Busting the top myths about AI and energy efficiency
Josh Parker is the senior director of corporate sustainability at Nvidia. This essay is part of the Global Energy Agenda.
The rapid growth of AI in recent years has sparked an unprecedented rush of investment in data centers worldwide to develop the next generation of algorithms, fueling concerns that running these systems will push the world toward an energy crisis.
However, to determine the true impact of AI on global energy consumption, consider the full picture:
- AI computing still makes up a tiny slice of the world’s energy consumption. Data centers accounted for about 2 percent of energy-related carbon emissions in 2022, according to the International Energy Agency—and today, not all data centers run AI.
- AI, powered by rapidly advancing accelerated computing technology, is becoming much more energy efficient every year.
- AI delivers insights and results that can increase energy efficiency in the domains that use energy the most—including energy generation, manufacturing, transportation, and residential heating and cooling.
Recent advancements in AI and accelerated computing have enabled developers to harness more computational capabilities while using less energy. Some—in climate science, financial services, and healthcare—already are. But to achieve widespread adoption, it’s critical to separate misconceptions from reality.
To that end, here are the top myths around AI and energy efficiency, and the long-term perspectives and facts that dispel them.
MYTH: The carbon footprint and energy consumption of data centers will grow at the same rate as computation.
Growing demand for computing power does not result in an equivalent rise in energy consumption.
Global data centers saw a 550 percent increase in compute instances—which are virtual machines—and a 2,500 percent jump in storage capacity between 2010 and 2018, while electricity use rose only 6 percent, noted a report from the Information Technology and Innovation Foundation, a Washington-based think tank.
These initial energy savings were largely due to the effects of Moore’s Law, which predicted that the number of transistors on a chip would double approximately every two years, leading to a biannual doubling in computing power while maintaining similar energy consumption.
However, by the mid-2010s, Moore’s Law began to slow as the physical limits of shrinking transistors became more challenging to overcome. This slowdown highlighted the need for new approaches to maintain and accelerate efficiency gains. Accelerated computing emerged as the solution, leveraging specialized hardware like graphics processing units (GPUs) to perform tasks more efficiently than central processing units (CPUs).
Today, accelerated computing is transforming the world’s data centers, with GPUs and advanced networking technology replacing traditional CPU servers that struggle to keep pace with the rise in computing demand. The parallel computing capabilities of GPUs make them twenty times more energy-efficient than CPUs. If every data center shifted from CPU-based to GPU-based infrastructure, the world would save an estimated 40 terawatt-hours of energy, equivalent to the annual energy usage of five million US homes.
MYTH: The computing processes required to run AI systems are much more resource intensive than previous methods.
The demand for new AI models, and therefore compute demand, is growing exponentially. The result is that AI is currently demanding more energy faster than computing is getting more efficient.
But both the performance and energy efficiency of accelerated computing increase with each GPU generation: meaning that with every advancement, developers and scientists can accomplish more compute work with less energy. Today’s most advanced AI chip matches the performance of supercomputers that were among the fastest in the world a decade ago.
The newest GPUs deliver thirty times more compute performance with a twenty-five-fold increase in energy efficiency compared to those built just two years ago. This adds up to greater efficiency over several years by a factor of 45,000.
MYTH: AI is consuming more energy than it will save.
The rate of AI adoption today is resulting in short-term increases in energy usage, but one long-term view is optimistic.
Claims of an “AI doomsday” often rely on extrapolations from published AI training statistics. But training predictive and generative AI models isn’t a goal in itself—the real goal is to use those models. The insights that an AI model provides during inference can save time and energy and reduce carbon emissions in resource-intensive domains such as agriculture, weather forecasting, transportation, manufacturing, and drug discovery.
Accelerated computing and AI can also power climate models that help global organizations more effectively predict weather patterns, manage natural disasters, build climate-resilient infrastructure, and save lives.
