Data strategies for an AI-powered government
The public sector’s increasing demand for tools that can apply artificial intelligence (AI) to government data poses significant challenges for federal chief information officers (CIOs), chief data officers (CDOs), and other information technology (IT) stakeholders in the data ecosystem. The technical applications of AI built on federal data are extensive, including hyper-personalization of information and service delivery, predictive analytics, autonomous systems, pattern and anomaly detection, and more.
This community must simultaneously manage growing data lakes (on premises and cloud-based), ensure they follow best practices in governing and stewarding their data, and address demand from both within and outside government for equitable and secure access to data, while maintaining strong privacy protections.
These demands require each data owner to have a data infrastructure appropriate for AI applications. However, many federal IT systems do not yet have that infrastructure to support such applications—or a strategy to establish one—and many stakeholders may not yet recognize what data infrastructure and resources are required or whom to ask for help developing strategies and plans to make AI and machine-learning (ML) applications possible. Moreover, the resources needed are regularly not controlled by the CIO/CDOs or are often undervalued and overlooked by those who set budgets. Finally, not all agencies have the workforce with the skills necessary to build, maintain, and apply an AI/ML-ready data mesh and data fabric.
In two private webinars, the GeoTech Center explored:
- Maximizing the value of data through AI and how that capacity can be expanded.
- The importance of infrastructure, resources, and workforce skills needed to create an AI/ML-ready data mesh and data fabric.
- The challenges that agencies face to create these data infrastructures along with effective strategies, tactics, approaches, best practices, and lessons learned.
Key findings, to date, can be structured into four categories:
- Establishing human capital and an “AI-ready” culture
- Planning and developing data-centric AI applications
- Piloting data-centric AI applications
- Procuring and/or scaling data-centric AI applications
1. Establishing human capital and an “AI-ready” culture
Human capital and workforce challenges are foundational: it is critically important to integrate humans into the AI and data management process across the ecosystem and application lifecycles and obtain leadership buy-in on strategic approaches to leveraging data that balance other concerns such as security. Solutions include creating cross-functional task forces and working groups, embedding technology with operational users for immediate feedback, and rewarding (limited) risk-taking on AI projects.
There is a broad need to improve AI literacy across the enterprise, especially at the leadership level, to have meaningful conversations on how to move forward. With ML being at the forefront there is a tendency, especially out in the field, to confuse ML as the only form of AI that exists currently. To improve AI literacy, agencies need to focus on human and organizational behavior; for example, incentivizing actual uptake of a training course and making it part of everyone’s job description to learn about AI. It is also important to develop more acceptance of risk related to AI applications; users are not inherently accepting of automated systems with the potential to take on large significant aspects of their work. But they will find value in tools that augment their capabilities but do not take over their decision making.
For organizations that have not routinely leveraged data for analysis or policy insights (with or without AI), identifying and socializing mission-specific needs and insights that can be addressed helps establish an initial stakeholder community—for example, priority and/or long-standing personnel, financial, operational, or policy questions where existing or new data and AI might reveal actionable insights.
Agencies should consider:
- Creating cross-functional task forces and working groups around getting data AI-ready–the solution is at least as much about organizational adaptation as it is about technological change. Such groups can also be tasked with identifying key questions where data and AI might reveal actionable insights.
- Rewarding (limited) risk-taking on AI projects, balancing ‘misuse versus missed use’ and encouraging an approach of ‘yes, unless’ for data sharing.
- Examining roadblocks within the organization to move the use-case forward and ensure the organization has an adequate workforce needed given the scale of each problem.
- Sending clear demand signals and, explaining the value proposition and scalability of data-centric AI applications, making clear the return on investment and measures of effectiveness.
- Working with service providers to understand how they use AI and how to use AI through their services.
2. Planning and developing data-centric AI applications
Federal agencies maintain and/or have access to an overwhelming quantity of data—structured and unstructured, qualitative and quantitative, inputs and outputs—that create unique data governance challenges. Data is often poorly structured and not organized in a way amenable to equity assessments or application/use by AI tools. Therefore, it is important to consider up front the data management pipeline, including how to efficiently obtain, clean, organize, and deploy data sets; i.e., getting the data “right” before using it in an AI application. Similarly, when possible, proactively consider what applications might arise from a data set before collection, which will improve the subsequent usability of that data and reduce ‘application drift’ (changes in use and scope beyond the original intention).
