spingarn, Leader, Identity Resolution (Global Matching), Dun & Bradstreet
Emma Rafkin, Software Engineer, John Hopkins Applied Physics Laboratory, John Hopkins University
Cetin Savkli, PhD, Chief Scientist, Johns Hopkins University, Applied Physics Laboratory
Image: Photo by Pawel Czerwinski on Unsplash