It takes a holistic, longitudinal view to fully calculate the efficiencies that stem from AI adoption. While many AI initiatives are currently in the infrastructure building or training phases, with widespread implementation still to come, early adopters are already seeing benefits.
Efforts to increase energy efficiency and decarbonize buildings across industries are one critical use case for AI. In the United States, buildings are responsible for 40 percent of total energy usage—and, according to the Environmental Protection Agency, 30 percent of energy used in commercial buildings is wasted.
Peter Herweck, former CEO of Schneider Electric, has predicted that in the next few years AI could reduce energy consumption in buildings by up to 25 percent. Data collected by smart home devices and smart meters are producing data that could train AI models to find optimizations across residential and commercial buildings.
For example, a pharmaceutical company worked with BrainBox AI, which helps customers optimize their buildings with AI, to boost equipment efficiency at its California campus, making improvements that resulted in annualized electricity savings of 156,000 kilowatt-hours.
Healthcare is energy intensive: The industry’s facilities account for close to 10 percent of commercial building energy consumption in the United States and about 4.6 percent of global greenhouse gas emissions. The life-saving research processed within them is also computationally demanding.
Genome sequencing is one example. Sequencing the DNA of tumors and healthy tissues is crucial to understanding genetic drivers of cancer and identifying treatments. Using AI, the Wellcome Sanger Institute has significantly reduced the “runtime” (i.e., how long a program runs to execute its function) and energy consumption of genomic analysis—saving approximately 1,000 megawatt-hours annually and potentially reducing costs by $1 million compared to traditional CPU-based methods.
MYTH: Electric grids can’t handle the energy load of growing AI use.
AI models can be trained anywhere—and there’s a significant opportunity to build future data centers in parts of the world where there’s excess energy, such as near geothermal reservoirs, which act as 24/7 renewable energy sources, unaffected by weather conditions.
Rather than placing every data center in urban areas that already have significant power demands, they could be built near these sources of renewable energy. Doing so minimizes transmission issues while simultaneously decreasing or eliminating operational carbon footprints.
Once they’re trained, models can be deployed to GPUs, which are twenty times more efficient for AI inference tasks than CPUs. Beyond large data centers, lightweight models optimized for inference can run anywhere—on small embedded systems on a robot or other edge device, on desktop workstations, or on cloud servers located in any part of the world.
AI is becoming an essential technology for businesses in nearly every industry to improve productivity and enable rapid new advancements and discoveries. And although AI’s direct energy footprint is certainly growing, AI is also proving to be a powerful tool for finding ways to save energy and may very well become the best tool we have for advancing sustainability worldwide.
All essays
Explore the program
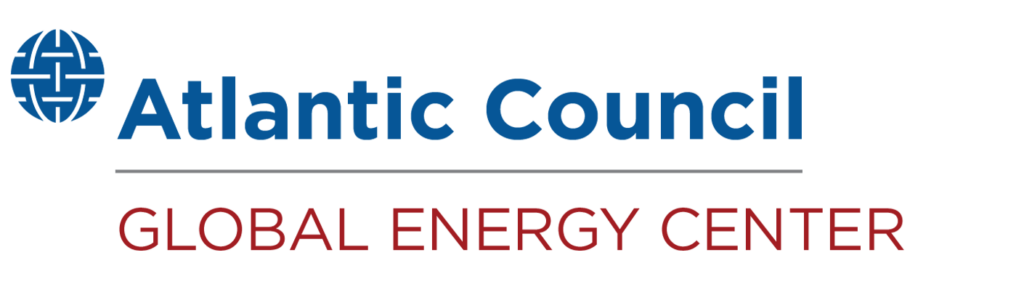
The Global Energy Center develops and promotes pragmatic and nonpartisan policy solutions designed to advance global energy security, enhance economic opportunity, and accelerate pathways to net-zero emissions.
Image: Data centers filled with rows and rows of servers as blinking light indicate constant processing, high detail, cinematic angle composition