The pipeline includes not just the technical aspects of data management but also the need to treat data management as a business problem. Moreover, data is often siloed and generally inaccessible to those outside of the organization in which it was created, preventing its use in machine learning applications outside of this closed ecosystem. Data may also be separated between networks, locations, and classifications. These silos hamper the efficient use of information.
AI relies on data, but senior leaders tend to look at AI as a capability rather than a technology that can create a capability when applied to the right data and/or problem—if agencies don’t have an application in mind, they need to start thinking about getting their data AI-ready—including thinking about getting their infrastructure ready. Digital modernization across the US government is an ongoing challenge, so infrastructure is often not being built fast enough or is being outsourced to the private sector, creating additional challenges, including privacy and security.
It is important to consider the value of curated or specialized data and the tension between quantity and quality. The challenge lies in choosing between high-precision, function-specific applications and more generalized data that can be applied to a broader range of solutions.
The White House Office of Science and Technology Policy (OSTP) is working to help agencies turn data into action by collecting data purposefully in such a way that they can more easily parse it and achieve equitable outcomes. OSTP views equitable data as data that allows for the rigorous assessment of the extent to which government programs yield fair, just outcomes for all individuals.
Some agencies are finding value in AI-generated synthetic data, that can be higher quality and more representative than human-labeled data for selected ML applications while addressing concerns about protecting privacy associated with real data (even when anonymized). However, recursive use of synthetic data—i.e., using information generated from synthetic data in repeated cycles of training—should be avoided as it leads to spurious output.
In the health sector, a major challenge continues to be the need to convert images (such as faxes, which are still widely used) into structured data suitable for AI applications.
Agencies should consider:
- Operationalizing data repositories into a data fabric, allowing for organization-wide access to data resources.
- Establishing a dedicated point of contact within agencies for data repository requests.
- Ensuring that customers know where their data is and who owns it.
- Treating data as a product that requires trust and continually seek feedback on how the data is being made available and used.
- Balancing data push (collecting data for an application) vs. data pull (using data for an application) by evaluating what applications can be done with existing data rather than collecting new data.
- Proactively considering what applications might arise from new data before collecting that data.
- Working across the interagency to create common tags for fair data and shared test data sets.
- Integrating privacy principles from the start in projects, including through privacy impact assessments, using appropriate types of encryption everywhere it is required, along with appropriate access controls.
- Stratifying applications based on risk, which will enable a graded approach, where lower risk applications can be pursued with relatively fewer restrictions, and higher risk applications would require rigorous testbed deployments and sufficient human oversight.
3. Piloting data-centric AI applications
As for the planning stage, managing and maintaining the data pipeline is key, from getting the data, cleaning and organizing the data, to deploying the data. Treating data as a business problem is just as important as treating it as a hardware/infrastructure problem. Ontology is very important to get data right and must evolve as the uses of the data evolve. Once there is a common ontology, the data can be released to model trainers and industry partners. The order of the workflow is as follows: getting data ‘right’…then deploying models utilizing that data. However, it is difficult to get program managers to think strategically about data up front, resulting in myriad challenges down the road. “Think about data first!”
When it comes to more specialized or narrowly focused data sets, one must prioritize quality over quantity. There is a tension between solving a particular problem with high precision vs a general problem with many solutions. Quantity may be a quality all on its own that can be addressed separately. There may be pressure to “go big” or “not at all”.
During pilots it is important to integrate the application with human systems, getting it into the hands of users and continuously obtaining feedback, reexamining the data, and updating the software in real time.
Agencies should consider:
- Embedding the technology with operational users as quickly as possible for immediate feedback and to identify unanticipated problems through extensive testing, including infrastructure and data challenges. To maximize this feedback loop, organizations may need to rethink where humans and machines interact and be willing to expose the user (or at least early users) to some level of complexity that may in the end be hidden.
