Artificial intelligence (AI) is causing significant structural changes to global competition and economic growth. AI may generate trillions of dollars in new value over the next decade, but this value will not be easily captured or evenly distributed across nations. Much of it will depend on how governments invest in the underlying computational infrastructure that makes AI possible.
Yet early signs point to a blind spot—a lack of understanding, measurement, and planning. It comes in the form of “compute divides” that throttle innovation across academia, startups, and industry. Policymakers must make AI compute a core component of their strategic planning in order to fully realize the anticipated economic windfall.
What is AI compute?
AI compute refers to a specialized stack of hardware and software optimized for AI applications or workloads. This “computer” can be located and accessed in different ways (public clouds, private data centers, individual workstations, etc.) and leveraged to solve complex problems across domains from astrophysics to e-commerce and to autonomous vehicles.
Conventional information technology (IT) infrastructure is now widely available as a utility through public cloud service providers. This idea of “infrastructure as a service” includes computing for AI, so the public cloud is duly credited with democratizing access. At the same time, it has created a state of complacency that AI compute will be there when we need it. AI, however, is not the same as IT.
Today, when nations plan for AI, they gloss over AI compute, with policies focused almost exclusively on data and algorithms. No government leader can answer three fundamental questions: How much domestic AI compute capacity do we have? How does this compare to other nations? And do we have enough capacity to support our national AI ambitions? This lack of uniform data, definitions, and benchmarks leaves government leaders (and their scientific advisors) unequipped to formulate a comprehensive plan for AI compute investments.
Recognizing this blind spot, the Organisation for Economic Cooperation and Development (OECD) recently established a new task force to tackle the issue. “There’s nothing that helps our member countries assess what [AI compute] they need and what they have, and so some of them are making large but not necessarily well-informed investments” in AI compute, Karine Perset, head of the OECD AI Policy Observatory, told VentureBeat in January.
Measuring domestic AI compute capacity is a complex task compounded by a paucity of good data. While there are widely accepted standards for measuring the performance of individual AI systems, they use highly technical metrics and don’t apply well to nations as a whole. There is also the nagging question of capacity versus effective use. What if a nation acquires sufficient AI compute capacity but lacks the skills and ecosystem to effectively use it? In this case, more public investment may not lead to more public benefit.
Nonetheless, more than sixty national AI strategies were formulated and published from June 2017 to December 2020. These plans average sixty-five pages and coalesce around a common set of topics including transforming legacy industries, expanding opportunities for AI education, advancing public-sector AI adoption, and promoting responsible or trustworthy AI principles. Many national plans are aspirational and not detailed enough to be operational.
In conducting a survey of forty national AI plans, and analyzing the scope and depth of content around AI compute, we found that on average national AI plans had 23,202 words while sections on AI compute averaged only 269 words. Put simply, 98.8 percent of the content of national AI strategies focuses on vision, and only 1.2 percent covers the infrastructure needed to execute this vision.
Engine for economic growth
This would be the equivalent of a national transportation strategy that devotes less than 2 percent of its recommendations to roads, bridges, and highways. Just like transportation investments, AI compute capacity will be a crucial driver of the future wealth of nations. And yet, national AI plans generally exclude detailed recommendations on this topic. There are exceptions, of course. France, Norway, India, and Singapore had particularly comprehensive sections on AI infrastructure, some with detailed recommendations for AI compute requirements, performance, and system size.
Exponential growth in computational power has led to astonishing improvements in AI capabilities, but it also raises questions of inequality in compute distribution. Fairness, inclusion, and ethics are now center stage in AI policy discussions—and these apply to compute, even though it is often ignored or relegated to side workshops at major conferences. Unequal access to computational power as well as the environmental cost of training computationally complex models require greater attention.
Some of the more popular AI models are large neural networks that run on state-of-the-art machines. For example, AlphaGo Zero and GPT-3 require millions of dollars in AI compute. This has led to AI research being dominated and shaped by a few actors mostly affiliated with big tech companies or elite universities. Governments may have to step up and reduce the compute divide by developing “national research clouds.” Initiatives in Europe and China are underway to develop indigenous high-performance computing (HPC) technologies and related computing supply chains that reduce their dependence on foreign sources and promote technology sovereignty.
Governments in the United States, Europe, China, and Japan have been making substantial investments in the “exascale race,” a contest to develop supercomputers capable of one billion billion (1018) calculations per second. The rationale behind investments in HPC is that the benefits are now going beyond scientific publications and prestige, as computing capabilities become a necessary instrument for scientific discovery and innovation. The machines are the engine for economic prosperity.
What can policymakers do?
Understanding compute requirements is a non-trivial measurement challenge. At a micro level, it is important to scientifically understand the relative contribution of AI compute to driving AI progress on, for example, natural-language understanding, computer vision, and drug discovery. At a macro level, nations and companies need to assess compute requirements using a data-driven approach that accounts for future industrial pathways. Wouldn’t it be eye-opening to benchmark how much compute power is being used at the company or national level and to help evaluate future compute needs?