- Being flexible, agile, and forward leaning with people on the “forward tip of the spear” and embedding data professionals in projects who understand both the data and the mission.
- Picking key anchor projects that have high leverage potential. Find an anchor tenant and build it so to quickly start interacting with data, managing access control, and optimizing the data platforms.
- Identifying applications and pilots that could be expanded across sectors/organizations, for example, by adding additional data repositories into a data fabric, creating agency-wide models, and/or making data resources available across the enterprise.
4. Procuring and/or scaling data-centric AI applications
It is common in the US government to consider the commercial sector ahead of the government in adopting new technology, including AI. Although AI-enabled applications have matured enough to be readily adopted for US government applications, commercial providers require data of sufficient quality to engender trust in the insights or outputs from deployed applications. Partnerships with the private sector are needed to move the needle across the US government—the current attention and momentum in commercial and government sectors are data and AI are exciting.
A promising area to scale is leveraging large language models (LLMs) ability to write code and find bugs. ChatGPT and other LLMs can now be as effective as previous bespoke tools. (A common non-result for ChatGPT is a request for more information, which when available can lead to useful results.) These technologies will help produce tools to find and fix bugs quickly—even when only applied to “easier/shallower” bugs, this application would be a huge win.
A challenge to scaling LLMs/generative AI at this time is that hallucination rates can approach 30 percent—this rate needs to be brought down before widespread use. Although the capabilities of these systems will ultimately lead to valuable applications, getting hallucination rates down will be difficult. The promise is great, but we have not yet reached the full potential, technology-wise.
Generative AI also introduces new threats that must be acknowledged and rapidly addressed, especially for misinformation from sound/video/image production. Moreover, AI agents will be connected to the Internet—and therefore the physical world. In combination with reinforcement learning such agents could be capable of autonomously causing harm in the physical world.
Agencies should consider:
- Involving downstream users from the start of the transition process and making sure they know what’s coming and can provide feedback.
- Developing a culture and messaging strategy that makes clear that the agency is not deploying AI without considering broader applications and future scale, and strongly encourages partnerships while still maintaining focus on the priority projects.
- Identifying solutions in the start-up community that can be shaped for different applications along with emerging capabilities that could be useful soon (and get the US government ready to adopt).
- Moving to contract using industry best-of-breed design principles and flexible acquisition authorities when available.
- Building transparency, testing and evaluation, privacy safeguards and other elements of responsible AI into the planning and procurement process.
Acknowledgements
These findings and recommendations were produced by the Atlantic Council GeoTech Center following private discussions with IT, data science, and AI leaders and experts in both the public and private sectors. This effort has been made possible through the generous support of Accenture Federal Services and Amazon Web Services.
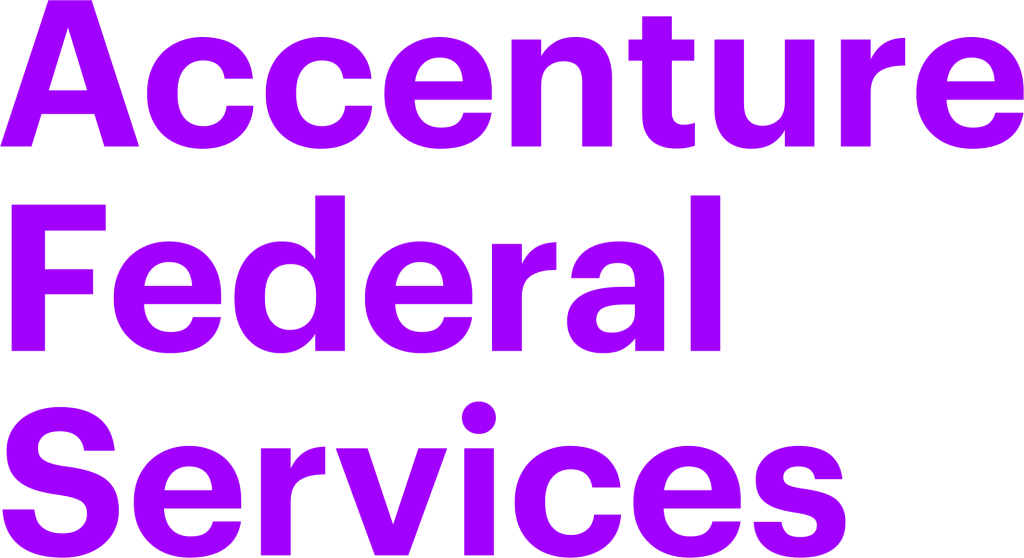
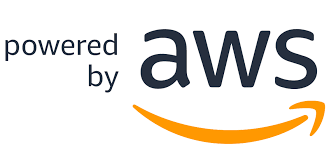
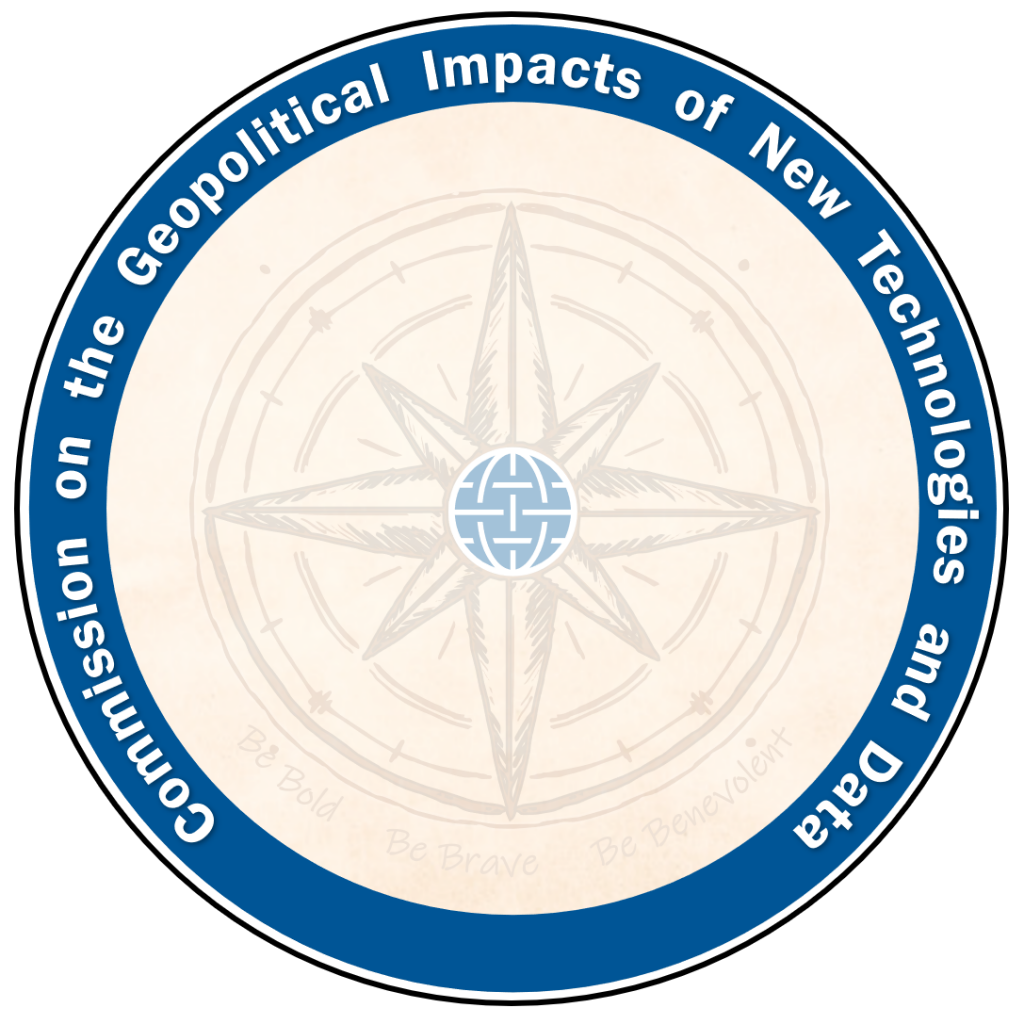
GeoTech Center
Championing positive paths forward that societies can pursue to ensure new technologies and data empower people, prosperity, and peace.