In 2019, Stanford University organized a workshop with over one hundred interdisciplinary experts to better understand opportunities and challenges related to measurement in AI policy. The participants unanimously agreed that “growth in computational power is leading to measurable improvements in AI capabilities, while also raising issues of the efficiency of AI models and the inherent inequality of compute distribution.” Getting measurement right will be a stepping stone to addressing this blind spot in national AI policymaking. Going forward, we recommend that governments pivot to develop a national AI compute plan with detailed sections on capacity, effectiveness, and resilience.
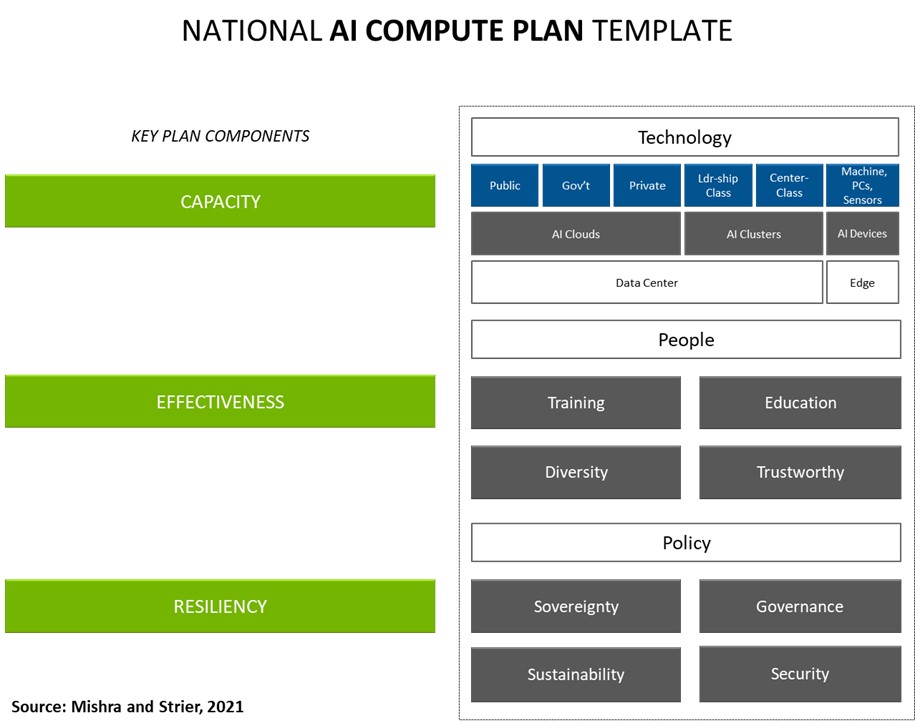
- Capacity for AI compute exists along a continuum. This includes public cloud, sovereign cloud (government-owned or controlled), private cloud, enterprise data centers, AI labs/Centers of Excellence, workstations, and “edge clusters” (small AI supercomputers situated outside a data center). Understanding the current state of AI compute capacity across this continuum will inform public priorities and investments. Partnerships with public cloud service providers expand access to AI compute and should be viewed as complementary (not in competition) with investments in domestic AI compute capacity. This hybrid approach will help close compute divides. Over the longer term, capacity planning should consider the shift from data center to edge computing. For example, self-driving trucks will be powered by on-board AI supercomputers, and it may be possible to run AI workloads on fleets of self-driving trucks, leveraging them as a “virtual” AI supercomputer when they are parked and garaged overnight.
- Effectiveness is achieved through a multitude of initiatives, from STEM education to open data policies. Examples include National Youth AI Challenges, industry or government hackathons, mid-career re-training programs, boot camps, and professional certification courses. Diversity across all these programs is key to promoting inclusiveness and creating a foundation for trustworthy AI.
- Resilience means nations should have an AI compute continuity plan, and possibly a strategic reserve, to ensure that mission-critical public AI research and governance functions can persist. It’s also important to focus on the carbon impact of national AI infrastructure, given that large-scale computing exacts an increasingly heavy toll on the environment and hence the broader economy. An optimal strategy would be a hybrid, multi-cloud model that blends public, private, and government-owned or controlled (sovereign) infrastructure, with accountability to encourage “Green AI.”
The completeness of a national AI strategy forecasts that nation’s ability to compete in the digital global economy. Few national AI strategies, however, reflect a robust understanding of domestic AI compute capacity, how to use it effectively, and how to structure it in a resilient manner. Nations need to take action by measuring and planning for the computational infrastructure needed to advance their AI ambitions. The future of their economies is at stake.
Saurabh Mishra is an economist and former researcher at Stanford University’s Institute for Human-Centered Artificial Intelligence.
Keith Strier is the chair of the AI Compute Taskforce at the Organization for Economic Cooperation and Development, and vice president for Worldwide AI Initiatives at the NVIDIA corporation.
Further reading
Image: Servers run inside the Facebook New Albany Data Center on Thursday, February 6, 2020 in New Albany, Ohio. Photo via Joshua A. Bickel/Dispatch and Reuters.